Nonnegative matrix factorization for the identification of EMG finger movements: evaluation using matrix analysis.
IEEE journal of biomedical and health informatics(2015)
摘要
Surface electromyography (sEMG) is widely used in evaluating the functional status of the hand to assist in hand gesture recognition, prosthetics and rehabilitation applications. The sEMG is a noninvasive, easy to record signal of superficial muscles from the skin surface. Considering the nonstationary characteristics of sEMG, recent feature selection of hand gesture recognition using sEMG signals necessitate designers to use nonnegative matrix factorization (NMF)-based methods. This method exploits both the additive and sparse nature of signals by extracting accurate and reliable measurements of sEMG features using a minimum number of sensors. The testing has been conducted for simple and complex finger flexions using several experiments with artificial neural network classification scheme. It is shown, both by simulation and experimental studies, that the proposed algorithm is able to classify ten finger flexions (five simple and five complex finger flexions) recorded from two sEMG sensors up to 92% (95% for simple and 87% for complex flexions) accuracy. The recognition performances of simple and complex finger flexions are also validated with NMF permutation matrix analysis.
更多查看译文
关键词
sparse signal exploitation,biomechanics,artificial neural network classification scheme,functional hand status evaluation,emg finger movement identification,matrix analysis-based finger movement analysis,medical signal detection,nmf permutation matrix analysis,principal component analysis (pca),rehabilitation applications,artificial neural network (ann),semg sensor-recorded simple finger flexion,additive signal exploitation,patient monitoring,complex finger flexion recognition performance,semg sensor-recorded complex finger flexion,prosthetics,hand gesture recognition,medical signal processing,image sensors,sensor-based semg feature extraction,surface electromyography feature extraction,feature extraction,skin surface muscular signal,nonnegative matrix factorization,patient care,nonnegative matrix factorization (nmf),matrix decomposition,accurate semg feature extraction,reliable semg feature measurements,noninvasive muscular signal recording tool,algorithm-based finger flexion classification,superficial muscle signal,electromyography-based finger movement identification,root mean square (rms),electromyography,sensor based semg feature measurement,nonstationary semg characteristics,gesture recognition,simple finger flexion recognition performance,feature selection,flexions,gestures,bioinformatics,nmf-based methods,surface electromyography (semg),auto regression (ar),skin,semg signal-based hand gesture recognition,patient diagnosis,neural nets,skin surface muscle signal,artificial neural networks
AI 理解论文
溯源树
样例
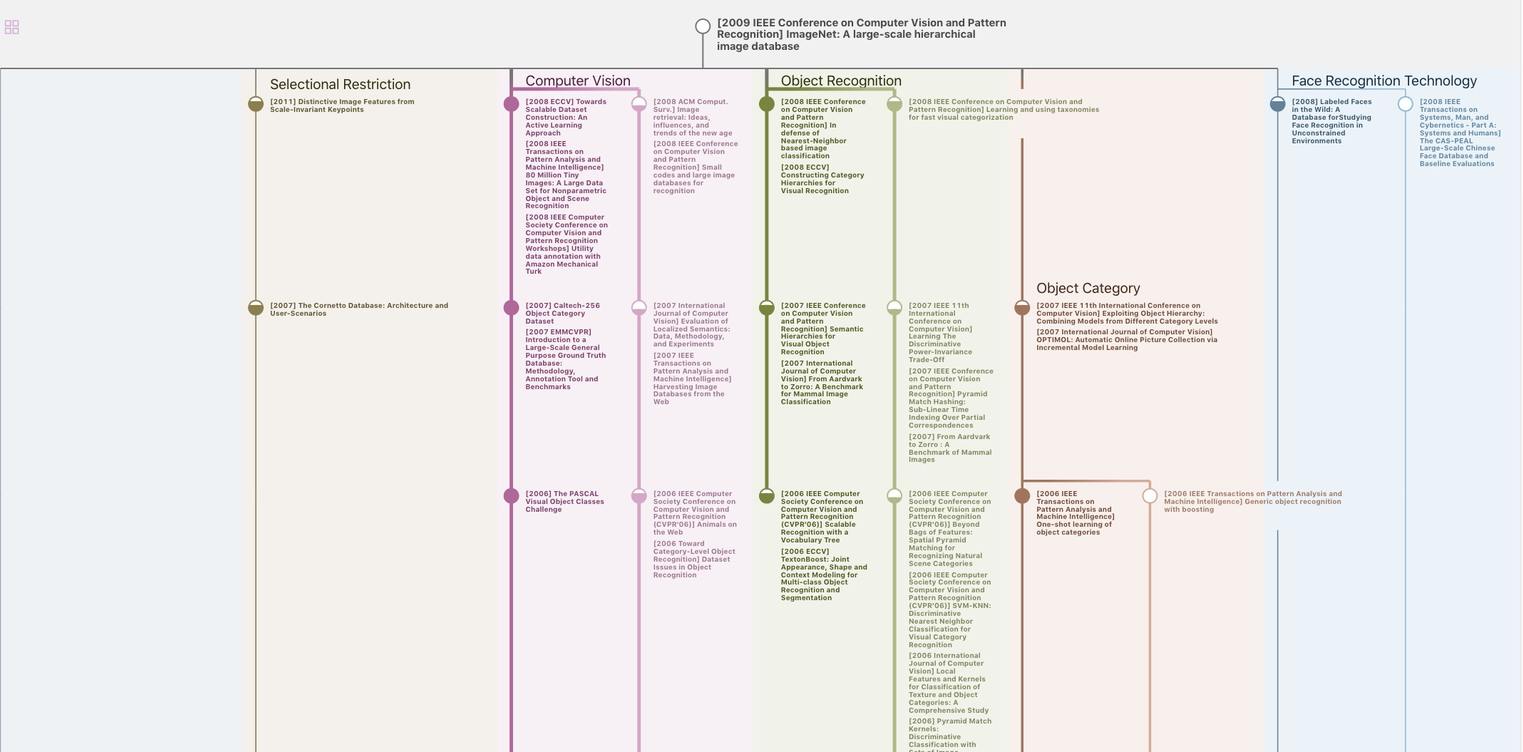
生成溯源树,研究论文发展脉络
Chat Paper
正在生成论文摘要