Linear-Time Subspace Clustering via Bipartite Graph Modeling.
IEEE transactions on neural networks and learning systems(2015)
摘要
We present a linear-time subspace clustering approach that combines sparse representations and bipartite graph modeling. The signals are modeled as drawn from a union of low-dimensional subspaces, and each signal is represented by a sparse combination of basis elements, termed atoms, which form the columns of a dictionary matrix. The sparse representation coefficients are arranged in a sparse affinity matrix, which defines a bipartite graph of two disjoint sets: 1) atoms and 2) signals. Subspace clustering is obtained by applying low-complexity spectral bipartite graph clustering that exploits the small number of atoms for complexity reduction. The complexity of the proposed approach is linear in the number of signals, thus it can rapidly cluster very large data collections. Performance evaluation of face clustering and temporal video segmentation demonstrates comparable clustering accuracies to state-of-the-art at a significantly lower computational load.
更多查看译文
关键词
bipartite graph,face clustering,dictionary,temporal video segmentation.,subspace clustering,sparse representation,optimization,dictionaries,matrix decomposition,clustering algorithms,sparse matrices
AI 理解论文
溯源树
样例
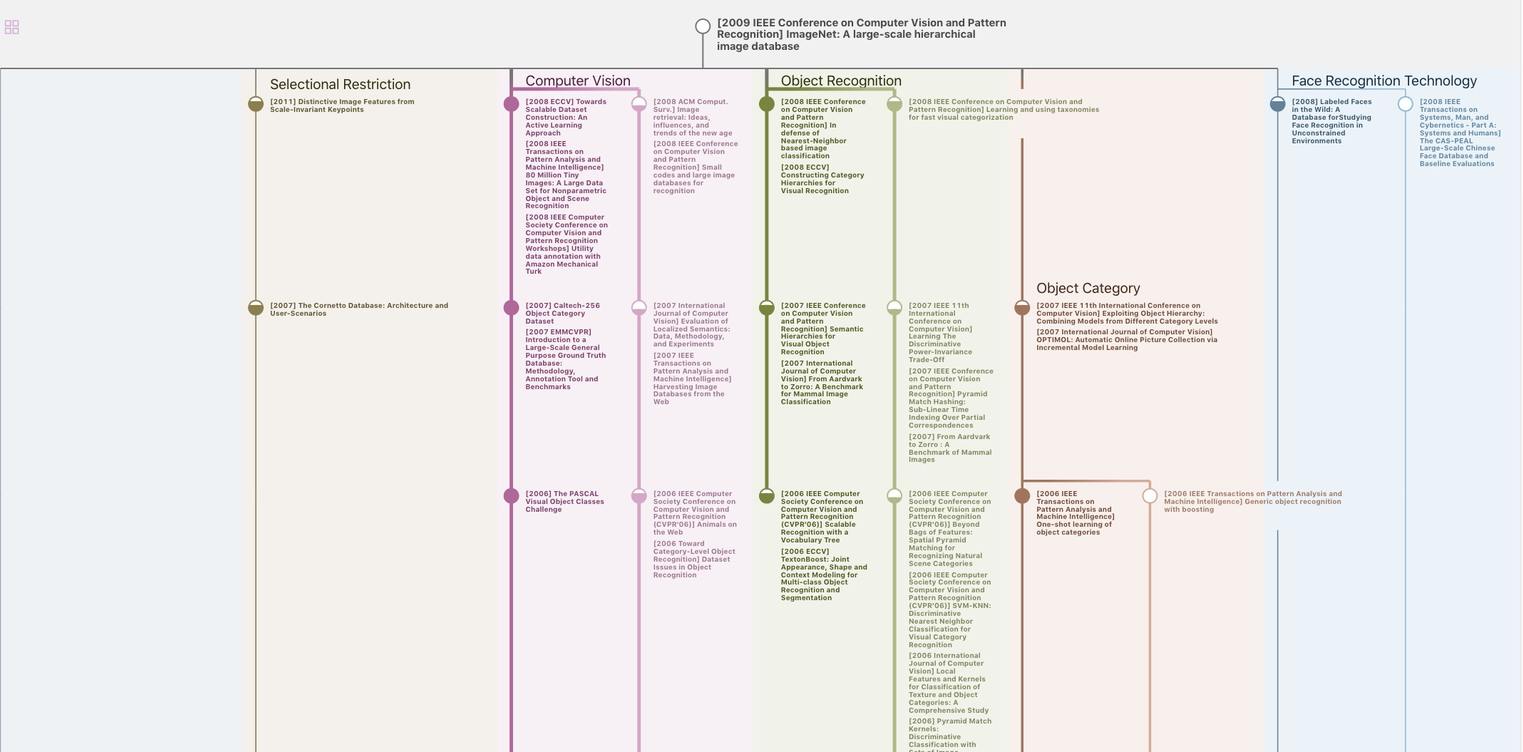
生成溯源树,研究论文发展脉络
Chat Paper
正在生成论文摘要