Robust Framework of Single-Frame Face Superresolution Across Head Pose, Facial Expression, and Illumination Variations
IEEE T. Human-Machine Systems(2015)
摘要
This paper presents a robust framework to solve the face hallucination problem across multiple factors, i.e., different expressions, head poses, and illuminations. It proposes a redundant transformation with diagonal loading for modeling the mappings among different new face factors, and a local reconstruction with geometry and position constraints for incorporating image details in the new factor spaces. Our proposed redundant and sparse strategies are discussed, and the experiments indicate that it is not necessary to adopt sparse representation in the proposed framework. The experimental results demonstrate that the proposed framework offers robustness when dealing with the inputs that have different expressions, head poses, and illuminations compared with the state-of-the-art methods, can generate high-resolution face images with better image qualities than the hierarchical tensor-based method, and improves the state of the art from single one output to multiple outputs with new factors.
更多查看译文
关键词
robust framework,face recognition,position constraints,illumination variations,diagonal loading,face hallucination problem,face superresolution,superresolution,single-frame face superresolution,facial expression,head pose,face hallucination,geometry,lighting,image reconstruction,robustness,image resolution,face
AI 理解论文
溯源树
样例
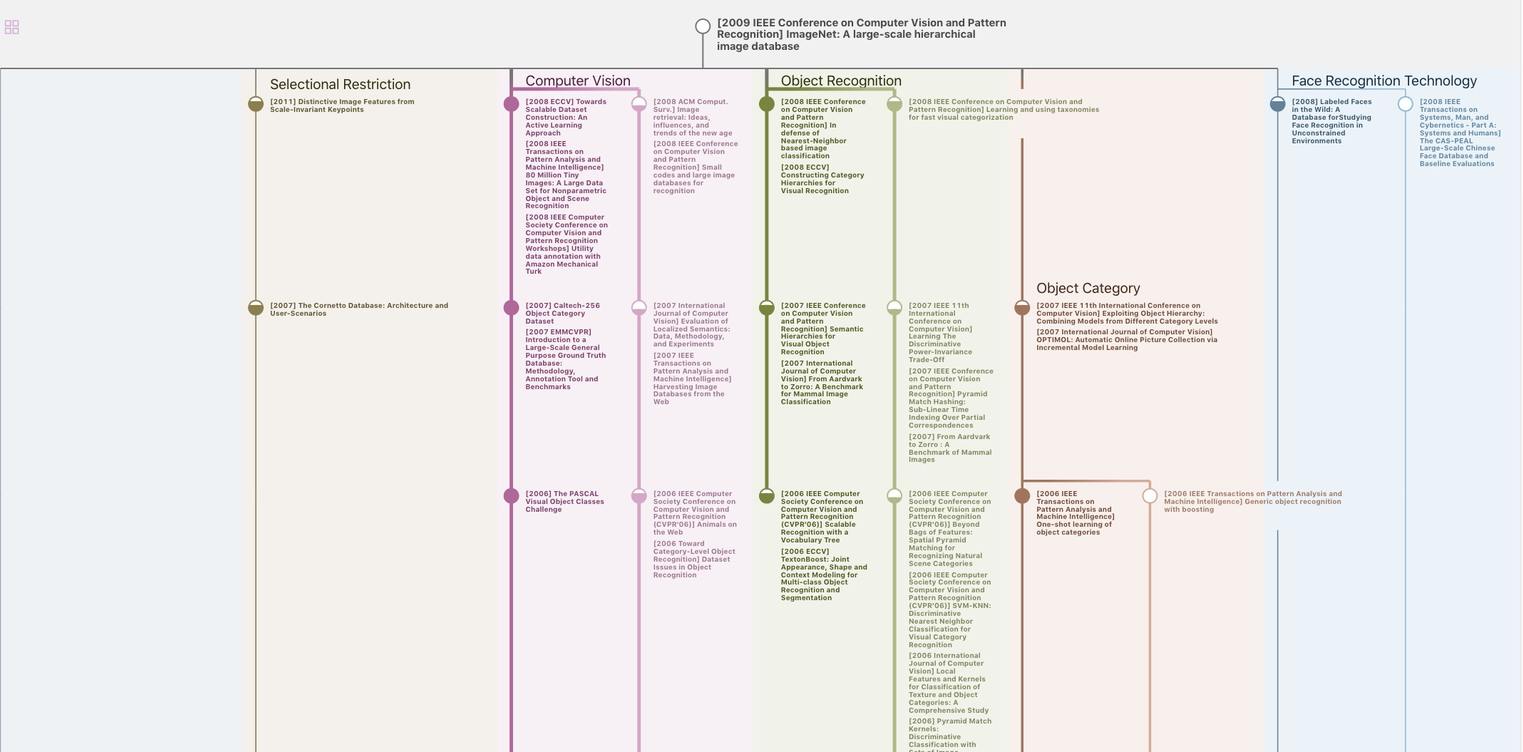
生成溯源树,研究论文发展脉络
Chat Paper
正在生成论文摘要