A Hierarchical Bayesian Model For Pattern Recognition
2012 INTERNATIONAL JOINT CONFERENCE ON NEURAL NETWORKS (IJCNN)(2012)
摘要
The success of automated classification hinges on the choice of the representation of the data. Much research has focused on feature extraction techniques that can identify highly informative representations of a dataset. In this paper, we adapt for the purposes of classification a hierarchical Bayesian model developed by Karklin and Lewicki to model the neurophysiological properties of the cortex. The hierarchical nature of the cortex enables it to capture successively abstract and nonlinear features within its stimulus. We show empirically that the properties of natural images that motivated this model are also present in non-homogenous data typical of classification tasks. We also propose a discriminative training method for the model that enables it to preferentially select features that best distinguish the output class labels. Finally, the performance of the model was tested on handwritten digit recognition and face recognition. We found that classification using features extracted from the model achieved greater performance than classification using the nonlinear features of Kernel Fisher Discriminant analysis alone.
更多查看译文
关键词
integrated circuits,image classification,pattern recognition,data models,feature extraction,face recognition,statistical analysis,kernel,kernel fisher discriminant analysis
AI 理解论文
溯源树
样例
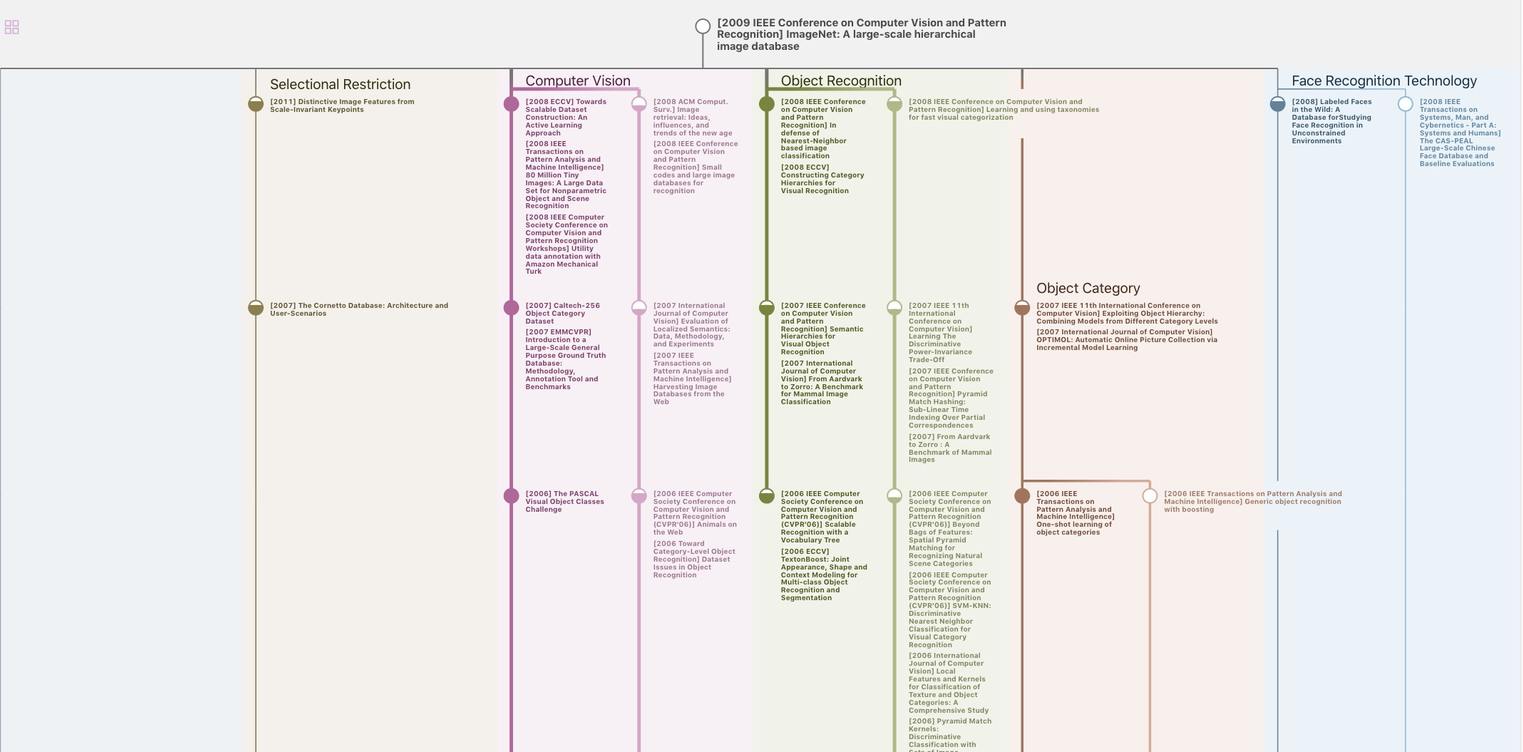
生成溯源树,研究论文发展脉络
Chat Paper
正在生成论文摘要