Combining ordered subsets and momentum for accelerated X-ray CT image reconstruction.
IEEE Trans. Med. Imaging(2015)
摘要
Statistical X-ray computed tomography (CT) reconstruction can improve image quality from reduced dose scans, but requires very long computation time. Ordered subsets (OS) methods have been widely used for research in X-ray CT statistical image reconstruction (and are used in clinical PET and SPECT reconstruction). In particular, OS methods based on separable quadratic surrogates (OS-SQS) are massively parallelizable and are well suited to modern computing architectures, but the number of iterations required for convergence should be reduced for better practical use. This paper introduces OS-SQS-momentum algorithms that combine Nesterov's momentum techniques with OS-SQS methods, greatly improving convergence speed in early iterations. If the number of subsets is too large, the OS-SQS-momentum methods can be unstable, so we propose diminishing step sizes that stabilize the method while preserving the very fast convergence behavior. Experiments with simulated and real 3D CT scan data illustrate the performance of the proposed algorithms.
更多查看译文
关键词
os-sqs-momentum algorithms,diagnostic radiography,computerised tomography,computed tomography (ct),clinical pet reconstruction,x-ray ct statistical image reconstruction,parallelizable iterative algorithms,statistical analysis,ordered subsets,separable quadratic surrogates,stochastic gradient,clinical spect reconstruction,momentum,parallelizable iterative algorithm,image reconstruction,statistical image reconstruction,convergence of numerical methods,ordered subset method based separable quadratic surrogates,combine nesterov momentum techniques,relaxation,fast convergence behavior,iterative methods,medical image processing,bioinformatics,biomedical research
AI 理解论文
溯源树
样例
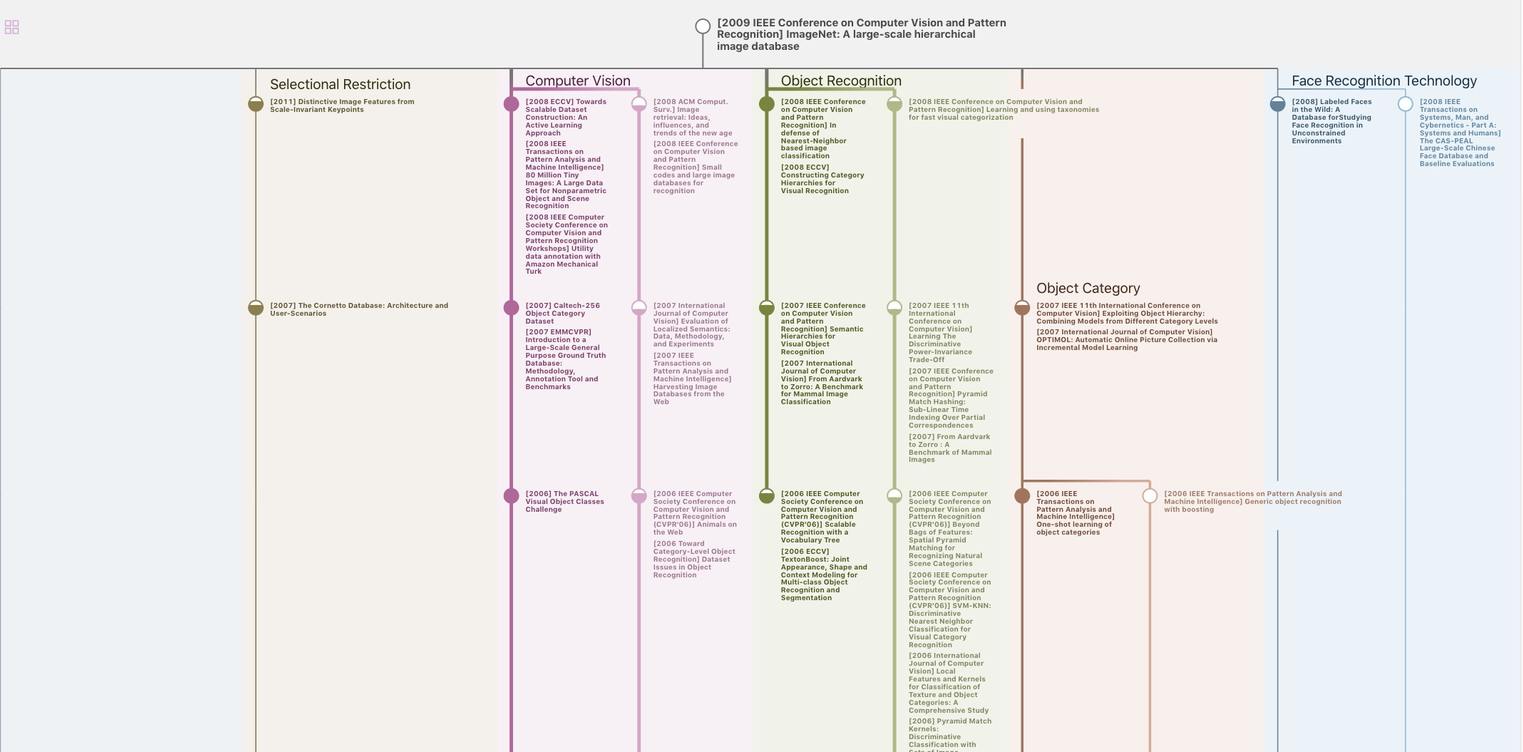
生成溯源树,研究论文发展脉络
Chat Paper
正在生成论文摘要