Detection of and Compensation for EMG Disturbances for Powered Lower Limb Prosthesis Control
IEEE transactions on neural systems and rehabilitation engineering : a publication of the IEEE Engineering in Medicine and Biology Society(2015)
摘要
Myoelectric pattern recognition algorithms have been proposed for the control of powered lower limb prostheses, but electromyography (EMG) signal disturbances remain an obstacle to clinical implementation. To address this problem, we investigated using a log likelihood metric to detect both simulated EMG disturbances and real disturbances acquired from EMG containing electrode shift. We found that features extracted from disturbed EMG signals have much lower log likelihoods than those from undisturbed signals and can be detected using a single threshold acquired from the training data. We designed a linear discriminant analysis (LDA) classifier that uses the log likelihood to decide between using a combination of EMG and mechanical sensors or using mechanical sensors only, to predict locomotion modes. When EMG signals contained disturbances, our classifier was able to detect those disturbances and disregard EMG data. Consequently our classifier had significantly lower prediction errors than a standard LDA classifier in the presence of EMG disturbances. The log likelihood classifier had a low false positive threshold, and thus did not perform significantly differently from the standard LDA classifier when EMG signals did not contain disturbances. The log likelihood threshold could also be applied to individual EMG channels, enabling specific channels containing EMG disturbances to be appropriately ignored when making locomotion mode predictions.
更多查看译文
关键词
adaptive algorithms,electromyography (emg),neural engineering,pattern recognition,powered prostheses
AI 理解论文
溯源树
样例
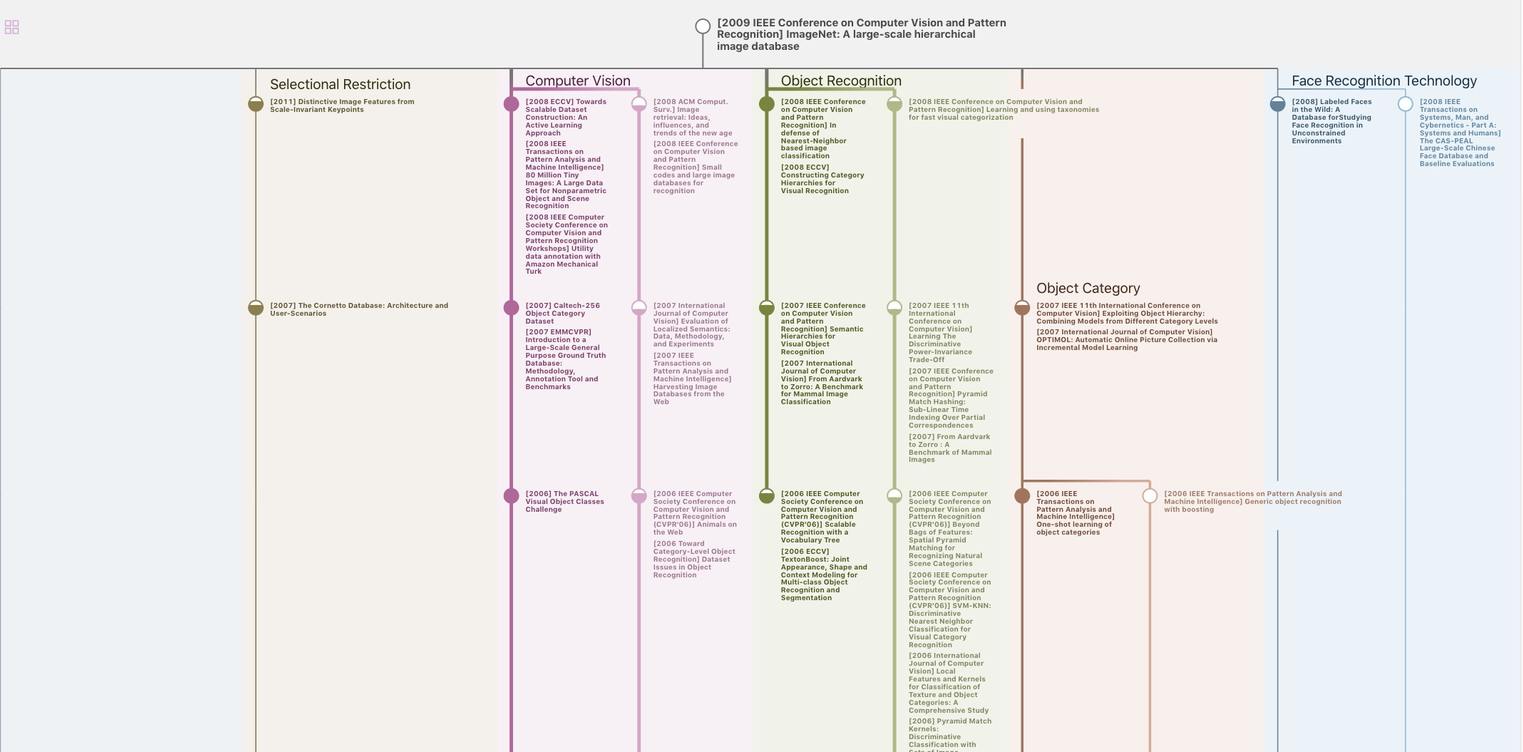
生成溯源树,研究论文发展脉络
Chat Paper
正在生成论文摘要