Unsupervised Feature Extraction Inspired by Latent Low-Rank Representation
WACV(2015)
摘要
Latent Low-Rank Representation (Lat LRR) has the empirical capability of identifying "salient" features. However, the reason behind this feature extraction effect is still not understood. Its optimization leads to non-unique solutions and has high computational complexity, limiting its potential in practice. We show that Lat LRR learns a transformation matrix which suppresses the most significant principal components corresponding to the largest singular values while preserving the details captured by the components with relatively smaller singular values. Based on this, we propose a novel feature extraction method which directly designs the transformation matrix and has similar behavior to Lat LRR. Our method has a simple analytical solution and can achieve better performance with little computational cost. The effectiveness and efficiency of our method are validated on two face recognition datasets.
更多查看译文
关键词
image representation,lat lrr,matrix algebra,unsupervised feature extraction,latent low-rank representation,feature extraction,transformation matrix learning,salient feature identification,unsupervised learning,principal component analysis,algorithm design and analysis,approximation algorithms,optimization,noise
AI 理解论文
溯源树
样例
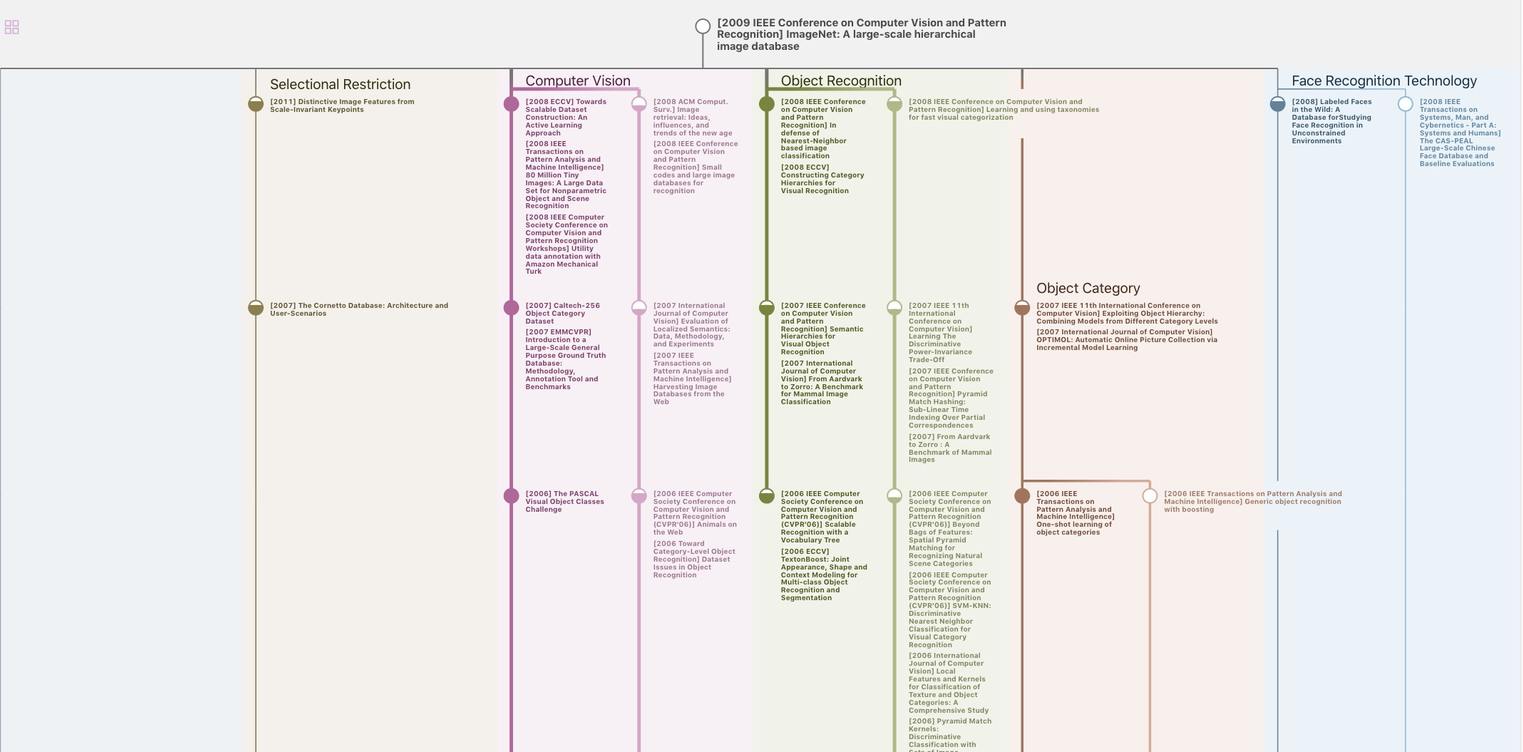
生成溯源树,研究论文发展脉络
Chat Paper
正在生成论文摘要