A composite method for feature selection of microarray data
JOURNAL OF COMPUTATIONAL AND THEORETICAL NANOSCIENCE(2014)
摘要
With rapid advancement of microarray technologies, biologists now can analysis thousands of gene expression values at one time. So they can have a 'global' view of the cell. Among all the problems during gene expression data analysis, the curse of dimensionality with thousands of gene expression values but only limited samples, commonly no more than one hundred, becomes more serious. The existence of numerous gene expression data, irrelevant to the classification of tumors, not only increases computational complexity but also makes the discovery of relevant genes impossible. Therefore, feature selection becomes critical important. In this paper, we first use a filter method to rank genes, and then select 'important' genes with high 'information exponential' score. Afterwards, we use a clustering method based on k-NN principle to find truly important genes. A support vector machine is applied to validate the classification performance of candidate genes. The experimental results demonstrate that our method can effective solve the problem caused by filter method, which doesn't consider the relationships among genes.
更多查看译文
关键词
Gene Expression Data,Filtering Method,Clustering Method,Support Vector Machine
AI 理解论文
溯源树
样例
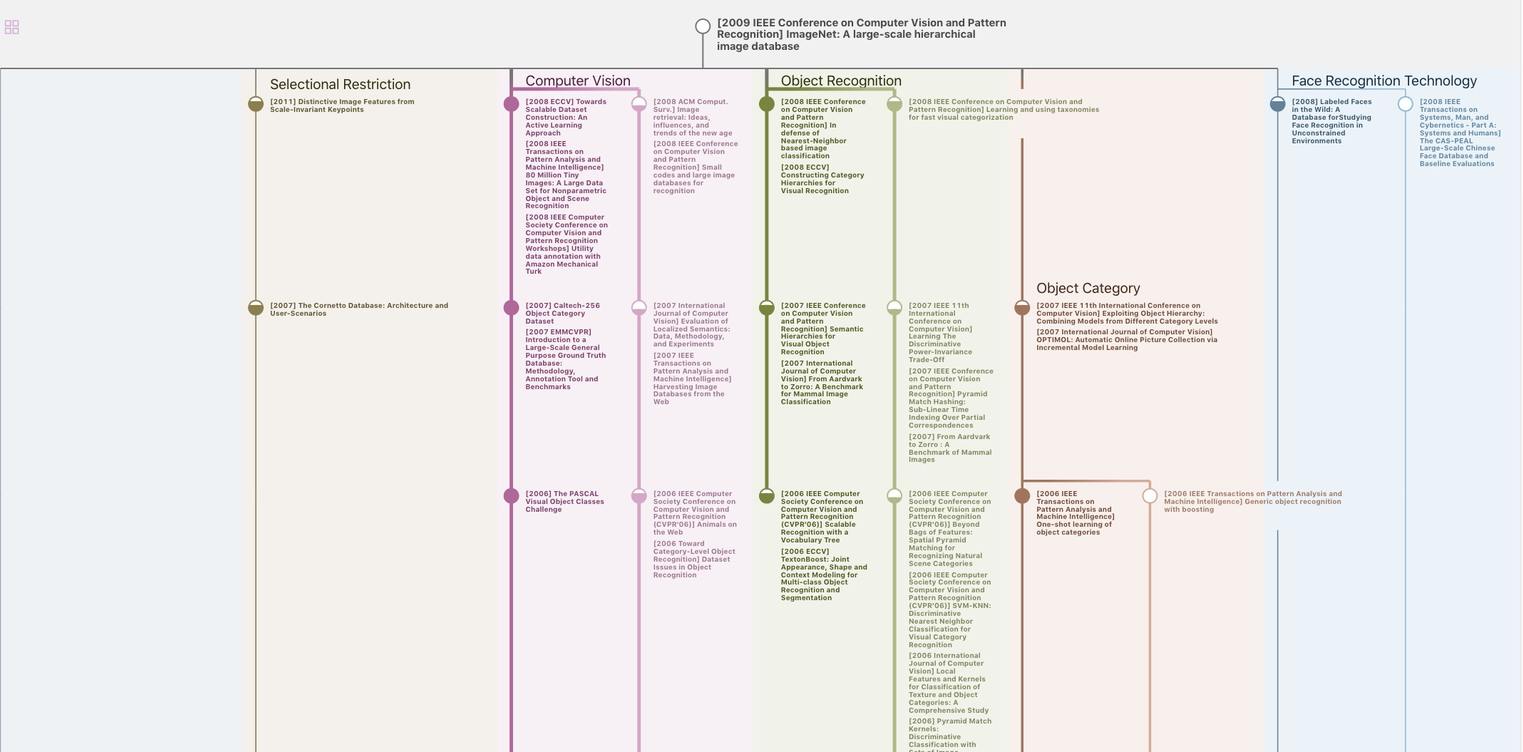
生成溯源树,研究论文发展脉络
Chat Paper
正在生成论文摘要