Topic Models with Topic Ordering Regularities for Topic Segmentation
Data Mining(2014)
摘要
Documents from the same domain usually discuss similar topics in a similar order. In this paper we present new ordering-based topic models that use generalised Mallows models to capture this regularity to constrain topic assignments. Specifically, these new models assume that there is a canonical topic ordering shared amongst documents from the same domain, and each document-specific topic ordering is allowed to vary from the canonical topic ordering. Instead of full orderings over a set of all possible topics covered by a domain, we make use of top-t orderings via a multistage ranking process. We show how to reformulate the new models so that a point-wise sampling algorithm from the Bayesian word segmentation literature can be used for posterior inference. Experimental results on several document collections with different properties show that our model performs much better than the other topic ordering-based models, and competitively with other state-of-the-art topic segmentation models.
更多查看译文
关键词
ordering-based topic models,belief networks,permutation,gmm,posterior inference,bayesian word segmentation literature,pattern classification,topic assignments,multistage ranking process,canonical topic ordering,generalised mallows models,document-specific topic ordering,top-t ordering,top-t orderings,topic model,topic segmentation,sampling methods,document handling,point-wise sampling algorithm,topic ordering regularities,internet,electronic publishing,encyclopedias,hidden markov models
AI 理解论文
溯源树
样例
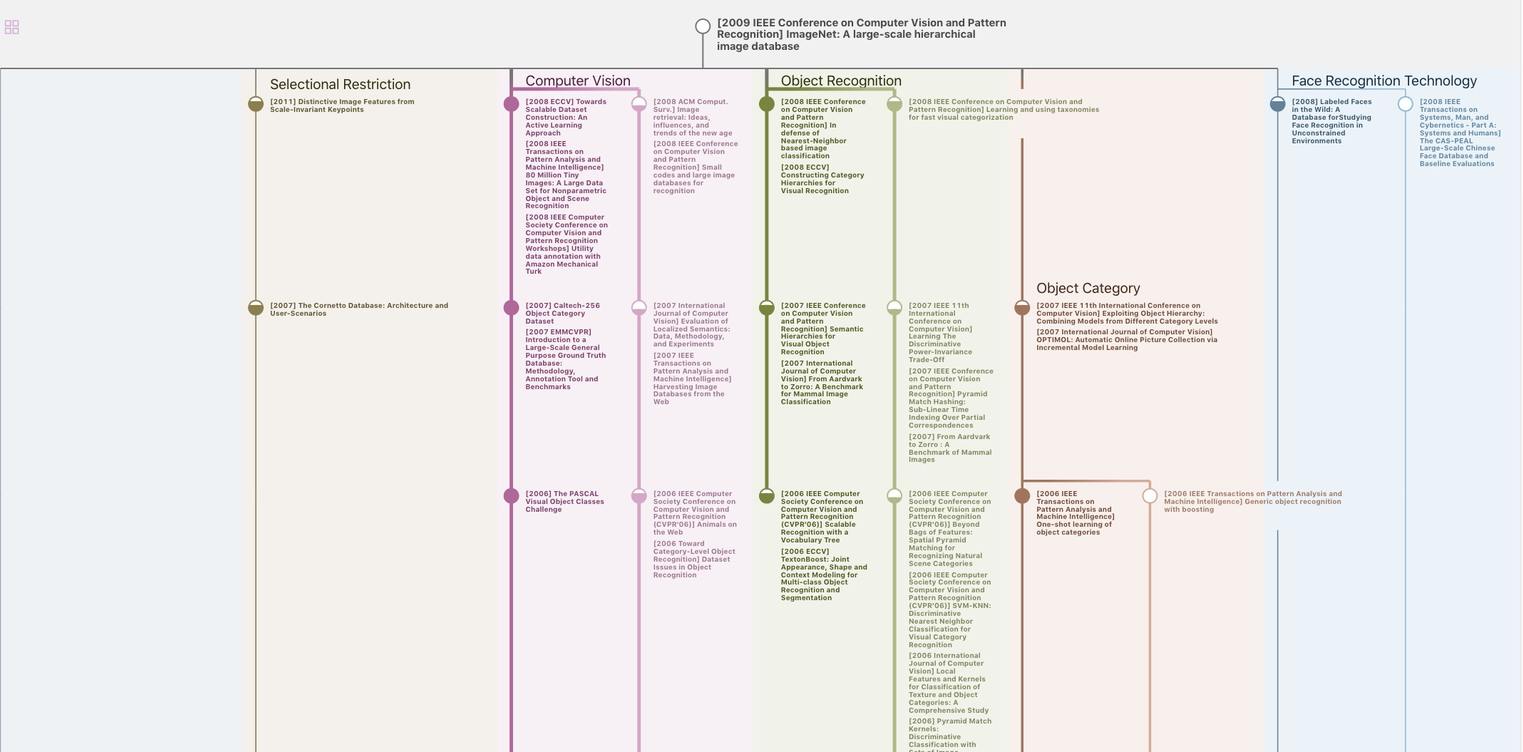
生成溯源树,研究论文发展脉络
Chat Paper
正在生成论文摘要