A document classifier for medicinal chemistry publications trained on the ChEMBL corpus
Journal of Cheminformatics(2014)
摘要
Background The large increase in the number of scientific publications has fuelled a need for semi- and fully automated text mining approaches in order to assist in the triage process, both for individual scientists and also for larger-scale data extraction and curation into public databases. Here, we introduce a document classifier, which is able to successfully distinguish between publications that are `ChEMBL-like’ (i.e. related to small molecule drug discovery and likely to contain quantitative bioactivity data) and those that are not. The unprecedented size of the medicinal chemistry literature collection, coupled with the advantage of manual curation and mapping to chemistry and biology make the ChEMBL corpus a unique resource for text mining. Results The method has been implemented as a data protocol/workflow for both Pipeline Pilot (version 8.5) and KNIME (version 2.9) respectively. Both workflows and models are freely available at: ftp://ftp.ebi.ac.uk/pub/databases/chembl/text-mining . These can be readily modified to include additional keyword constraints to further focus searches. Conclusions Large-scale machine learning document classification was shown to be very robust and flexible for this particular application, as illustrated in four distinct text-mining-based use cases. The models are readily available on two data workflow platforms, which we believe will allow the majority of the scientific community to apply them to their own data. Abstract
更多查看译文
关键词
Machine learning,Triage,Curation,Document classification
AI 理解论文
溯源树
样例
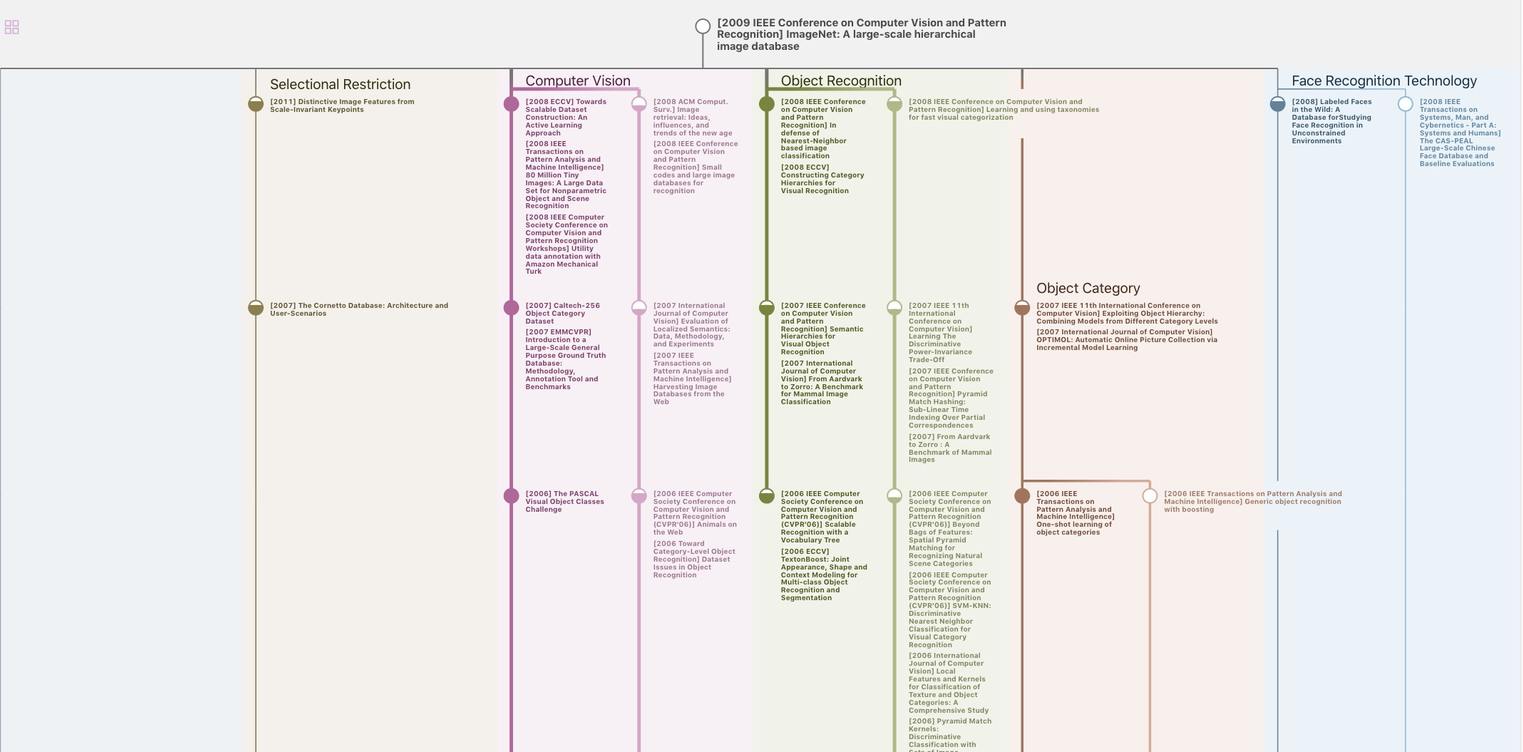
生成溯源树,研究论文发展脉络
Chat Paper
正在生成论文摘要