A Novel Remote Sensing Image Classification Scheme Based on Data Fusion, Multiple Features and Ensemble Learning
Journal of the Indian Society of Remote Sensing(2012)
摘要
In this paper, we propose a novel scheme to improve the accuracy of remote sensing image classification by integrating data fusion, multiple feature combination and ensemble learning. Intensity-Hue-Saturation (IHS), Gram-Schmidt (GS), Brovey and wavelet fusion methods are first performed to obtain the optimal fusion images of high resolution and multispectral images. Support Vector Machine (SVM) classifier is then adopted to classify the fused image with different feature sets, and ensemble learning algorithm based on dynamic classifier selection (DCS) is finally used to integrate multiple classification maps. The proposed classification scheme is implemented with three remote sensing data sets, obtaining the highest overall accuracy and kappa coefficient in all cases (92.63% and 0.8917 for BJ-1 data set, 81.89% and 0.7513 for Landsat TM and SPOT4 data set, 92.21% and 0.8838 for ALOS data set respectively). The experimental results show that the integration of data fusion, feature combination and ensemble learning improves the classification performance obviously and has great potential in practical uses.
更多查看译文
关键词
Data fusion,Remote sensing,Ensemble learning,Classification,Support vector machine (SVM),Dynamic classifier selection (DCS)
AI 理解论文
溯源树
样例
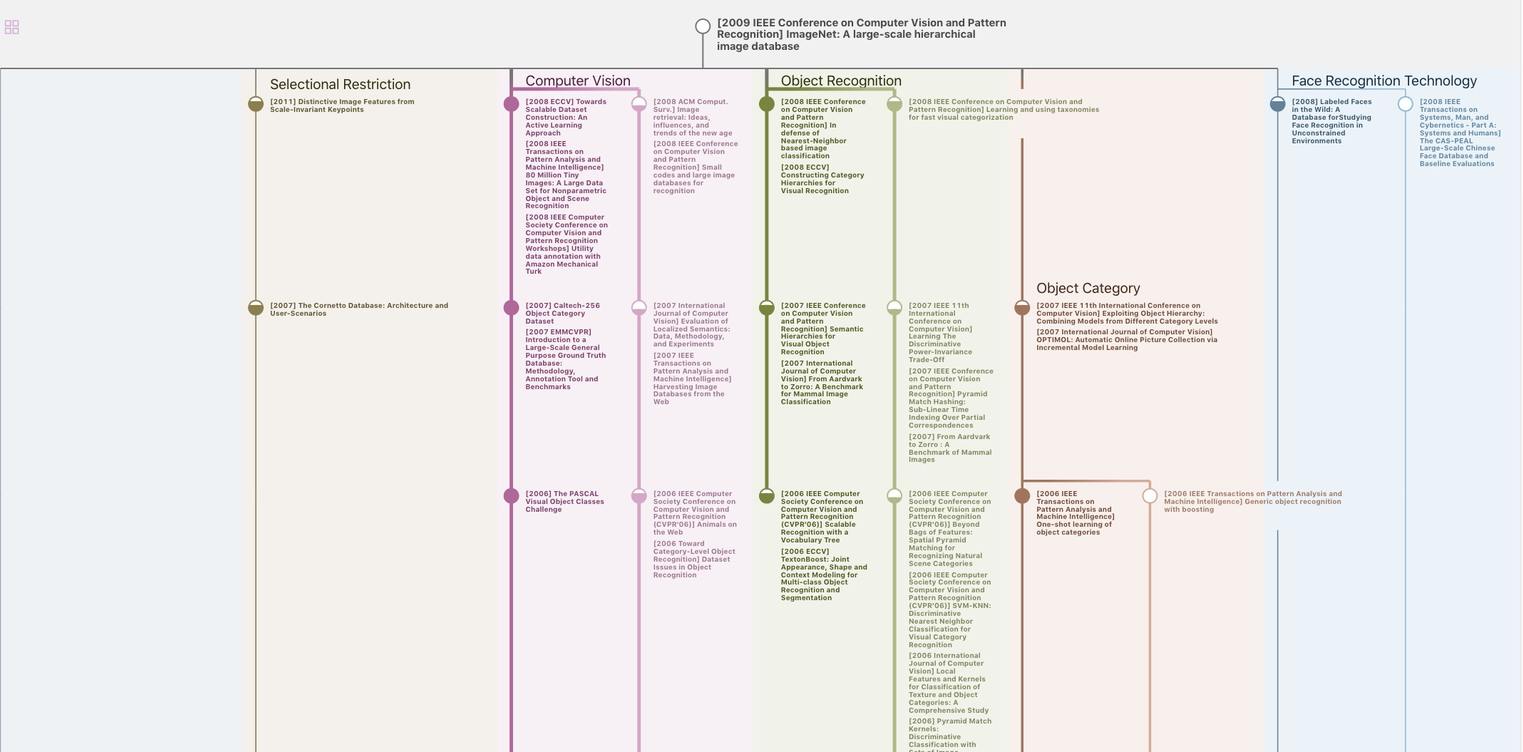
生成溯源树,研究论文发展脉络
Chat Paper
正在生成论文摘要