Prediction of Significant Vasospasm in Aneurysmal Subarachnoid Hemorrhage Using Automated Data
Neurocritical care(2014)
摘要
Background When vasospasm is detected after aneurysmal subarachnoid hemorrhage (aSAH), it is treated with hypertensive or endovascular therapy. Current classification methods are resource-intensive, relying on specialty-trained professionals (nursing exams, transcranial dopplers, and perfusion imaging). More passively obtained variables such as cerebrospinal fluid drainage volumes, sodium, glucose, blood pressure, intracranial pressure, and heart rate, have not been used to predict vasospasm. We hypothesize that these features may yield as much information as resource-intensive features to classify vasospasm. Methods We studied data from 81 aSAH patients presenting within two days of onset. Vasospasm class (VSP) was defined by angiographic vasospasm warranting endovascular treatment. Naïve Bayes (NB) and logistic regression (LR) classifiers were trained on selected variable feature sets from the first three days of illness. Performance of trained classifiers was evaluated using area under the receiver operator characteristic curve (AUC classifier) and F-measure (F classifier). Ablation analysis determined incremental utility of each variable and subsets. Results 43.2 % developed VSP. During feature selection, the only passively collected variable that did not yield a statistically significant summary statistic was CSF drainage volume. NB classifier trained on all passively obtained features (AUC NB 0.708 and F NB 0.636) outperformed NB classifier trained on resource-intensive features (AUC NB 0.501 and F NB 0.349). Conclusions Data-driven analysis of passively obtained clinical data predicted VSP better than current targeted resource-intensive monitoring techniques after aSAH. Automated classification of VSP may be possible.
更多查看译文
关键词
Clinical decision making,Machine learning,Subarachnoid hemorrhage,Vasospasm detection
AI 理解论文
溯源树
样例
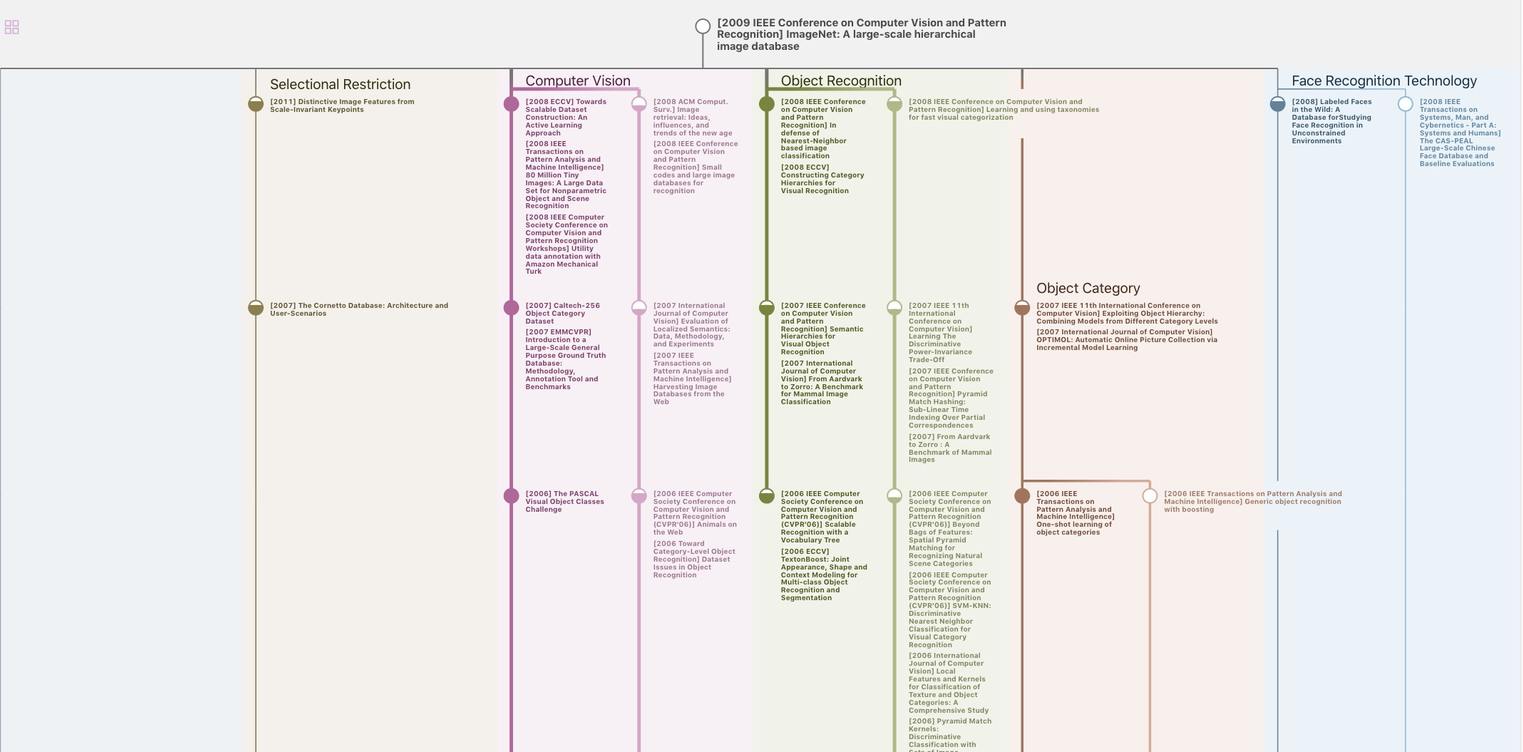
生成溯源树,研究论文发展脉络
Chat Paper
正在生成论文摘要