Recognition of Mental Workload Levels Under Complex Human–Machine Collaboration by Using Physiological Features and Adaptive Support Vector Machines
IEEE T. Human-Machine Systems(2015)
摘要
In order to detect human operator performance degradation or breakdown, this paper proposes an adaptive support vector machine-based method to classify operator mental workload (MWL) into few discrete levels based on psychophysiological measures. Electroencephalogram, electrocardiogram, and electrooculography signals were recorded continuously while the operator was performing safety-critical process control operations in a simulated human-machine system. In coarse-grained analysis, the adaptive exponential smoothing (AES) technique is used to smooth the psychophysiological data and to remove strong artifacts without requiring templates. The MWL level is classified every 30 s by using bounded support vector machine (BSVM) and tenfold cross-validation techniques. Locality preservation projection (LPP) technique is utilized to derive salient psychophysiological features by means of feature reduction. By combining the AES-LPP and BSVM methods, the accuracy of the coarse-grained MWL classification was significantly improved by 11-13%. On the other hand, to perform MWL classification with higher temporal resolution and cross-subject and cross-trial generalizability, finer-grained data analysis is also conducted to recognize MWL levels every 5 s based on a combination of adaptive BSVM (ABSVM) and AES techniques. In comparison with the use of the BSVM algorithm alone, a significant performance improvement by 10-20% is achieved by using the AES-ABSVM method in the finer-grained MWL classification.
更多查看译文
关键词
electrooculography,mental workload (mwl),classification,adaptive exponential smoothing,man-machine systems,adaptive support vector machines,complex human-machine collaboration,physiology,man–machine system,electroencephalogram,physiological features,operator mental workload,psychophysiology,man???machine system,electrocardiogram,machine learning,human operator performance degradation,mental workload levels recognition,support vector machines,accuracy,electroencephalography,data analysis
AI 理解论文
溯源树
样例
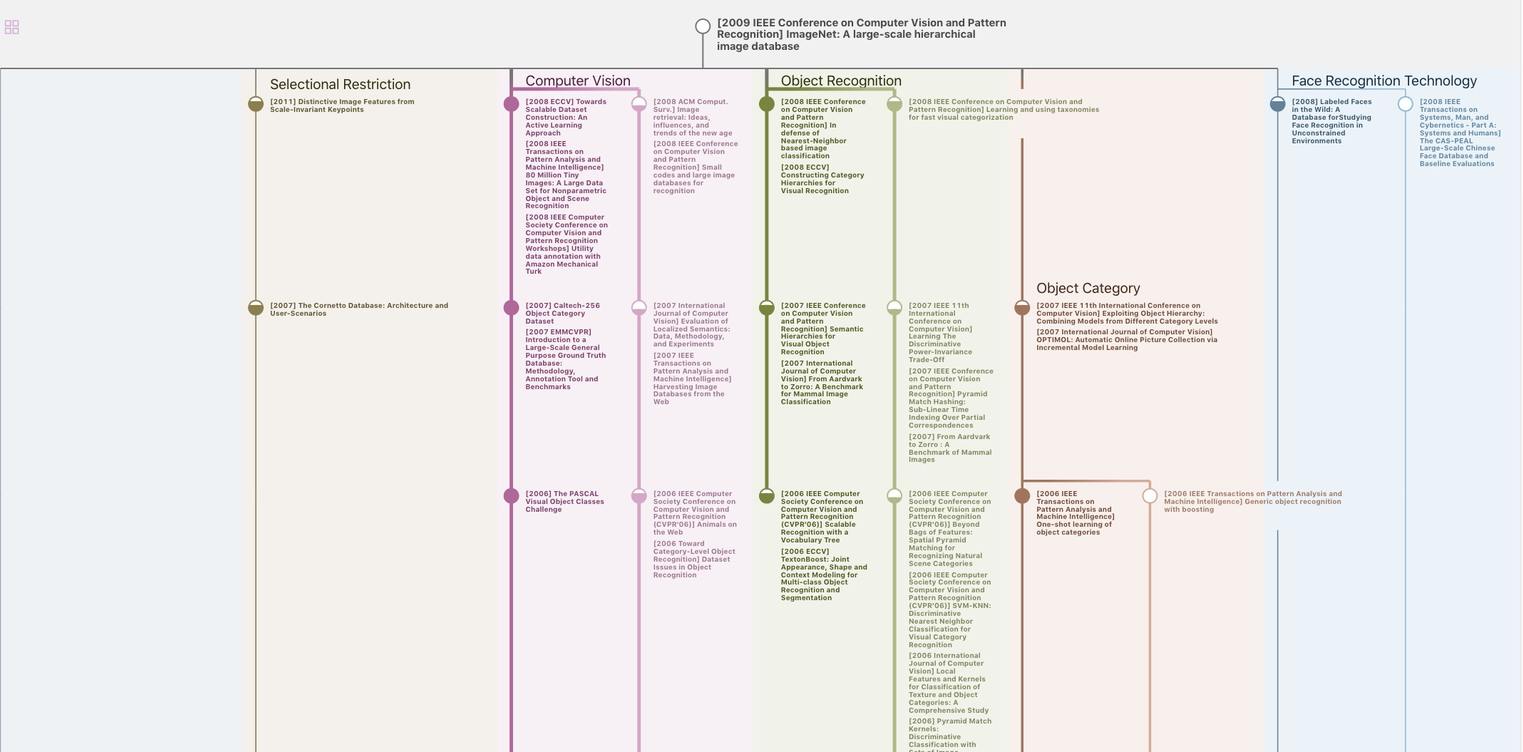
生成溯源树,研究论文发展脉络
Chat Paper
正在生成论文摘要