Semi-nonnegative matrix factorization with global statistical consistency for collaborative filtering.
Proceedings of the 18th ACM conference on Information and knowledge management(2009)
摘要
Collaborative Filtering, considered by many researchers as the most important technique for information filtering, has been extensively studied by both academic and industrial communities. One of the most popular approaches to collaborative filtering recommendation algorithms is based on low-dimensional factor models. The assumption behind such models is that a user's preferences can be modeled by linearly combining item factor vectors using user-specific coefficients. In this paper, aiming at several aspects ignored by previous work, we propose a semi-nonnegative matrix factorization method with global statistical consistency. The major contribution of our work is twofold: (1) We endow a new understanding on the generation or latent compositions of the user-item rating matrix. Under the new interpretation, our work can be formulated as the semi-nonnegative matrix factorization problem. (2) Moreover, we propose a novel method of imposing the consistency between the statistics given by the predicted values and the statistics given by the data. We further develop an optimization algorithm to determine the model complexity automatically. The complexity of our method is linear with the number of the observed ratings, hence it is scalable to very large datasets. Finally, comparing with other state-of-the-art methods, the experimental analysis on the EachMovie dataset illustrates the effectiveness of our approach.
更多查看译文
AI 理解论文
溯源树
样例
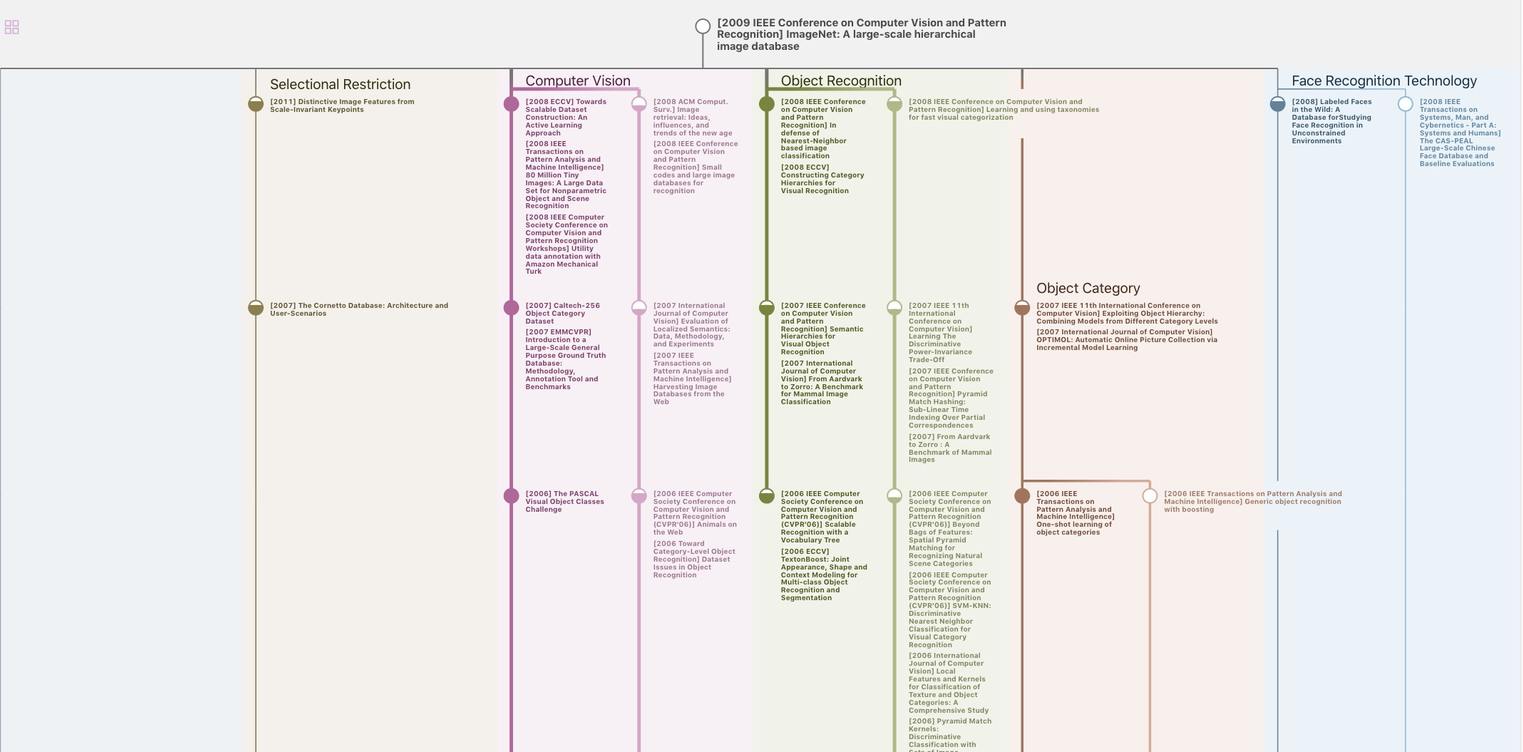
生成溯源树,研究论文发展脉络
Chat Paper
正在生成论文摘要