A biterm topic model for short texts
WWW(2013)
摘要
Uncovering the topics within short texts, such as tweets and instant messages, has become an important task for many content analysis applications. However, directly applying conventional topic models (e.g. LDA and PLSA) on such short texts may not work well. The fundamental reason lies in that conventional topic models implicitly capture the document-level word co-occurrence patterns to reveal topics, and thus suffer from the severe data sparsity in short documents. In this paper, we propose a novel way for modeling topics in short texts, referred as biterm topic model (BTM). Specifically, in BTM we learn the topics by directly modeling the generation of word co-occurrence patterns (i.e. biterms) in the whole corpus. The major advantages of BTM are that 1) BTM explicitly models the word co-occurrence patterns to enhance the topic learning; and 2) BTM uses the aggregated patterns in the whole corpus for learning topics to solve the problem of sparse word co-occurrence patterns at document-level. We carry out extensive experiments on real-world short text collections. The results demonstrate that our approach can discover more prominent and coherent topics, and significantly outperform baseline methods on several evaluation metrics. Furthermore, we find that BTM can outperform LDA even on normal texts, showing the potential generality and wider usage of the new topic model.
更多查看译文
关键词
coherent topic,conventional topic model,new topic model,real-world short text collection,short document,topic learning,word co-occurrence pattern,whole corpus,short text,biterm topic model,content analysis,topic model
AI 理解论文
溯源树
样例
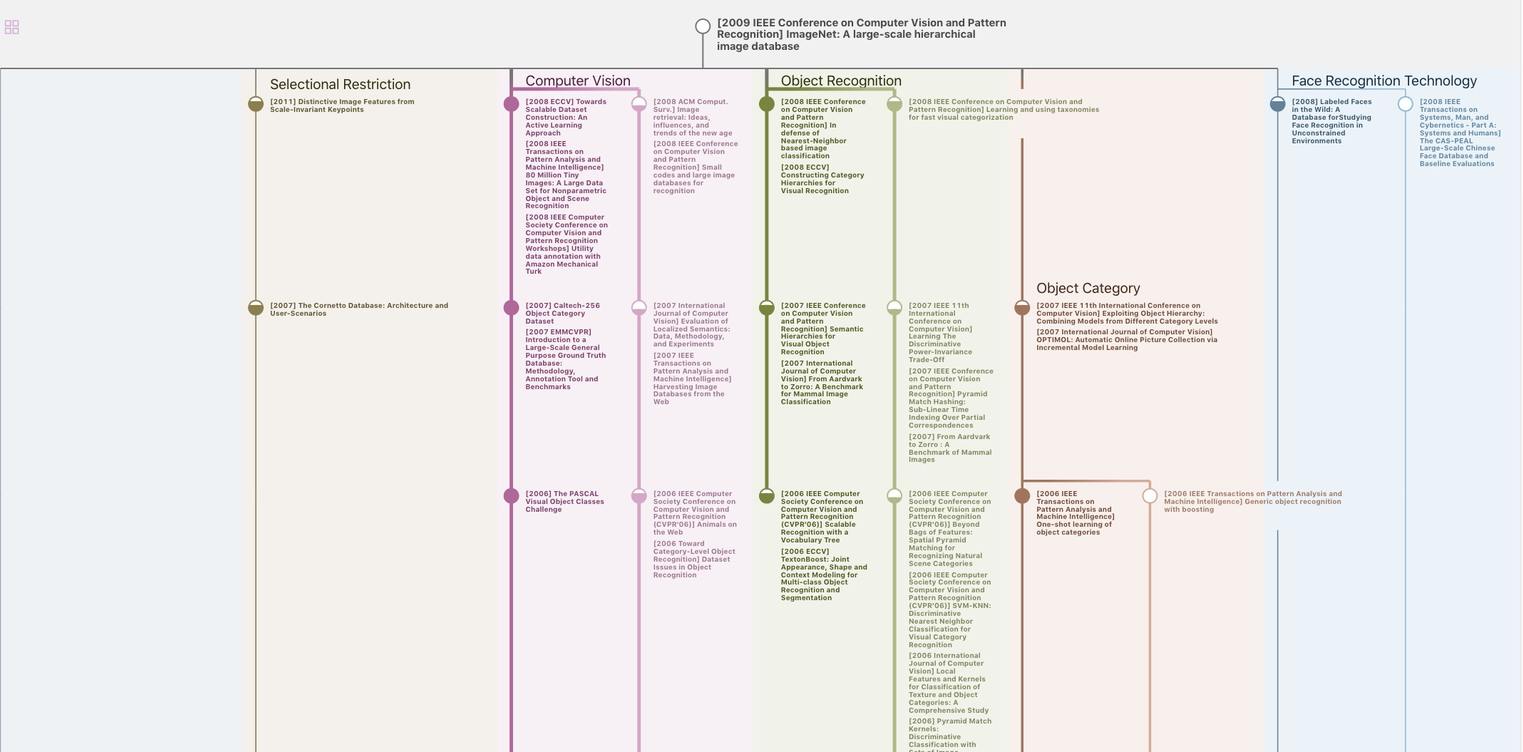
生成溯源树,研究论文发展脉络
Chat Paper
正在生成论文摘要