Improvements In Joint Domain-Range Modeling For Background Subtraction
PROCEEDINGS OF THE BRITISH MACHINE VISION CONFERENCE 2012(2012)
摘要
In many algorithms for background modeling, a distribution over feature values is modeled at each pixel. These models, however, do not account for the dependencies that may exist among nearby pixels. The joint domain- range kernel density estimate (KDE) model by Sheikh and Shah [7], which is not a pixel- wise model, represents the background and foreground processes by combining the three color dimensions and two spatial dimensions into a five- dimensional joint space. The Sheikh and Shah model, as we will show, has a peculiar dependence on the size of the image. In contrast, we build three- dimensional color distributions at each pixel and allow neighboring pixels to influence each other's distributions. Our model is easy to interpret, does not exhibit the dependency on image size, and results in higher accuracy. Also, unlike Sheikh and Shah, we build an explicit model of the prior probability of the background and the foreground at each pixel. Finally, we use the adaptive kernel variance method of Narayana et al. [5] to adapt the KDE covariance at each pixel. With a simpler and more intuitive model, we can better interpret and visualize the effects of the adaptive kernel variance method, while achieving accuracy comparable to state-of-the-art on a standard backgrounding benchmark.
更多查看译文
AI 理解论文
溯源树
样例
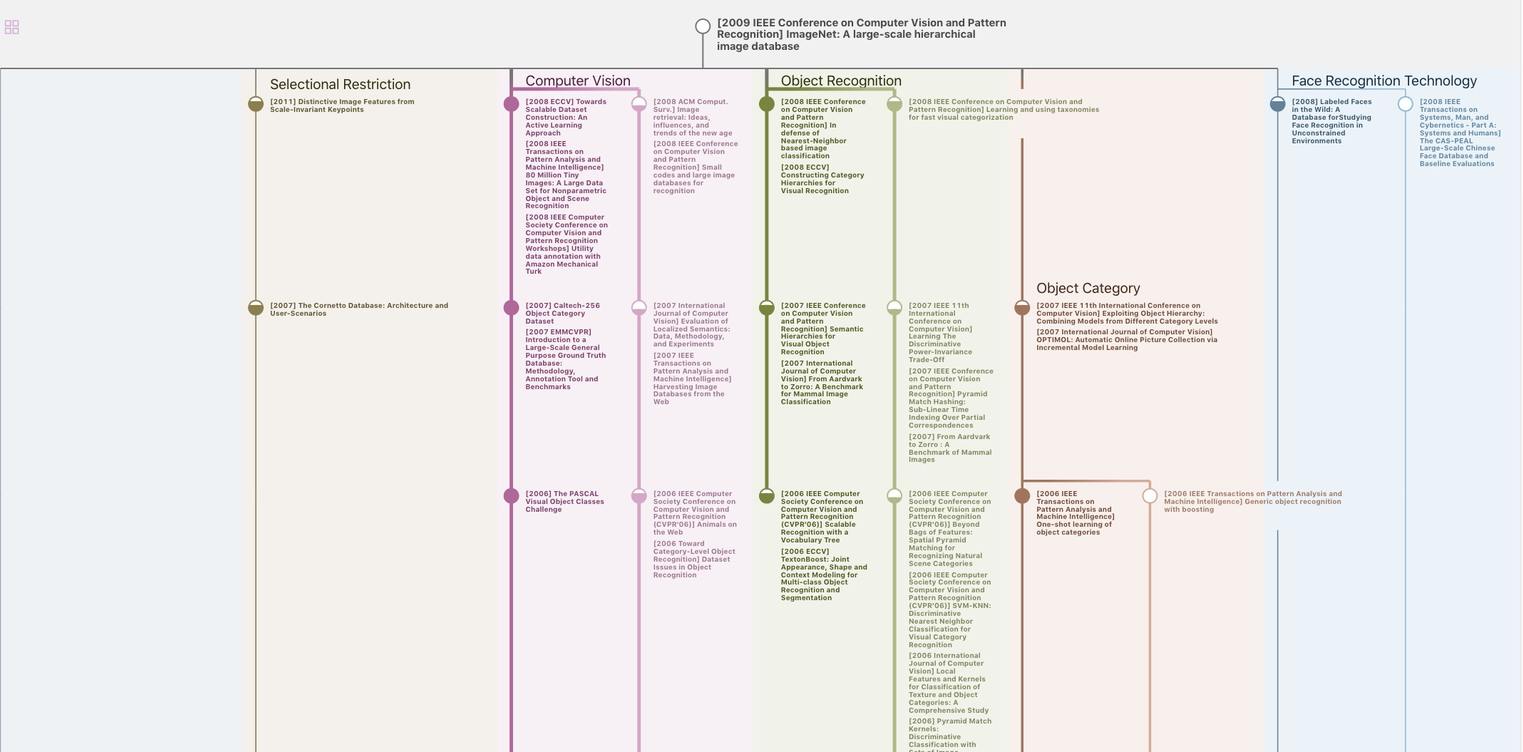
生成溯源树,研究论文发展脉络
Chat Paper
正在生成论文摘要