Semi-supervised multi-label collective classification ensemble for functional genomics
BMC GENOMICS(2014)
摘要
Background With the rapid accumulation of proteomic and genomic datasets in terms of genome-scale features and interaction networks through high-throughput experimental techniques, the process of manual predicting functional properties of the proteins has become increasingly cumbersome, and computational methods to automate this annotation task are urgently needed. Most of the approaches in predicting functional properties of proteins require to either identify a reliable set of labeled proteins with similar attribute features to unannotated proteins, or to learn from a fully-labeled protein interaction network with a large amount of labeled data. However, acquiring such labels can be very difficult in practice, especially for multi-label protein function prediction problems. Learning with only a few labeled data can lead to poor performance as limited supervision knowledge can be obtained from similar proteins or from connections between them. To effectively annotate proteins even in the paucity of labeled data, it is important to take advantage of all data sources that are available in this problem setting, including interaction networks, attribute feature information, correlations of functional labels, and unlabeled data. Results In this paper, we show that the underlying nature of predicting functional properties of proteins using various data sources of relational data is a typical collective classification (CC) problem in machine learning. The protein functional prediction task with limited annotation is then cast into a semi-supervised multi-label collective classification (SMCC) framework. As such, we propose a novel generative model based SMCC algorithm, called GM-SMCC, to effectively compute the label probability distributions of unannotated protein instances and predict their functional properties. To further boost the predicting performance, we extend the method in an ensemble manner, called EGM-SMCC, by utilizing multiple heterogeneous networks with various latent linkages constructed to explicitly model the relationships among the nodes for effectively propagate the supervision knowledge from labeled to unlabeled nodes. Conclusion Experimental results on a yeast gene dataset predicting the functions and localization of proteins demonstrate the effectiveness of the proposed method. In the comparison, we find that the performances of the proposed algorithms are better than the other compared algorithms.
更多查看译文
关键词
Protein function prediction, protein interaction networks, collective classification, semi-supervised learning, multi-label learning
AI 理解论文
溯源树
样例
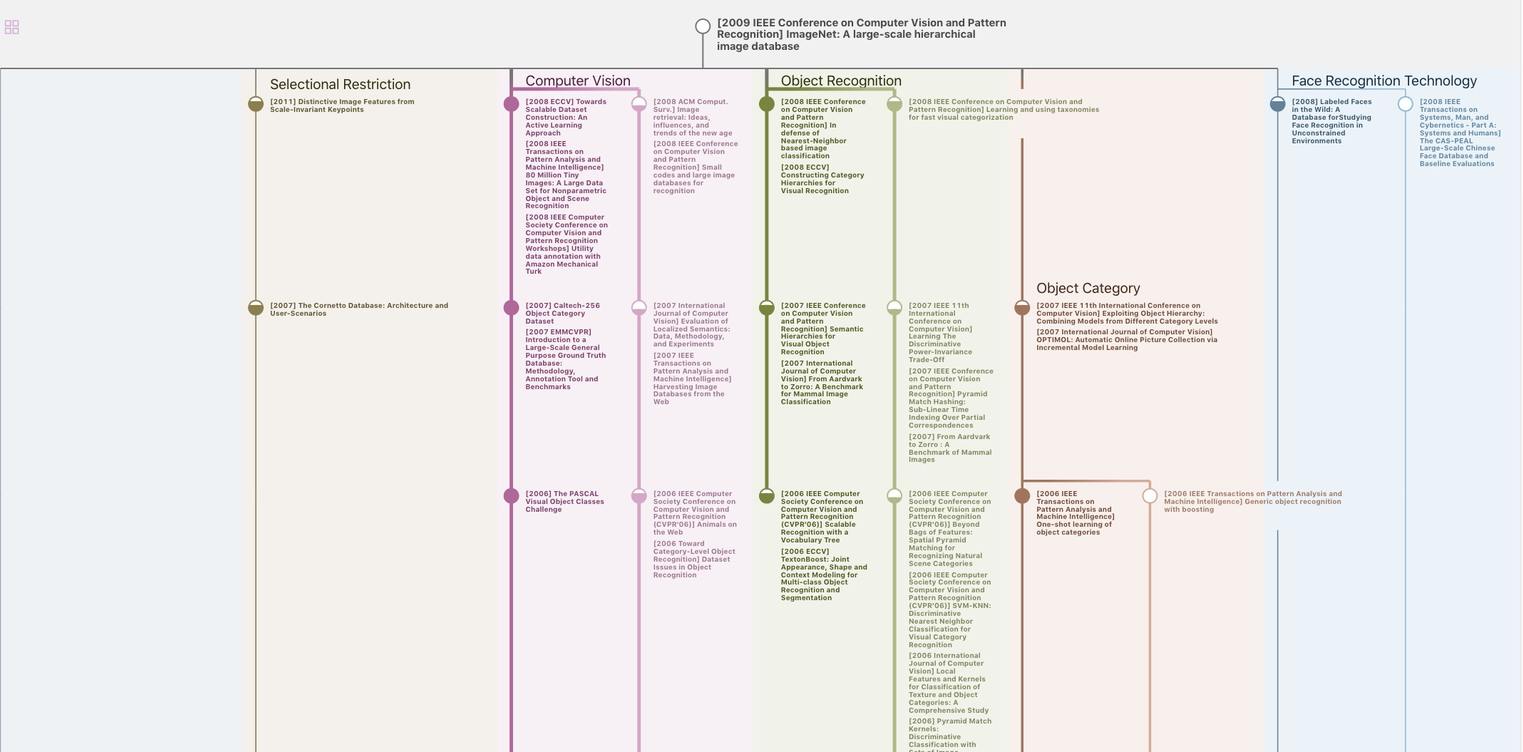
生成溯源树,研究论文发展脉络
Chat Paper
正在生成论文摘要