Robust representation and recognition of facial emotions using extreme sparse learning.
IEEE Transactions on Image Processing(2015)
摘要
Recognition of natural emotions from human faces is an interesting topic with a wide range of potential applications, such as human-computer interaction, automated tutoring systems, image and video retrieval, smart environments, and driver warning systems. Traditionally, facial emotion recognition systems have been evaluated on laboratory controlled data, which is not representative of the environment faced in real-world applications. To robustly recognize the facial emotions in real-world natural situations, this paper proposes an approach called extreme sparse learning, which has the ability to jointly learn a dictionary (set of basis) and a nonlinear classification model. The proposed approach combines the discriminative power of extreme learning machine with the reconstruction property of sparse representation to enable accurate classification when presented with noisy signals and imperfect data recorded in natural settings. In addition, this paper presents a new local spatio-temporal descriptor that is distinctive and pose-invariant. The proposed framework is able to achieve the state-of-the-art recognition accuracy on both acted and spontaneous facial emotion databases.
更多查看译文
关键词
facial emotion recognition,recognition accuracy,image representation,facial emotion,face recognition,acted facial emotion databases,facial emotion recognition systems,learning (artificial intelligence),discriminative power,visual databases,reconstruction property,smart environments,dictionary learning,driver warning systems,automated tutoring systems,emotion recognition,image classification,image retrieval,local spatiotemporal descriptor,real-world natural situations,laboratory controlled data,extreme learning machine,nonlinear classification model,spontaneous facial emotion databases,pose-invariance,extreme sparse learning,video retrieval,sparse representation,human-computer interaction,feature extraction,head,dictionaries,human computer interaction,vectors,learning artificial intelligence,robustness,image reconstruction
AI 理解论文
溯源树
样例
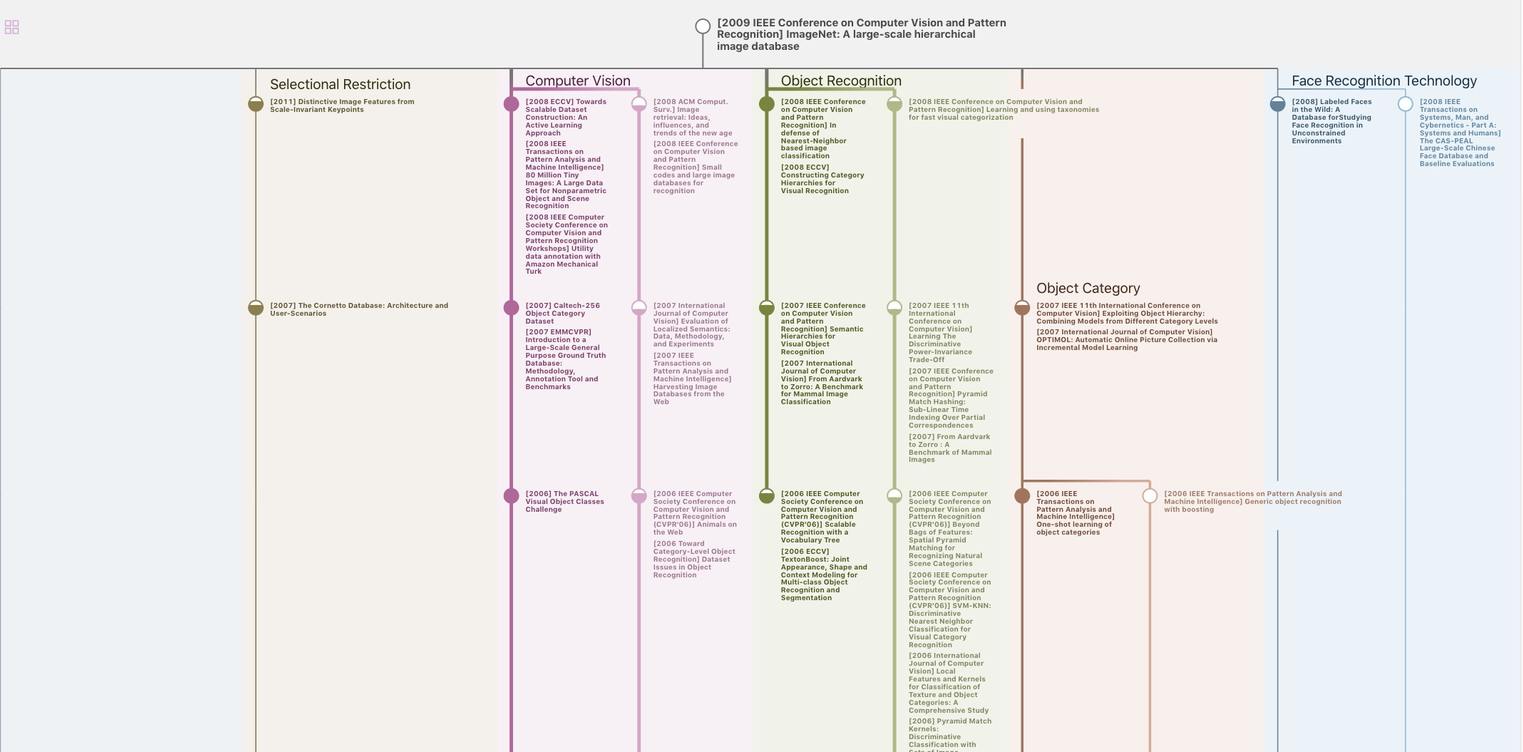
生成溯源树,研究论文发展脉络
Chat Paper
正在生成论文摘要