An algorithm for multi-label learning based on minimum threshold
Proceedings of the World Congress on Intelligent Control and Automation (WCICA)(2010)
摘要
Multi-label learning is a hot spot in machine learning and data mining. The multi-label learning model can predict one or more labels for a test instance. PT5 is a effective method to solving multi-label learning problem, it is critical to set an optimal threshold in this method. More error labels will be predicted if a lower threshold was set, and the labels will be predicted not entire if a big threshold was set. In this paper, a new algorithm TFEL (Threshold for Each Label) is proposed. An optimal threshold will be set for each label by learning from the training data set. When the score for a test instance to one label is bigger then threshold which is set for the label, the label will be added to the last classifying result for the test instance. The experiments results show that the TFEL method can get a well performance on classifying multi-label data set. © 2010 IEEE.
更多查看译文
关键词
data mining,minimum threshold,multi-label learning,iris,iris recognition,hot spot,diabetes,learning artificial intelligence,data models,machine learning
AI 理解论文
溯源树
样例
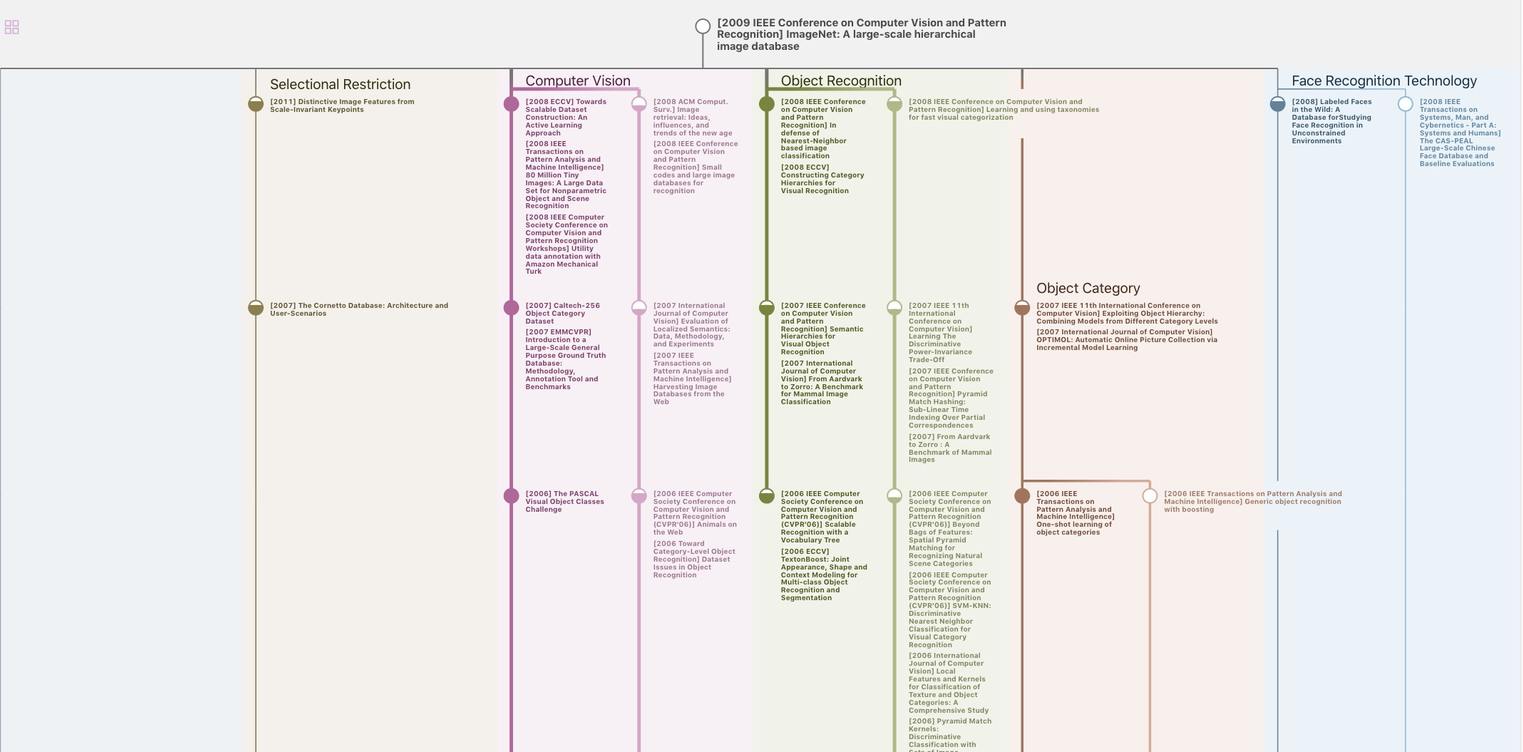
生成溯源树,研究论文发展脉络
Chat Paper
正在生成论文摘要