A Study Of Adaboost With Naive Bayesian Classifiers: Weakness And Improvement
COMPUTATIONAL INTELLIGENCE(2003)
摘要
This article investigates boosting naive Bayesian classification. It first shows that boosting does not improve the accuracy of the naive Bayesian classifier as much as we expected in a set of natural domains. By analyzing the reason for boosting's weakness, we propose to introduce tree structures into naive Bayesian classification to improve the performance of boosting when working with naive Bayesian classification. The experimental results show that although introducing tree structures into naive Bayesian classification increases the average error of the naive Bayesian classification for individual models, boosting naive Bayesian classifiers with tree structures can achieve significantly lower average error than both the naive Bayesian classifier and boosting the naive Bayesian classifier, providing a method of successfully applying the boosting technique to naive Bayesian classification. A bias and variance analysis confirms our expectation that the naive Bayesian classifier is a stable classifier with low variance and high bias. We show that the boosted naive Bayesian classifier has a strong bias on a linear form, exactly the same as its base learner. Introducing tree structures reduces the bias and increases the variance, and this allows boosting to gain advantage.
更多查看译文
关键词
bias and variance analysis, boosting, naive Bayesian classification
AI 理解论文
溯源树
样例
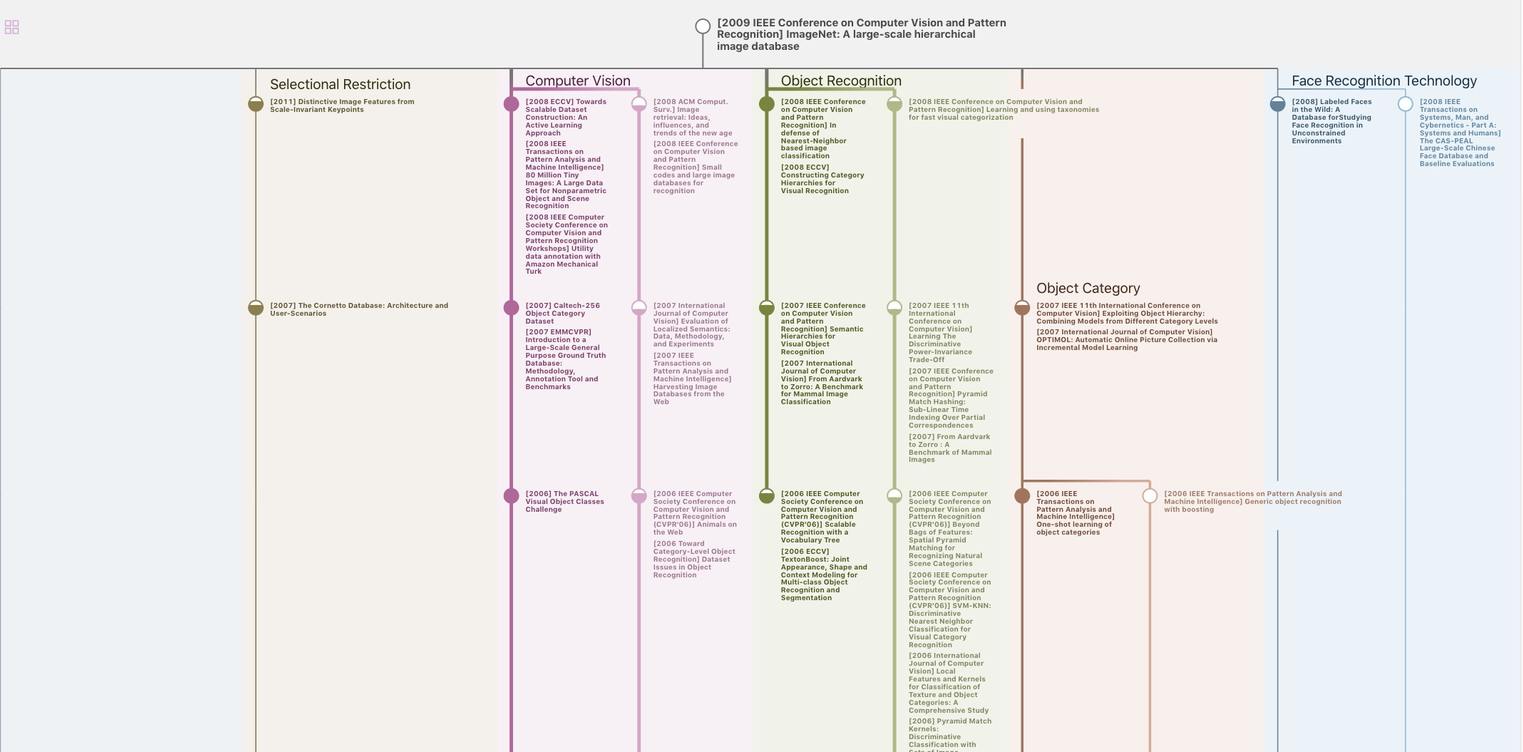
生成溯源树,研究论文发展脉络
Chat Paper
正在生成论文摘要