Graph-based local concept coordinate factorization
Knowl. Inf. Syst.(2013)
摘要
Ubiquitous data are increasingly expanding in large volumes due to human activities, and grouping them into appropriate clusters is an important and yet challenging problem. Existing matrix factorization techniques have shown their significant power in solving this problem, e.g., nonnegative matrix factorization, concept factorization. Recently, one state-of-the-art method called locality-constrained concept factorization is put forward, but its locality constraint does not well reveal the intrinsic data structure since it only requires the concept to be as close to the original data points as possible. To address this issue, we present a graph-based local concept coordinate factorization (GLCF) method, which respects the intrinsic structure of the data through manifold kernel learning in the warped Reproducing Kernel Hilbert Space. Besides, a generalized update algorithm is developed to handle data matrices containing both positive and negative entries. Since GLCF is essentially based on the local coordinate coding and concept factorization, it inherits many advantageous properties, such as the locality and sparsity of the data representation. Moreover, it can better encode the locally geometrical structure via graph Laplacian in the manifold adaptive kernel. Therefore, a more compact and better structured representation can be obtained in the low-dimensional data space. Extensive experiments on several image and gene expression databases suggest the superiority of the proposed method in comparison with some alternatives.
更多查看译文
关键词
Manifold kernel learning,Local coordinate coding,Graph Laplacian,Concept factorization,Clustering
AI 理解论文
溯源树
样例
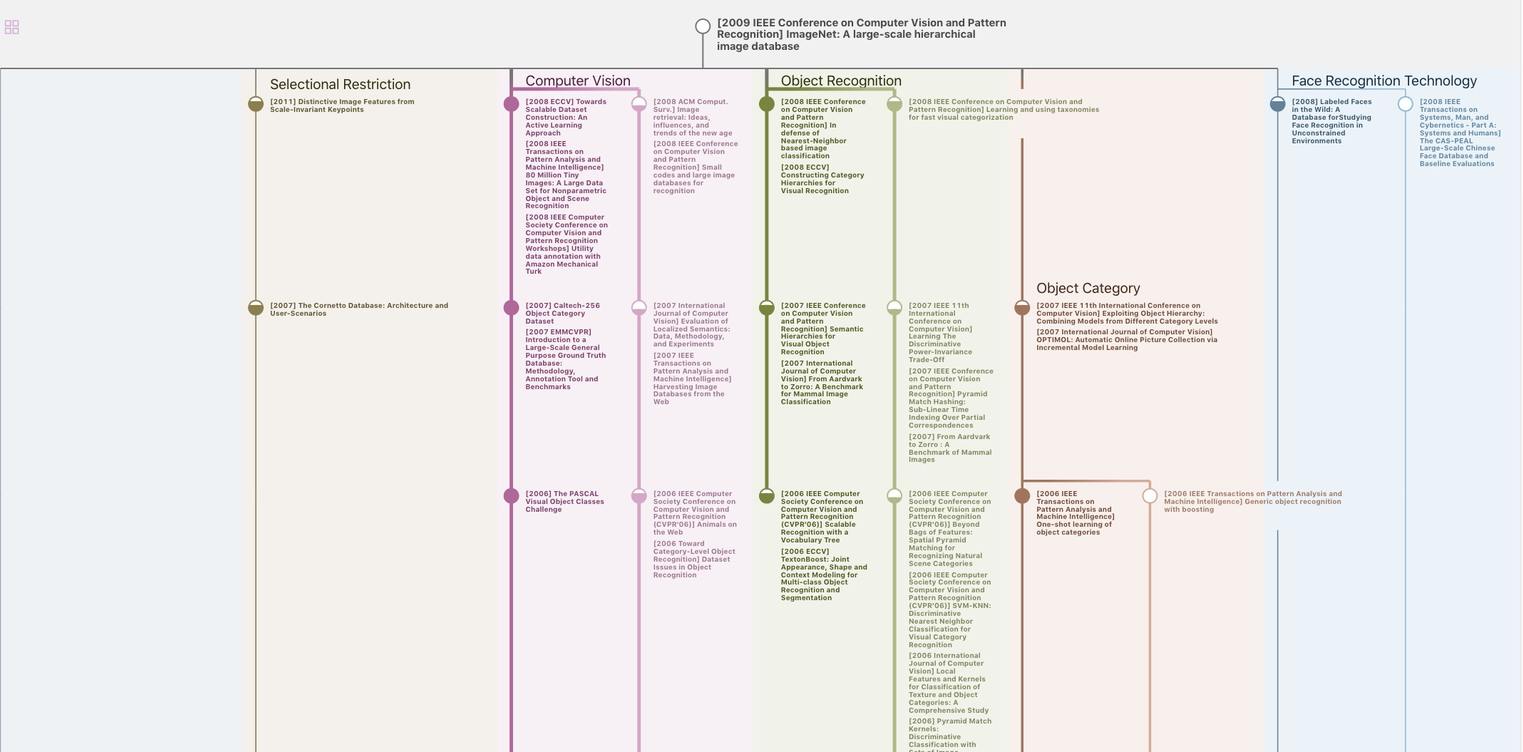
生成溯源树,研究论文发展脉络
Chat Paper
正在生成论文摘要