Robust multiple cameras pedestrian detection with multi-view Bayesian network
Pattern Recognition(2015)
摘要
Multi-camera pedestrian detection is the challenging problem in the field of surveillance video analysis. However, existing approaches may produce "phantoms" (i.e., fake pedestrians) due to the heavy occlusions in real surveillance scenario, while calibration errors and the diverse heights of pedestrians may also heavily decrease the detection performance. To address these problems, this paper proposes a robust multiple cameras pedestrian detection approach with multi-view Bayesian network model (MvBN). Given the preliminary results obtained by any multi-view pedestrian detection method, which are actually comprised of both real pedestrians and phantoms, the MvBN is used to model both the occlusion relationship and the homography correspondence between them in all camera views. As such, the removal of phantoms can be formulated as an MvBN inference problem. Moreover, to reduce the influence of the calibration errors and keep robust to the diverse heights of pedestrians, a height-adaptive projection (HAP) method is proposed to further improve the detection performance by utilizing a local search process in a small neighborhood of heights and locations of the detected pedestrians. Experimental results on four public benchmarks show that our method outperforms several state-of-the-art algorithms remarkably and demonstrates high robustness in different surveillance scenes. HighlightsA multi-view Bayesian network is proposed to model pedestrian candidates and their occlusion relationships in all views.A parameter learning algorithm is developed for MvBN by using a set of auxiliary, real-valued, and continuous variables.A height-adaptive projection is proposed to make the final detection robust to synthesis noises and calibration errors.Our approach is recognized as the best performer in five PETS evaluations from 2009 to 2013.
更多查看译文
关键词
bayesian inference
AI 理解论文
溯源树
样例
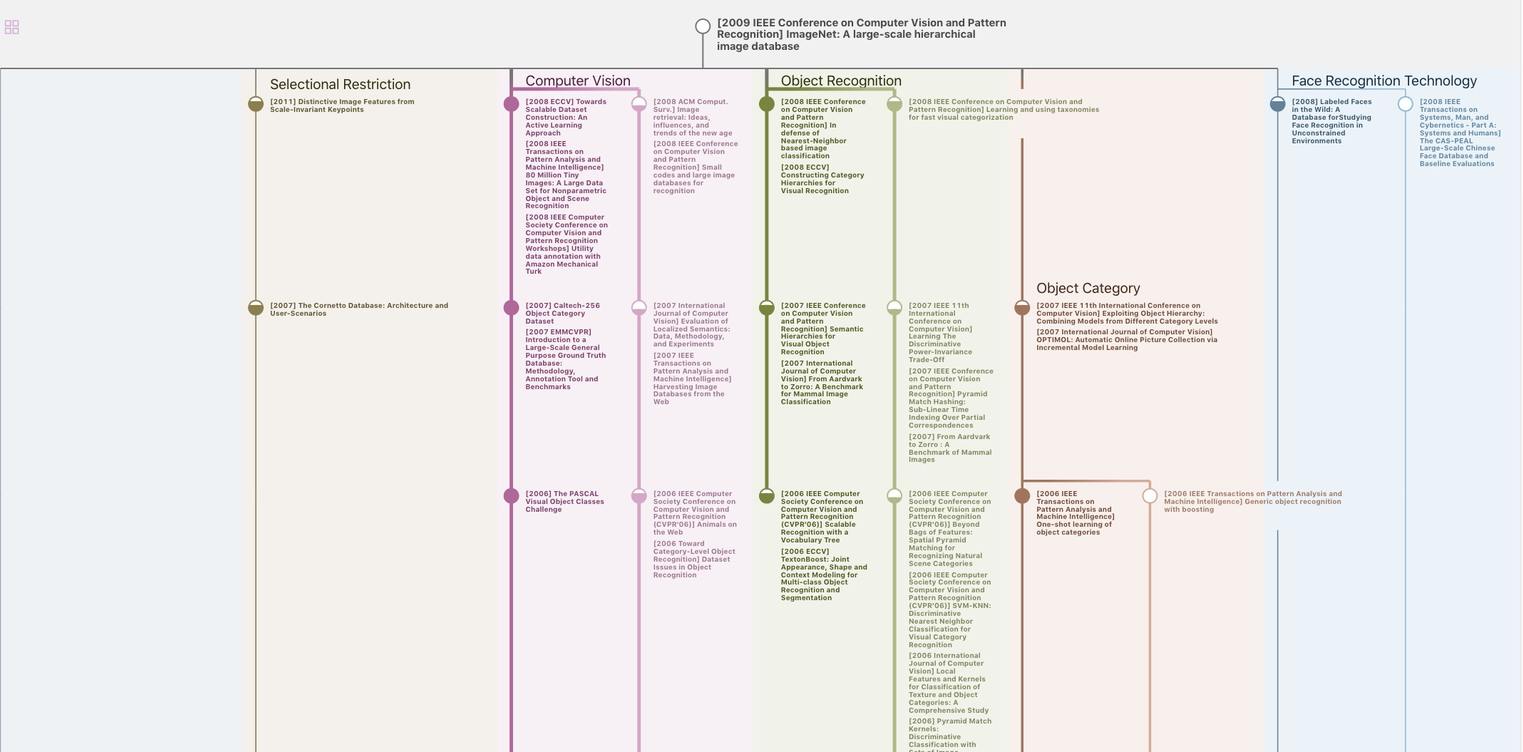
生成溯源树,研究论文发展脉络
Chat Paper
正在生成论文摘要