Boundary extraction using supervised edgelet classification
OPTICAL ENGINEERING(2012)
摘要
Traditional learning-based boundary extraction algorithms classify each pixel edge separately and then get boundaries from the local decisions of a classifier. However, we propose a supervised learning method for boundary extraction by using edgelets as boundary elements. First, we extract edgelets by clustering probabilities of boundary. Second, we use features of edgelets to train a classifier that determines whether an edgelet belongs to a boundary. The classifier is trained by utilizing edgelet features, including local appearance, multiscale features, and global scene features such as saliency maps. Finally, we use the classifier to decide the probability that the edgelet belongs to the boundary. The experimental results in the Berkeley Segmentation Dataset demonstrate that our algorithm can improve the performance of boundary extraction. (C) 2012 Society of Photo-Optical Instrumentation Engineers (SPIE). [DOI: 10.1117/1.OE.51.1.017002]
更多查看译文
关键词
boundary extraction,edgelet,supervised learning,boosted decision tree,logistic regression
AI 理解论文
溯源树
样例
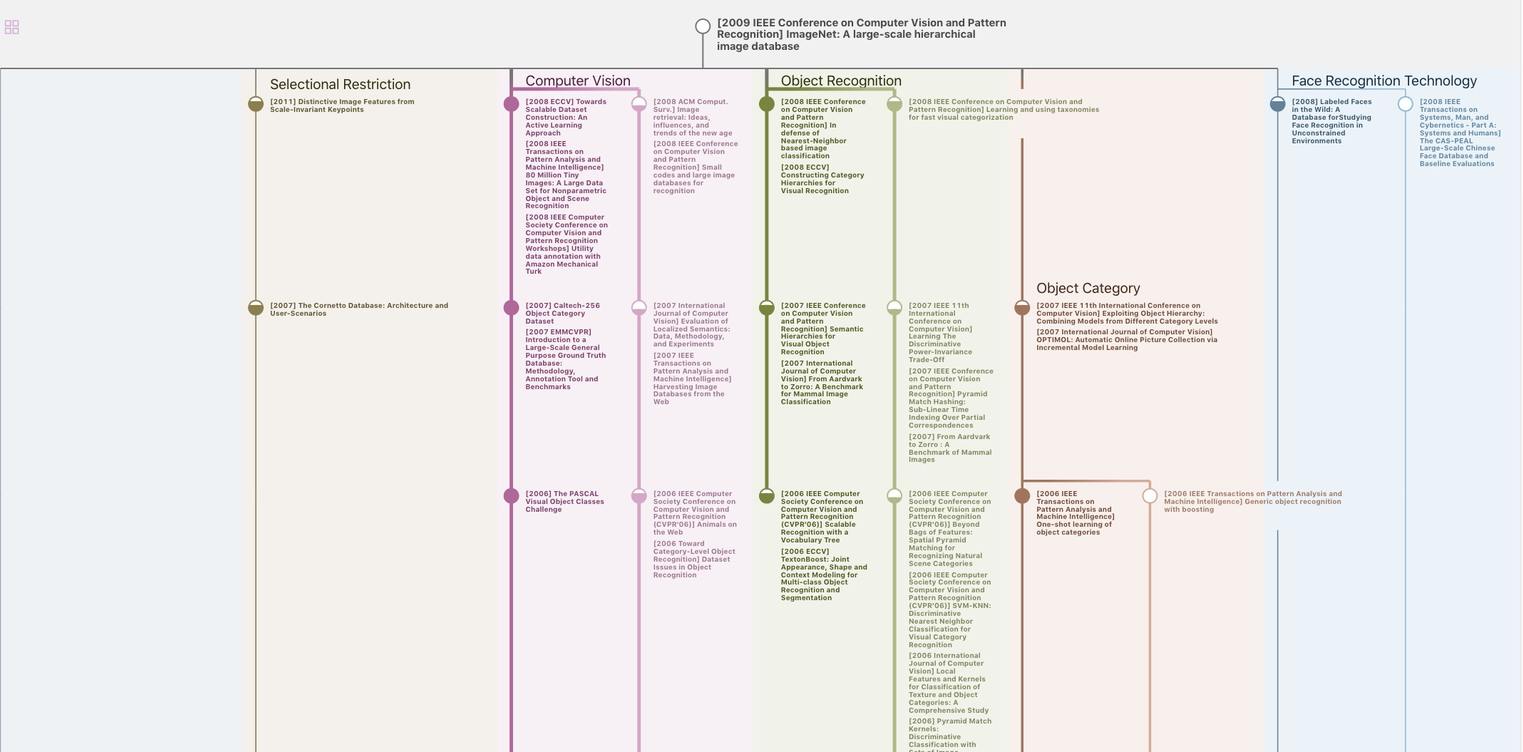
生成溯源树,研究论文发展脉络
Chat Paper
正在生成论文摘要