Passive Image-Splicing Detection by a 2-D Noncausal Markov Model
IEEE Trans. Circuits Syst. Video Techn.(2015)
摘要
In this paper, a 2-D noncausal Markov model is proposed for passive digital image-splicing detection. Different from the traditional Markov model, the proposed approach models an image as a 2-D noncausal signal and captures the underlying dependencies between the current node and its neighbors. The model parameters are treated as the discriminative features to differentiate the spliced images from the natural ones. We apply the model in the block discrete cosine transformation domain and the discrete Meyer wavelet transform domain, and the cross-domain features are treated as the final discriminative features for classification. The support vector machine which is the most popular classifier used in the image-splicing detection is exploited in our paper for classification. To evaluate the performance of the proposed method, all the experiments are conducted on public image-splicing detection evaluation data sets, and the experimental results have shown that the proposed approach outperforms some state-of-the-art methods.
更多查看译文
关键词
block discrete cosine transformation domain,wavelet transforms,discrete meyer wavelet transform domain,discrete meyer wavelet transform,passive image-splicing detection,2d noncausal markov model,passive digital image-splicing detection,markov processes,discrete cosine transforms,2-d noncausal markov model,image classification,support vector machine,2d noncausal signal,block discrete cosine transformation (bdct),discriminative features,support vector machines,splicing,computational modeling,hidden markov models,feature extraction
AI 理解论文
溯源树
样例
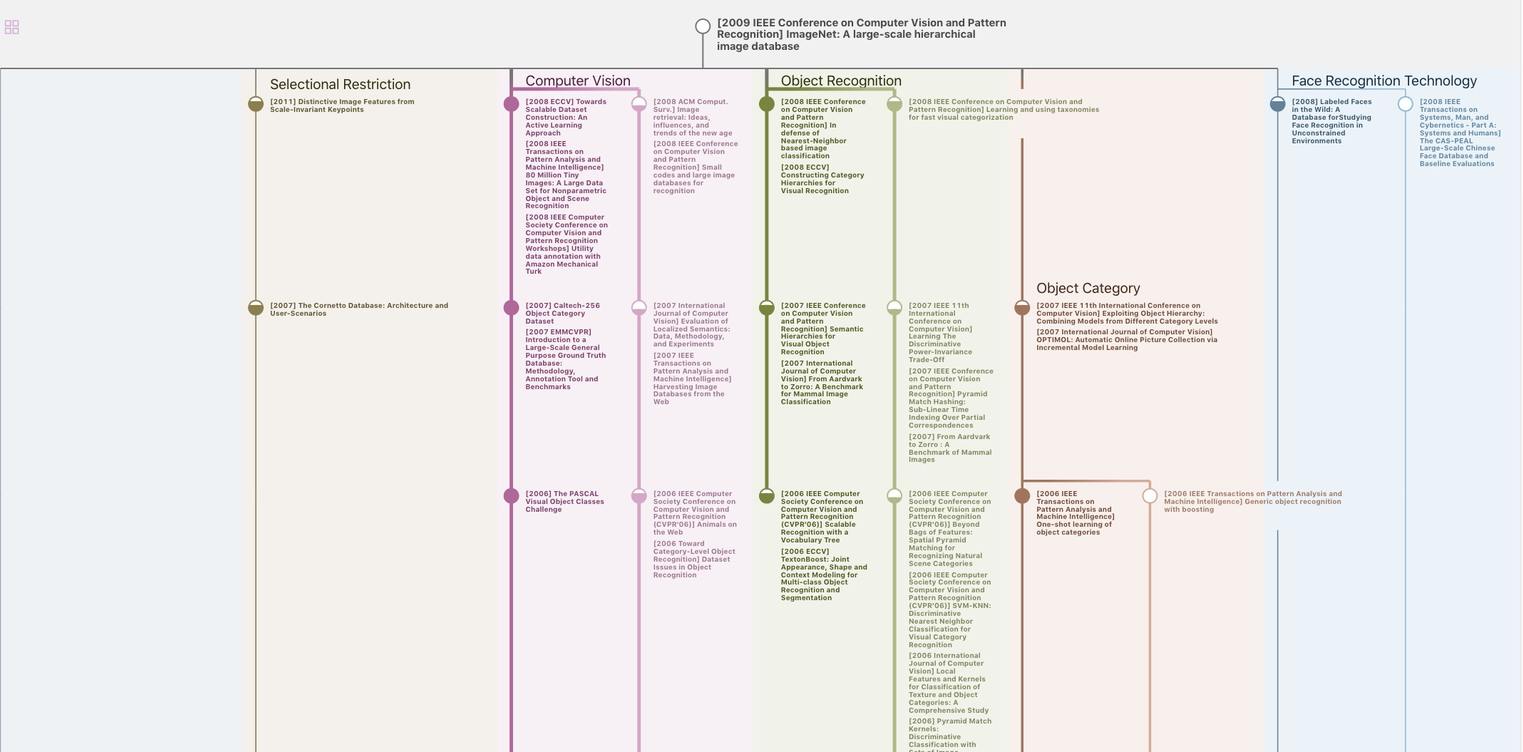
生成溯源树,研究论文发展脉络
Chat Paper
正在生成论文摘要