Applying optimal algorithm to data-dependent kernel for hyperspectral image classification
IGARSS(2010)
摘要
In the kernel methods, it is very important to choose a proper kernel function to avoid overlapping data. Based this fact, in this paper we mainly utilize a unified kernel optimization framework on the hyperspectral image classification to augment the margin between different classes, and under the kernel optimization framework, to employ the Fisher discriminant criteria formulated in a pairwise manner as the objective functions to optimize the kernel function in Kernel-based nonparametric weighted feature extraction. The experimental results display the superiority of the optimizing kernel function over the RBF kernel function with 5-fold cross-validation method, especially, in the small sample size problem.
更多查看译文
关键词
feature space,hyperspectral image classification,kernel methods,data-dependent kernel,operating system kernels,kernel optimization,support vector machine,pairwise manner,fisher criteria,feature extraction,image classification,kernel-based nonparametric weighted feature extraction,fisher discriminant criteria,cross validation,support vector machines,objective function,kernel function,kernel,optimization,kernel method,classification algorithms,hyperspectral imaging
AI 理解论文
溯源树
样例
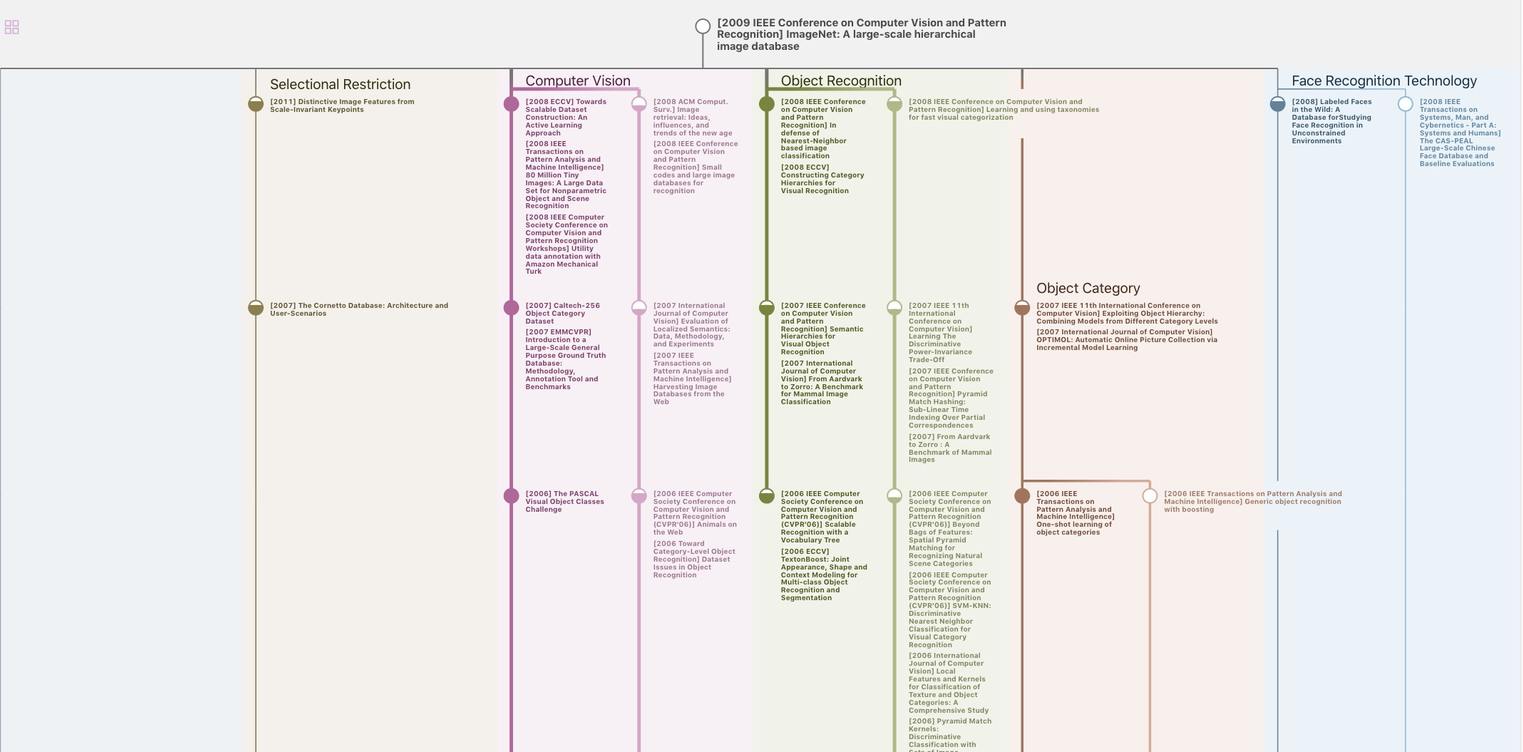
生成溯源树,研究论文发展脉络
Chat Paper
正在生成论文摘要