State-Clustering Based Multiple Deep Neural Networks Modeling Approach for Speech Recognition
IEEE/ACM Transactions on Audio, Speech & Language Processing(2015)
摘要
The hybrid deep neural network (DNN) and hidden Markov model (HMM) has recently achieved dramatic performance gains in automatic speech recognition (ASR). The DNN-based acoustic model is very powerful but its learning process is extremely time-consuming. In this paper, we propose a novel DNN-based acoustic modeling framework for speech recognition, where the posterior probabilities of HMM states are computed from multiple DNNs (mDNN), instead of a single large DNN, for the purpose of parallel training towards faster turnaround. In the proposed mDNN method all tied HMM states are first grouped into several disjoint clusters based on data-driven methods. Next, several hierarchically structured DNNs are trained separately in parallel for these clusters using multiple computing units (e.g. GPUs). In decoding, the posterior probabilities of HMM states can be calculated by combining outputs from multiple DNNs. In this work, we have shown that the training procedure of the mDNN under popular criteria, including both frame-level cross-entropy and sequence-level discriminative training, can be parallelized efficiently to yield significant speedup. The training speedup is mainly attributed to the fact that multiple DNNs are parallelized over multiple GPUs and each DNN is smaller in size and trained by only a subset of training data. We have evaluated the proposed mDNN method on a 64-hour Mandarin transcription task and the 320-hour Switchboard task. Compared to the conventional DNN, a 4-cluster mDNN model with similar size can yield comparable recognition performance in Switchboard (only about 2% performance degradation) with a greater than 7 times speed improvement in CE training and a 2.9 times improvement in sequence training, when 4 GPUs are used.
更多查看译文
关键词
multiple deep neural networks modeling,state clustering,sequence-level discriminative training,mdnn method,mandarin transcription task,cross entropy training,gpu,speech recognition,frame-level cross-entropy,time 64 hour,hmm,graphics processing units,sequence training,multiple dnns (mdnn),switchboard task,state-clustering,asr,deep neural networks (dnn),speech recognition equipment,hidden markov model,model parallelism,dnn-based acoustic model,parallel training,hidden markov models,hybrid deep neural network,time 320 hour,neural nets,data partition,automatic speech recognition,speech,computational modeling,acoustics,training data
AI 理解论文
溯源树
样例
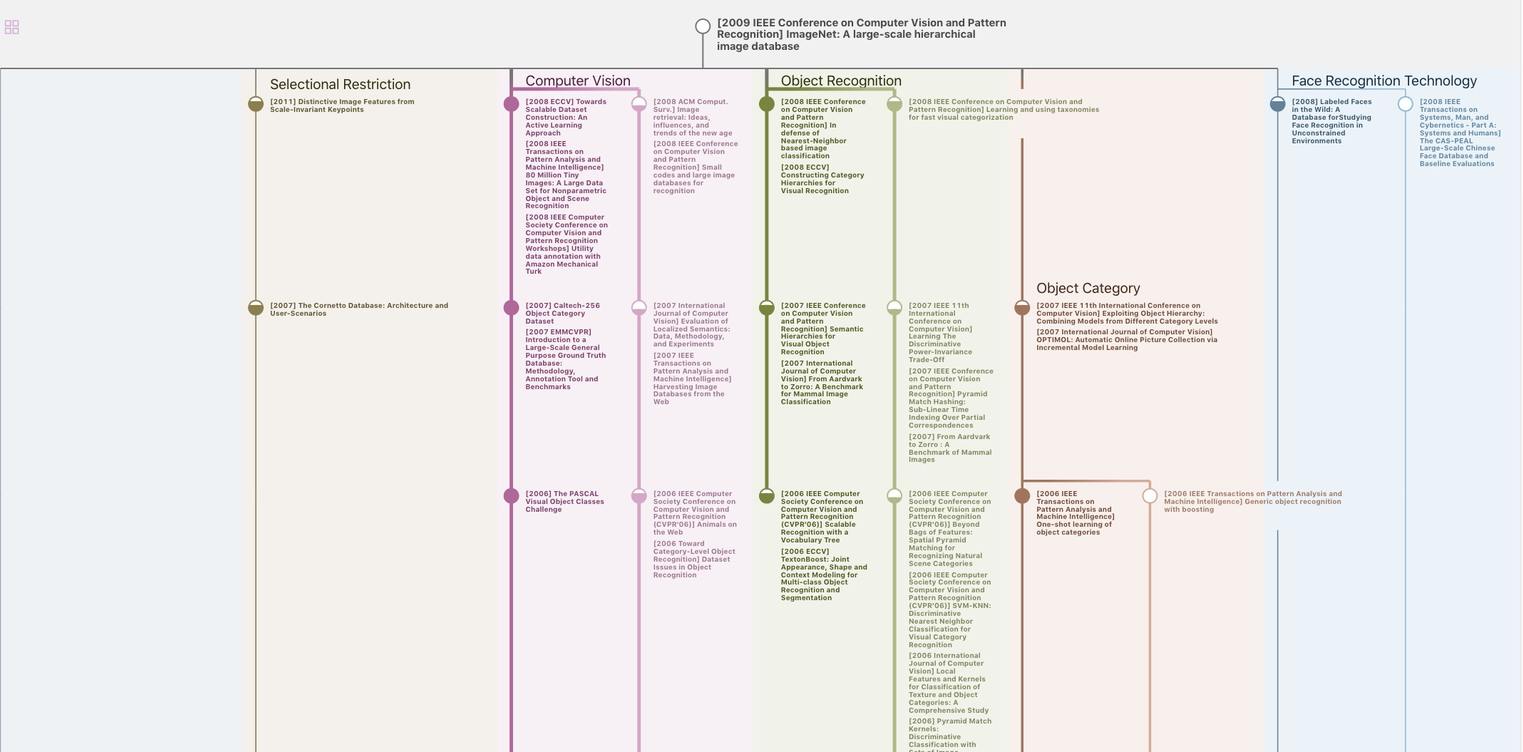
生成溯源树,研究论文发展脉络
Chat Paper
正在生成论文摘要