Bayesian Learning with Selective Subsets of Populations in Genetic Programming
msra
摘要
An interesting element in the forecasting of time series data is the choice of a predictive model for the underlying process. Of particular interest are predictive models based on Bayesian Networks organized according to a two- tiered approach that loosely follows the human cognitive model. Past work has shown that an evolutionary search for this type of Bayesian Network in which the fittest model is chosen as the representation of the underlying process model provides reasonable results in the prediction of both synthesized and stock market data. However, observation of the evolutionary process also indicates that prior to concentrating the search in the neighborhood of one best model, several very good models are generated and then discarded over time. In this paper, the authors explore the retention of such good models and their combined use under Bayesian Learning to provide a forecast of time series data. A method for selecting individual models is presented that builds a set of forecast models over multiple generations of the genetic programming search process. Experimental results in comparing the single best model to the combined model are presented with suggestions for future study related to defining the fitness of the genetic process to evolve better predictive sets.
更多查看译文
AI 理解论文
溯源树
样例
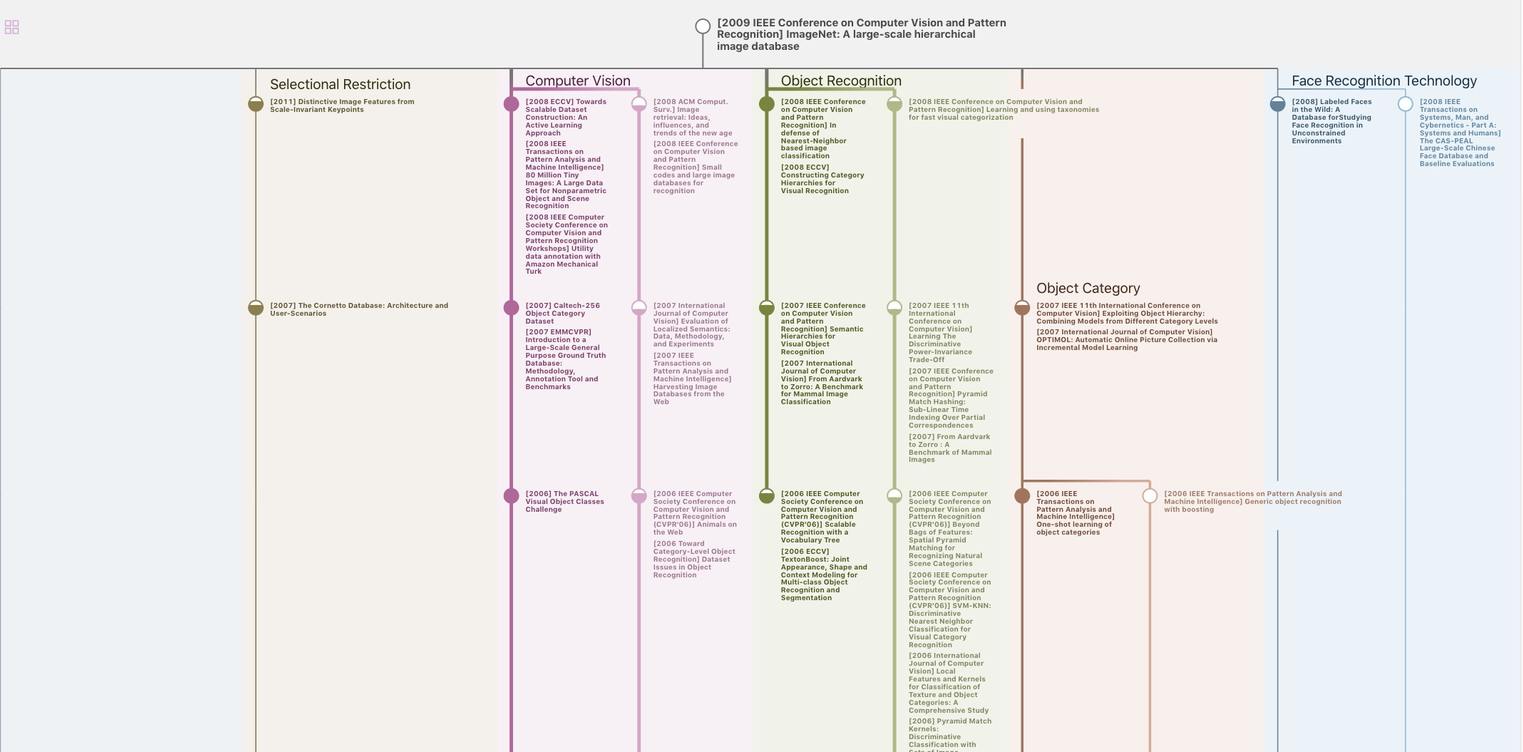
生成溯源树,研究论文发展脉络
Chat Paper
正在生成论文摘要