Physics-based enzyme design: predicting binding affinity and catalytic activity.
PROTEINS-STRUCTURE FUNCTION AND BIOINFORMATICS(2014)
摘要
Computational enzyme design is an emerging field that has yielded promising success stories, but where numerous challenges remain. Accurate methods to rapidly evaluate possible enzyme design variants could provide significant value when combined with experimental efforts by reducing the number of variants needed to be synthesized and speeding the time to reach the desired endpoint of the design. To that end, extending our computational methods to model the fundamental physical-chemical principles that regulate activity in a protocol that is automated and accessible to a broad population of enzyme design researchers is essential. Here, we apply a physics-based implicit solvent MM-GBSA scoring approach to enzyme design and benchmark the computational predictions against experimentally determined activities. Specifically, we evaluate the ability of MM-GBSA to predict changes in affinity for a steroid binder protein, catalytic turnover for a Kemp eliminase, and catalytic activity for -Gliadin peptidase variants. Using the enzyme design framework developed here, we accurately rank the most experimentally active enzyme variants, suggesting that this approach could provide enrichment of active variants in real-world enzyme design applications. Proteins 2014; 82:3397-3409. (c) 2014 Wiley Periodicals, Inc.
更多查看译文
关键词
computational enzyme design,structure-based design,binding optimization,transition state optimization,ranking,automated workflow,DIG-binder protein,Kemp eliminase (KE07),-gliadin peptidase
AI 理解论文
溯源树
样例
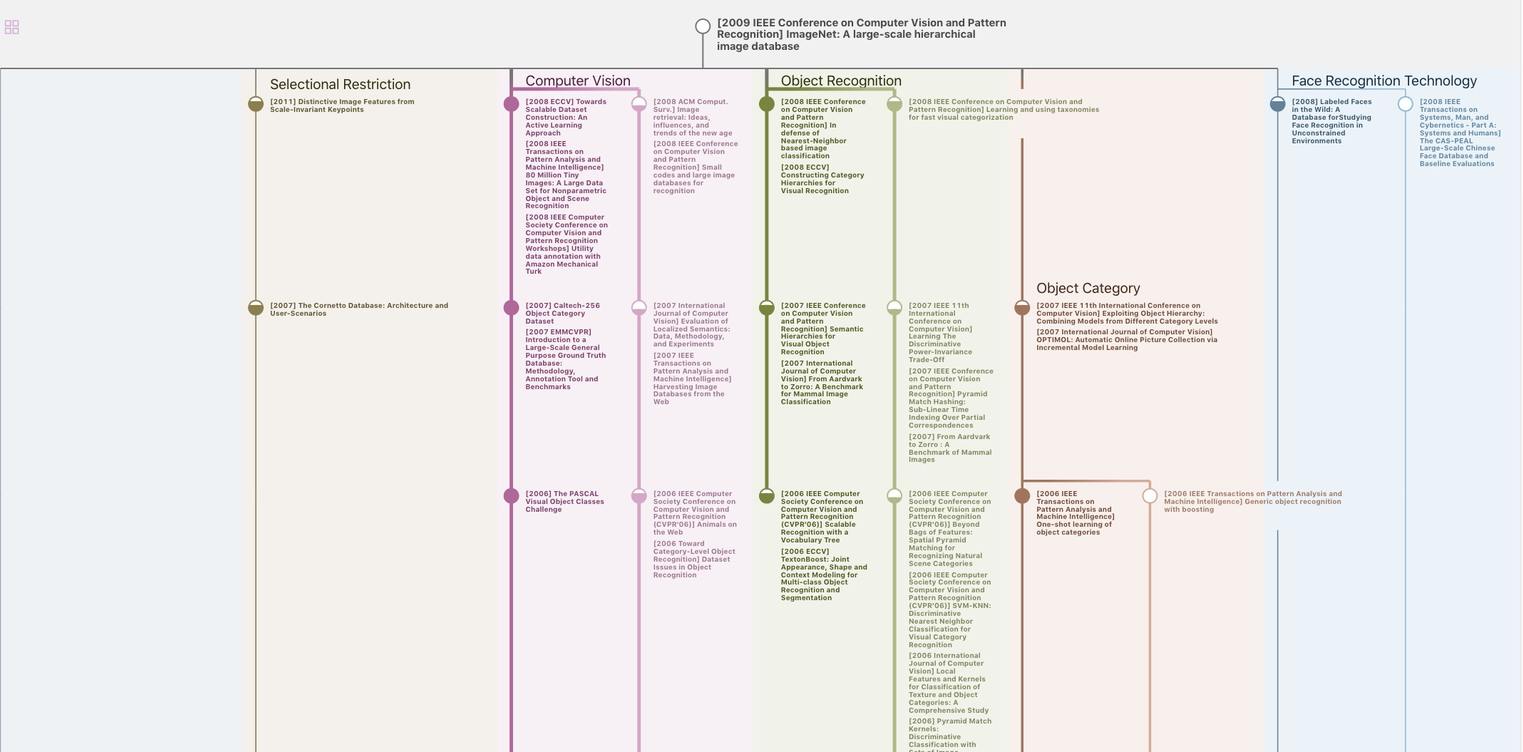
生成溯源树,研究论文发展脉络
Chat Paper
正在生成论文摘要