A Scalable Method for Exact Sampling from Kronecker Family Models
Data Mining(2014)
摘要
The recent interest in modeling complex networks has fueled the development of generative graph models, such as Kronecker Product Graph Model (KPGM) and mixed KPGM (mKPGM). The Kronecker family of models are appealing because of their elegant fractal structure, as well as their ability to capture important network characteristics such as degree, diameter, and (in the case of mKPGM) clustering and population variance. In addition, scalable sampling algorithms for KPGMs made the analysis of large-scale, sparse networks feasible for the first time. In this work, we show that the scalable sampling methods, in contrast to prior belief, do not in fact sample from the underlying KPGM distribution and often result in sampling graphs that are very unlikely. To address this issue, we develop a new representation that exploits the structure of Kronecker models and facilitates the development of novel grouped sampling methods that are provably correct. In this paper, we outline efficient algorithms to sample from mKPGMs and KPGMs based on these ideas. Notably, our mKPGM algorithm is the first available scalable sampling method for this model and our KPGM algorithm is both faster and more accurate than previous scalable methods. We conduct both theoretical analysis and empirical evaluation to demonstrate the strengths of our algorithms and show that we can sample a network with 75 million edges in 87 seconds on a single processor.
更多查看译文
关键词
complex networks,graph theory,sampling methods,Kronecker family models,Kronecker product graph model,complex networks,exact sampling,fractal structure,generative graph models,mKPGM,mixed KPGM,scalable sampling methods
AI 理解论文
溯源树
样例
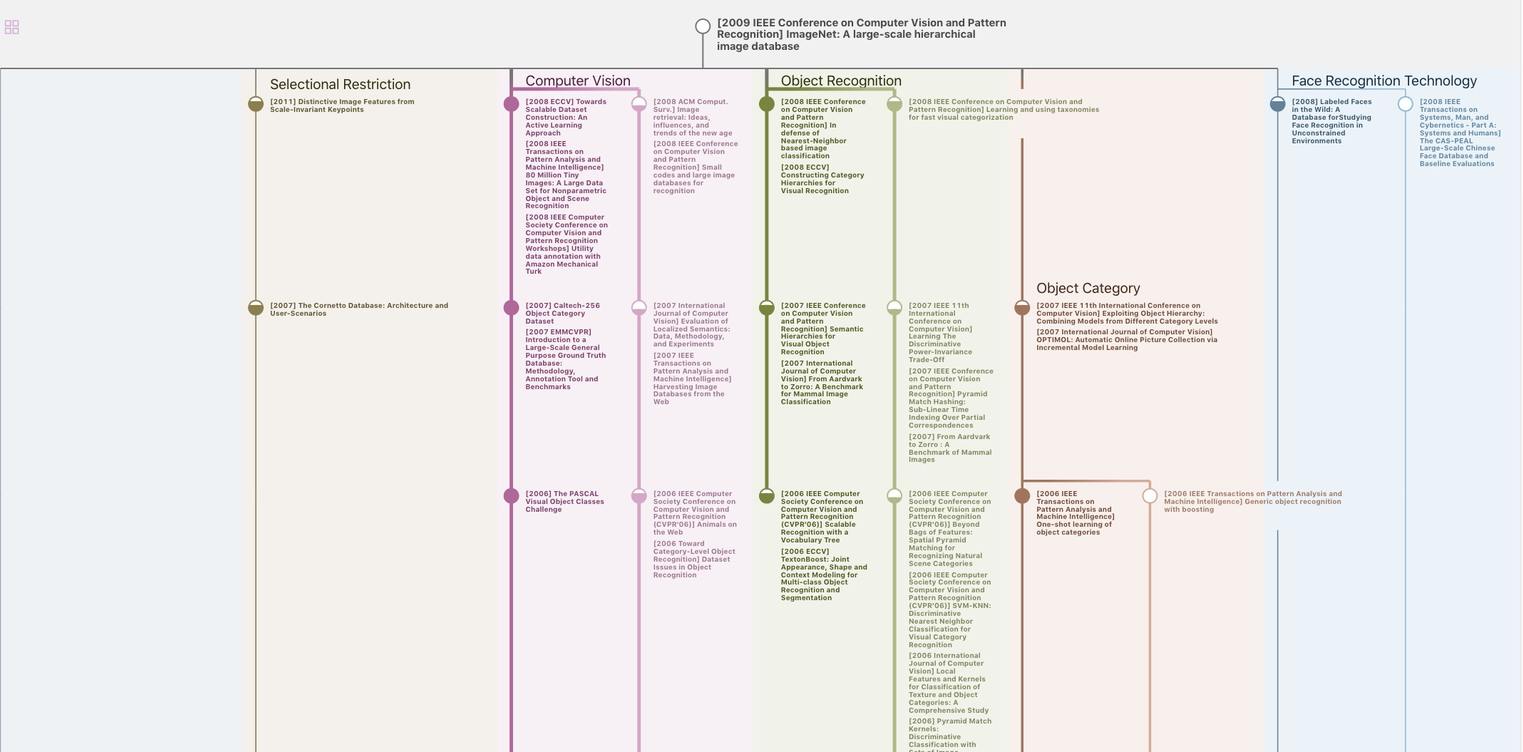
生成溯源树,研究论文发展脉络
Chat Paper
正在生成论文摘要