Texture feature extraction based on wavelet transform
ICCASM), 2010 International Conference(2010)
摘要
The 2-D lifting-based DWT 9/7 wavelet filter is used here, without additional computations, giving lifting-based architectures a significant advantage over convolutional filter band-based architectures. This paper describes the texture classification using (i) the known texture images are decomposed using 9/7 wavelet. Then, mean and standard deviation of approximation and detail sub-bands of 3- level decomposed images are calculated. They are wavelet statistical features (WSFs) (ii) In order to improve the correct classification rate further, it is proposed to find co-occurrence matrix features for original image, approximation and detail sub-bands of 1-level 9/7 wavelet decomposed images. The various co-occurrence features such as contrast, energy, entropy and homogeneity are calculated from the co-occurrence matrix. These are wavelet co-occurrence features (WCFs). (iii) At last, the combination of WSFs and WCFs (feature vector) are used to classify images.
更多查看译文
关键词
wavelet statistical features (wsfs),wavelet co-occurrence features (wcfs),feature extraction,wavelet transforms,cooccurrence matrix feature,2d lifting based dwt 9/7wavelet filter,wavelet decomposed image,lifting based architecture,texture classification,convolutional filter band based architecture,matrix algebra,3 level decomposed image,texture image,wavelet cooccurrence feature,image classification,wavelet transform,texture feature extraction,image texture,wavelet statistical feature,standard deviation,feature vector,image resolution,co occurrence matrix
AI 理解论文
溯源树
样例
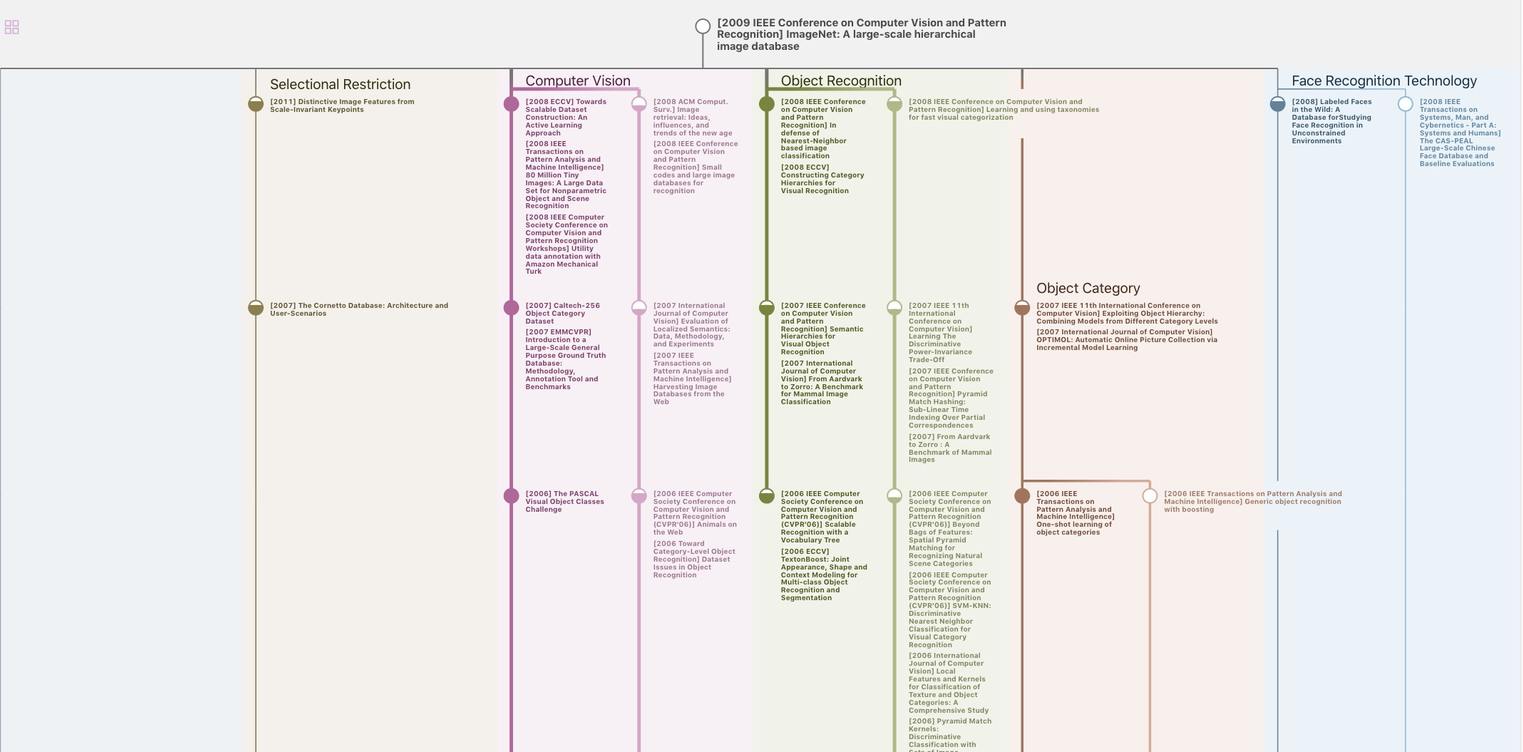
生成溯源树,研究论文发展脉络
Chat Paper
正在生成论文摘要