Multi-label Classification with Meta-Labels
Data Mining(2014)
摘要
The area of multi-label classification has rapidly developed in recent years. It has become widely known that the baseline binary relevance approach can easily be outperformed by methods which learn labels together. A number of methods have grown around the label power set approach, which models label combinations together as class values in a multi-class problem. We describe the label-power set-based solutions under a general framework of meta-labels and provide some theoretical justification for this framework which has been lacking, explaining how meta-labels essentially allow a random projection into a space where non-linearities can easily be tackled with established linear learning algorithms. The proposed framework enables comparison and combination of related approaches to different multi-label problems. We present a novel model in the framework and evaluate it empirically against several high-performing methods, with respect to predictive performance and scalability, on a number of datasets and evaluation metrics. This deployment obtains competitive accuracy for a fraction of the computation required by the current meta-label methods for multi-label classification.
更多查看译文
关键词
learning (artificial intelligence),pattern classification,baseline binary relevance approach,evaluation metrics,label combinations,label powerset approach,label-powerset-based solutions,linear learning algorithms,meta-labels,multilabel classification,classification,multi-label
AI 理解论文
溯源树
样例
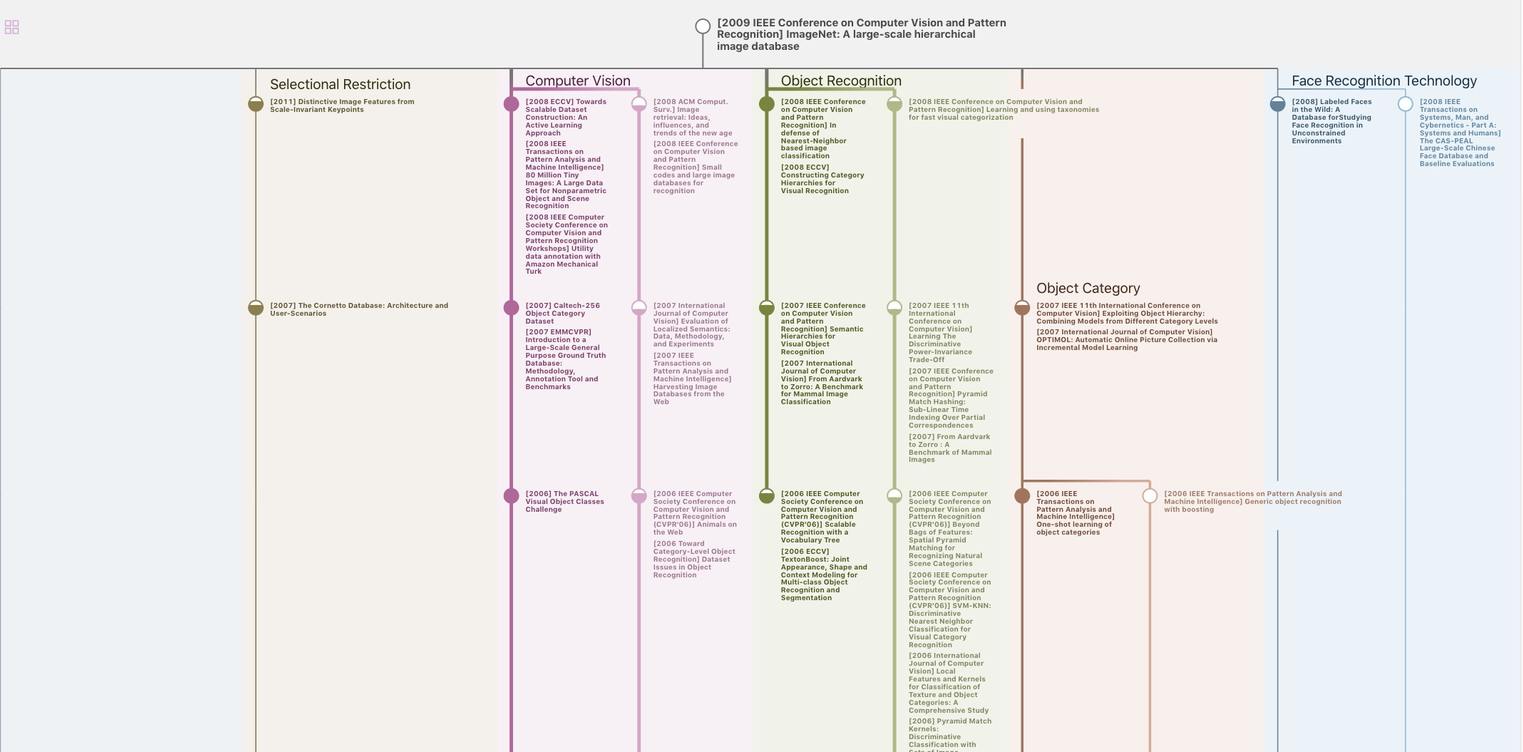
生成溯源树,研究论文发展脉络
Chat Paper
正在生成论文摘要