Research on the Heat Supply Prediction Method of a Heat Pump System Based on Timing Analysis and a Neural Network
Energy and Built Environment(2024)
Abstract
The prediction of heat pump system has more complicated characteristics, and the prediction accuracy of the existing single model is not ideal. From the perspective of energy efficiency and energy consumption, it is necessary to improve the accuracy of prediction. A sewage source heat pump system in Shenyang, China, was used as the research object in this paper. The ARIMA model, the BP neural network model, and the ARIMA-BP integrated model, were built. The accuracy of the predicted values of heat supply obtained by the models was verified. The prediction accuracy of the model was verified in extreme weather. The completeness of the model validation was improved. Three prediction models had been applied to the water source heat pump system and the soil source heat pump system. The adaptability and generalization of the model were verified. The number of training sets for heat supply prediction was divided. The number of training sets at the beginning of the heating season was analyzed. The results showed that the mean absolute percentage errors of the ARIMA model, BP neural network model and ARIMA-BP integrated model were 5.37 %, 5.97 % and 3.21 %, respectively. The root mean square errors were 177.31, 186.98, 139.44, respectively. The ARIMA-BP integrated model had a prediction accuracy that improved by 2.16 % compared to the ARIMA model. The ARIMA-BP integrated model had a prediction accuracy that improved by 2.76 % compared to the BP model. In extreme weather, the mean absolute percentage error was 7.83 %, the root mean square error was 296.42. The overall error was also within a reasonable range. The ARIMA-BP integrated model had high prediction accuracy and good applicability and generalization. At the beginning of the heating season, the heat supply can be better predicted when the number of training sets is 4 days.
MoreTranslated text
Key words
Heat supply prediction,Energy efficiency,ARIMA-BP integrated model,Training sets
AI Read Science
Must-Reading Tree
Example
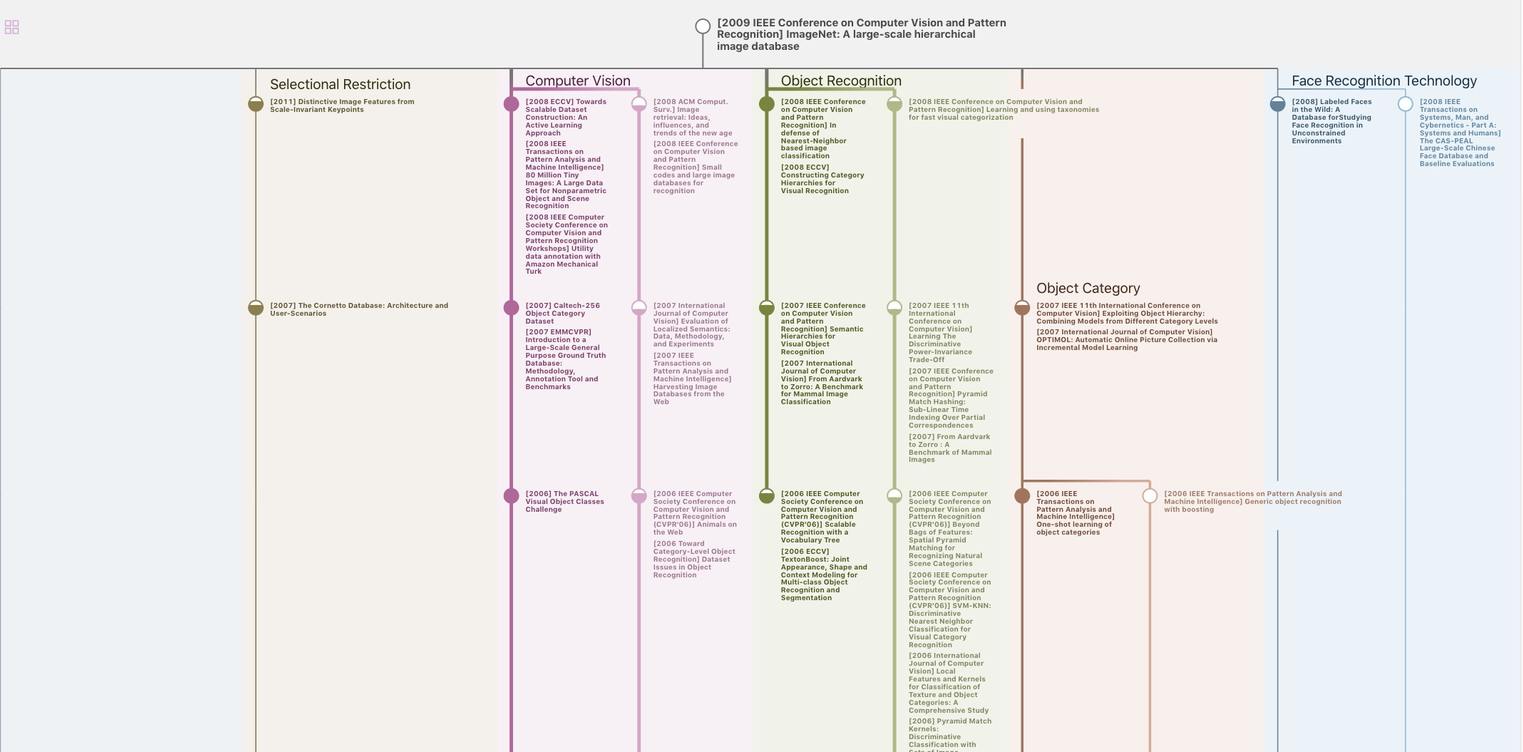
Generate MRT to find the research sequence of this paper
Chat Paper
Summary is being generated by the instructions you defined