Multi-aspect heterogeneous information network for MOOC knowledge concept recommendation
Applied Intelligence(2022)
摘要
Recently, the Massive Open Online Course (MOOC) learning system has become a popular education platform, and the information overload issue within it is becoming much more serious, due to the increasing of online education needs. Consequently, the studies that focus on recommending learning resources (e.g., courses and knowledge concepts) have become a hot research topic. Moreover, as courses are composed of sets of knowledge concepts, directly recommending courses will ignore the students’ fine grained learning state. In this work, we focus on the Knowledge Concept Recommendation (KCR) task that aims at exploring the concepts that a student needs to master. Existing methods on KCR are mainly well designed graph convolutional models that pay more attention to exploring students’ preference similarity. However, most of these methods only have limited representation ability, as they mainly rely on a single type of nodes, and the diversified relationships among students and concepts are not fully explored. In light of this, we propose a Multi-aspect Heterogeneous Information Network (Multi-HIN) for KCR. Specifically, to consider the impact of multi-types of entities on preference learning, we first construct a Heterogeneous Information Network (HIN) to link concepts and multiple entities in a network. Then, to learn a more accurate node representation, we dynamically assign an aspect context to each node by viewing aspects as students’ interest dimensions. To learn items’ representations based on the current aspect, we further conduct the aspect selection process via the Gumbel–Softmax method. Finally, we use an extended Matrix Factorization (MF) method to make concept recommendations. We conduct extensive experiments on a real-world dataset to demonstrate the superiority of our proposed method.
更多查看译文
关键词
Knowledge concept recommendation, Representation learning, Heterogeneous information network
AI 理解论文
溯源树
样例
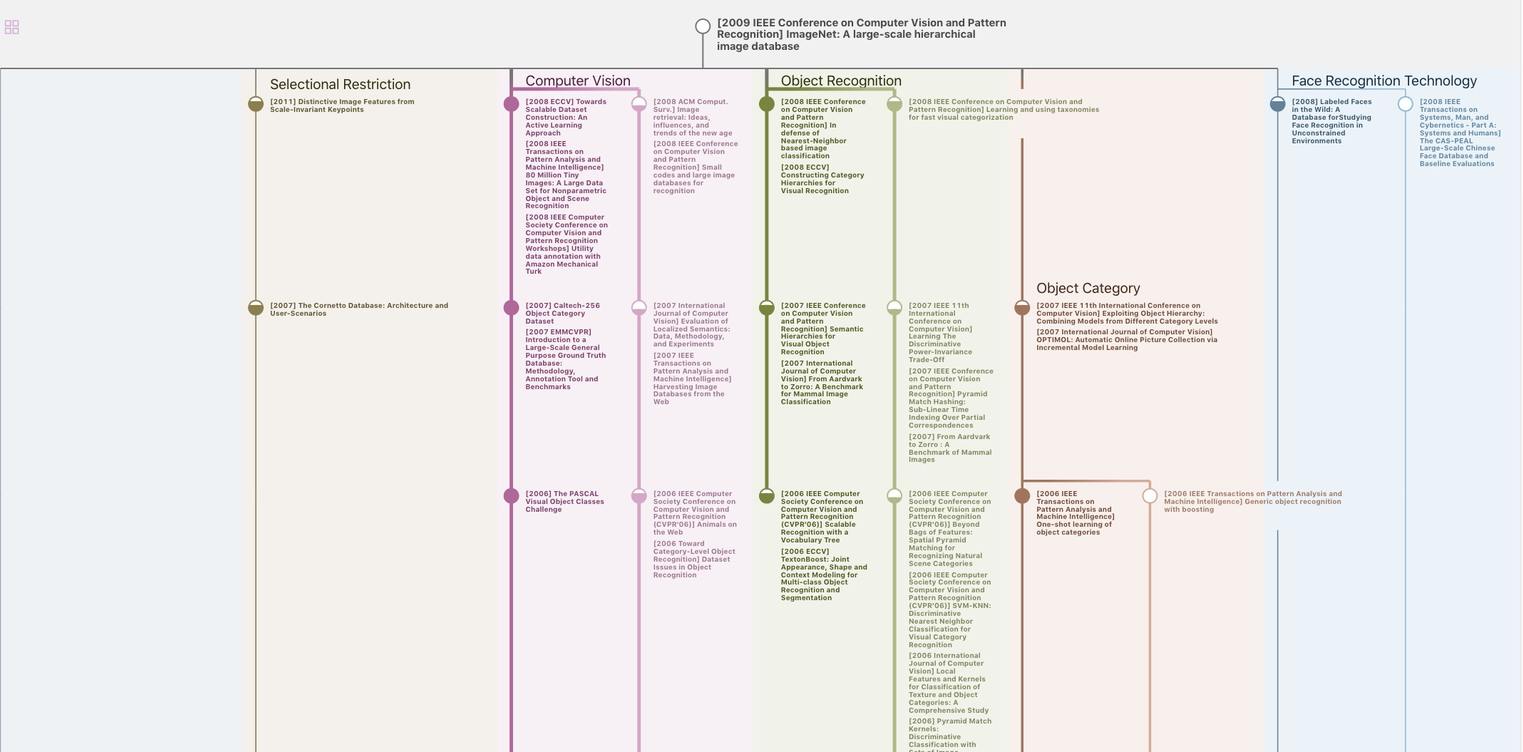
生成溯源树,研究论文发展脉络
Chat Paper
正在生成论文摘要