Multi-level Asymmetric Contrastive Learning for Volumetric Medical Image Segmentation Pre-training
arxiv(2023)
摘要
Medical image segmentation is a fundamental yet challenging task due to the
arduous process of acquiring large volumes of high-quality labeled data from
experts. Contrastive learning offers a promising but still problematic solution
to this dilemma. Because existing medical contrastive learning strategies focus
on extracting image-level representation, which ignores abundant multi-level
representations. And they underutilize the decoder either by random
initialization or separate pre-training from the encoder, thereby neglecting
the potential collaboration between the encoder and decoder. To address these
issues, we propose a novel multi-level asymmetric contrastive learning
framework named MACL for volumetric medical image segmentation pre-training.
Specifically, we design an asymmetric contrastive learning structure to
pre-train encoder and decoder simultaneously to provide better initialization
for segmentation models. Moreover, we develop a multi-level contrastive
learning strategy that integrates correspondences across feature-level,
image-level, and pixel-level representations to ensure the encoder and decoder
capture comprehensive details from representations of varying scales and
granularities during the pre-training phase. Finally, experiments on 12
volumetric medical image datasets indicate our MACL framework outperforms
existing 11 contrastive learning strategies. i.e. Our MACL achieves
a superior performance with more precise predictions from visualization figures
and 2.28%, 1.32%, 1.62% and 1.60% Average Dice higher than previous best
results on CHD, MMWHS, CHAOS and AMOS, respectively. And our MACL also has a
strong generalization ability among 5 variant U-Net backbones. Our code will be
available at https://github.com/stevezs315/MACL.
更多查看译文
AI 理解论文
溯源树
样例
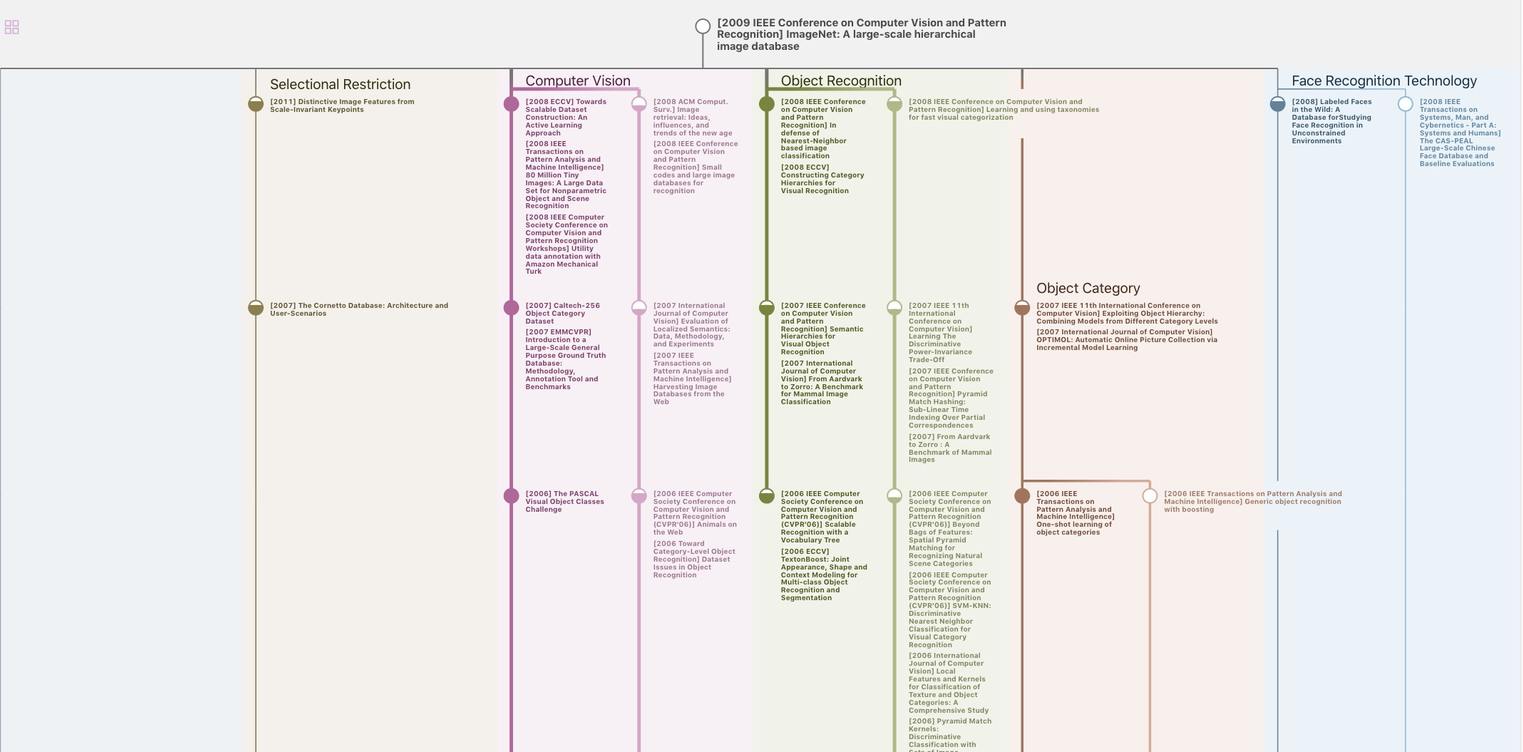
生成溯源树,研究论文发展脉络
Chat Paper
正在生成论文摘要