General nonunitary constrained ICA and its application to complex-valued fMRI data.
IEEE transactions on bio-medical engineering(2015)
摘要
Constrained independent component analysis (C-ICA) algorithms provide an effective way to introduce prior information into the complex- and real-valued ICA framework. The work in this area has focus on adding constraints to the objective function of algorithms that assume a unitary demixing matrix. The unitary condition is required in order to decouple-isolate-the constraints applied for each individual source. This assumption limits the optimization space and, therefore, the separation performance of C-ICA algorithms. We generalize the existing C-ICA framework by using a novel decoupling method that preserves the larger optimization space for the demixing matrix. This framework allows for the constraining of either the sources or the mixing coefficients. A constrained version of the nonunitary entropy bound minimization algorithm is introduced and applied to actual complex-valued fMRI data. We show that constraining the mixing parameters using a temporal constraint improves the estimation of the spatial map and timecourses of task-related components.
更多查看译文
关键词
mutual information (mi),mixing coefficients,c-ica algorithms,optimization space,matrix algebra,nonunitary entropy bound minimization algorithm,independent component analysis,nonunitary constrained ica,biomedical mri,decoupling method,unitary demixing matrix,complex-valued fmri data,constrained ica,decoupled,mutual information,minimisation,entropy bound,medical image processing,algorithm design and analysis,measurement,optimization,biomedical engineering,vectors,entropy,linear programming
AI 理解论文
溯源树
样例
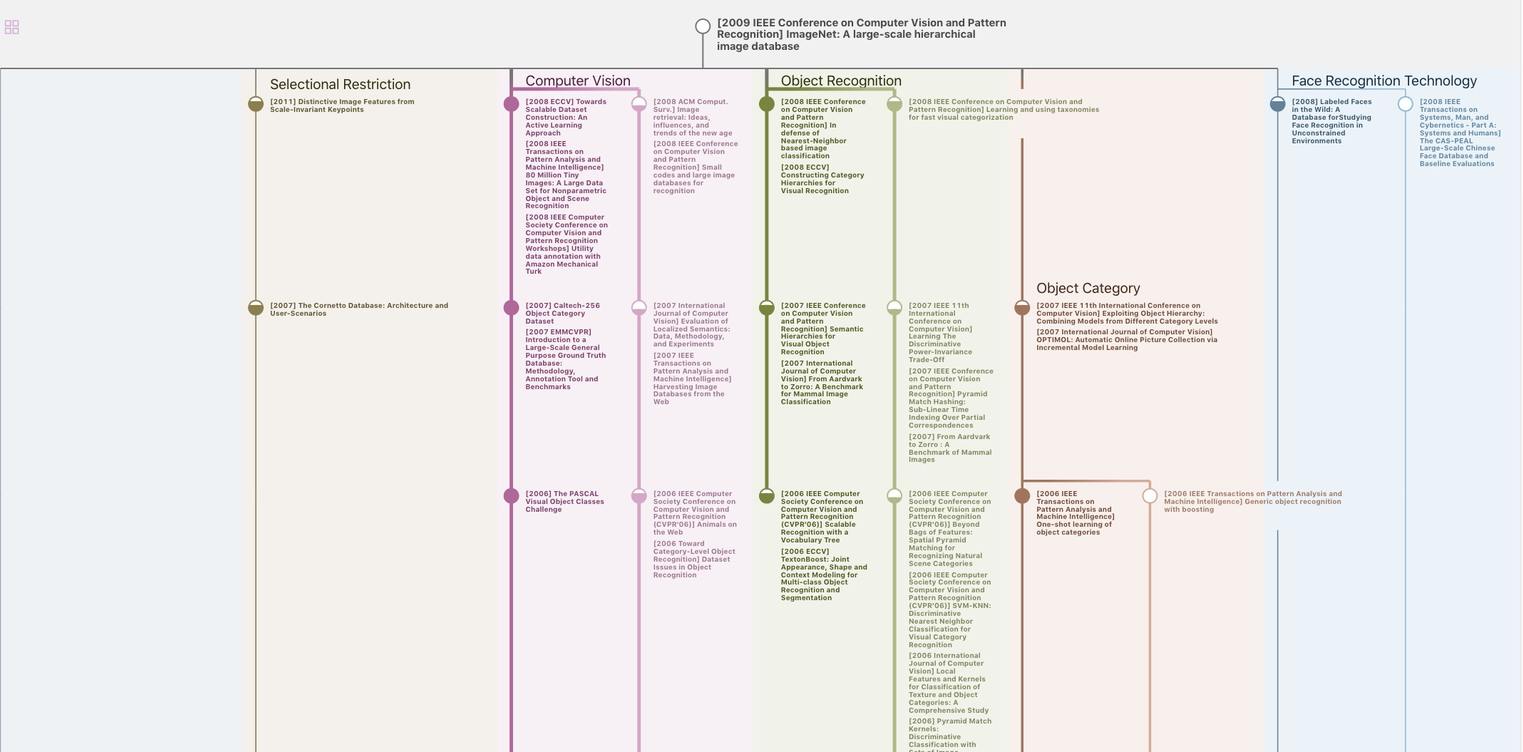
生成溯源树,研究论文发展脉络
Chat Paper
正在生成论文摘要