Learning to suggest questions in social media
Knowl. Inf. Syst.(2014)
摘要
Social media systems with Q&A functionalities have accumulated large archives of questions and answers. Two representative types are online forums and community-based Q&A services. To enable users to explore the large number of questions and answers in social media systems effectively, it is essential to suggest interesting items to an active user. In this article, we address the problem of question suggestion, which targets at suggesting questions that are semantically related to a queried question. Existing bag-of-words approaches suffer from the shortcoming that they could not bridge the lexical chasm between semantically related questions. Therefore, we present a new framework, and propose the topic-enhanced translation-based language model (TopicTRLM), which fuses both the lexical and latent semantic knowledge. This fusing enables TopicTRLM to find semantically related questions to a given question even when there is little word overlap. Moreover, to incorporate the answer information into the model to make the model more complete, we also propose the topic-enhanced translation-based language model with answer ensemble. Extensive experiments have been conducted with real-world datasets. Experimental results indicate our approach is very effective and outperforms other popular methods in several metrics.
更多查看译文
关键词
Social media,Online forum,Community-based Q&A,Question suggestion,Language model,Topic modeling
AI 理解论文
溯源树
样例
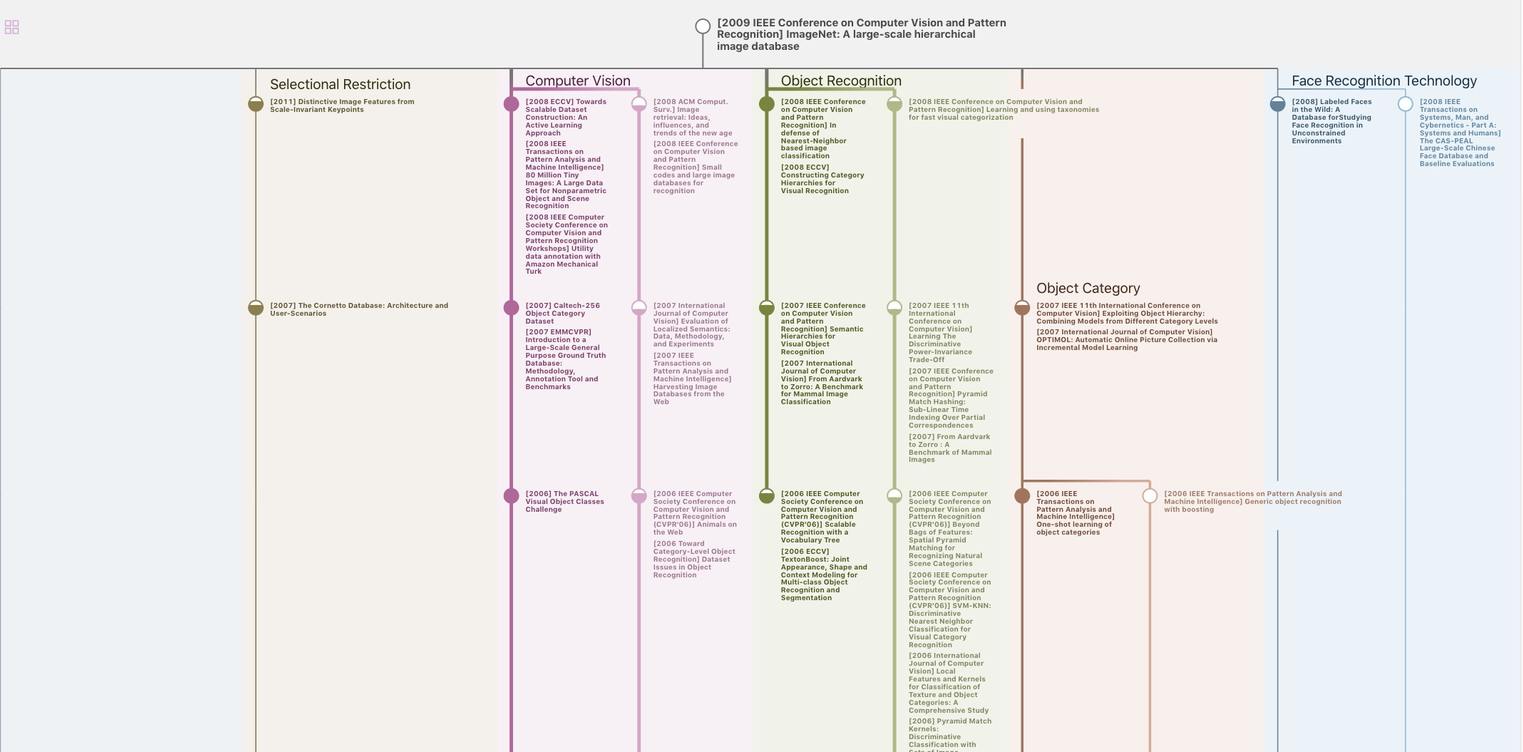
生成溯源树,研究论文发展脉络
Chat Paper
正在生成论文摘要