Modified MFCCs for robust speaker recognition
Proceedings - 2010 IEEE International Conference on Intelligent Computing and Intelligent Systems, ICIS 2010(2010)
摘要
Mel-scale frequency cepstrum coefficienls (MFCCs) are commonly used katues in speaker recognition systems, but MFCC values are not very robust in the presence of noise. thus, the modified MFCCs (named as SMN-CMN-MFCC) based on the general noisy speech model is proposed in this paper, which uses spectrum mean normalization (SMN) to suppress the additive noise, and uses cepstral mean normalization (CMN) to remove the effect of convolutional noise. Theoretical analyses show that the combination of SMN and CMN can inhibit additive and convolutional noise at the same time. To verify the performance of the SMN-CMN-MFCC, \\e have conducted some speaker recognition tesls. With the same convolutional noise component, the additive white noise experimenls and the additive factory noise experimenls show that SMN-CMN-MFCC provides 10.5% and 9.6% relative improvement than the conventional MFCC and ΔMFCC features, respectively. ©2010 IEEE.
更多查看译文
关键词
feature extraction,mel-scale frequency cepstral coefficients,speaker recognition,mel frequency cepstral coefficient,robustness,signal to noise ratio,convolution
AI 理解论文
溯源树
样例
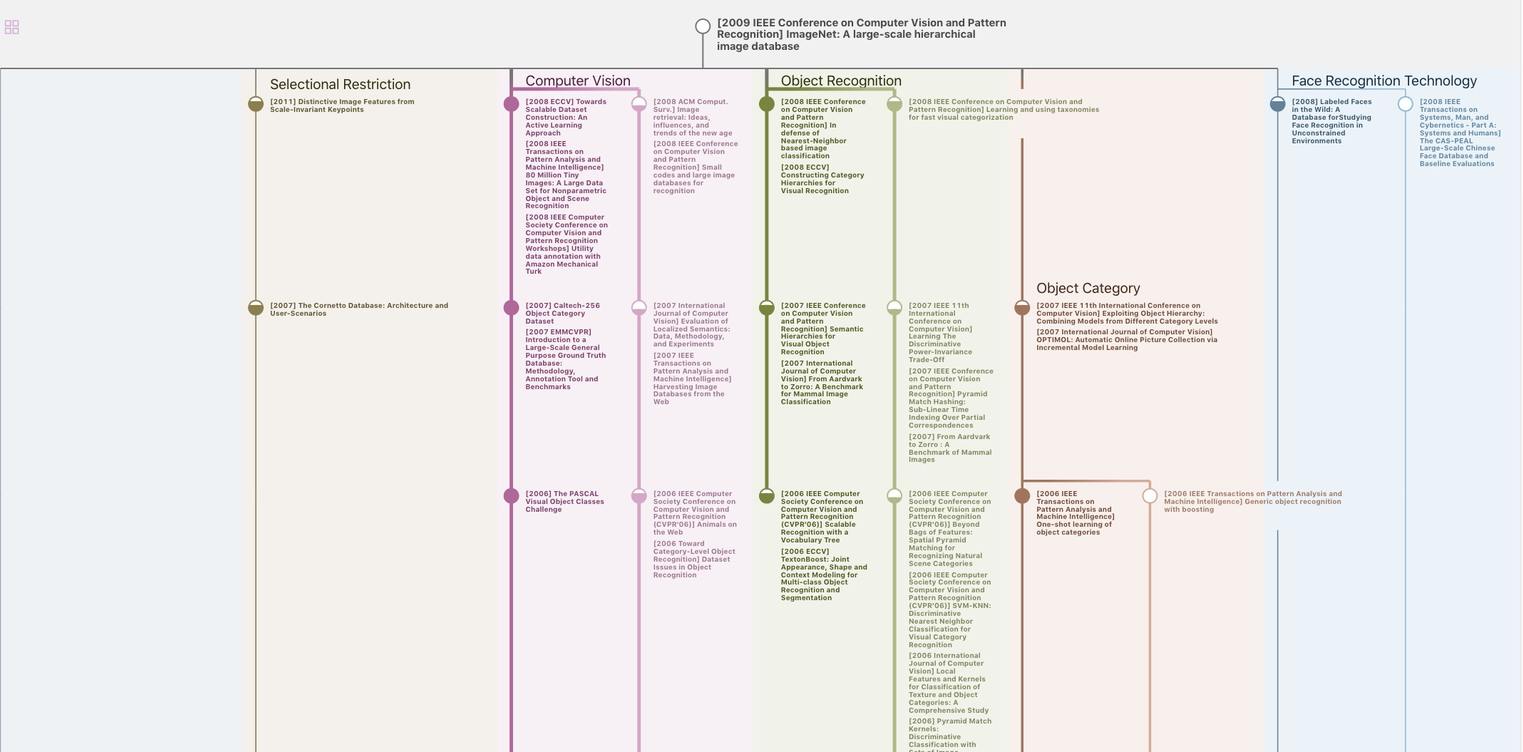
生成溯源树,研究论文发展脉络
Chat Paper
正在生成论文摘要