Sensory Prediction or Motor Control? Application of Marr-Albus Type Models of Cerebellar Function to Classical Conditioning.
FRONTIERS IN COMPUTATIONAL NEUROSCIENCE(2010)
摘要
Marr-Albus adaptive filter models of the cerebellum have been applied successfully to a range of sensory and motor control problems. Here we analyze their properties when applied to classical conditioning of the nictitating membrane response in rabbits. We consider a system-level model of eyeblink conditioning based on the anatomy of the eyeblink circuitry, comprising an adaptive filter model of the cerebellum, a comparator model of the inferior olive and a linear dynamic model of the nictitating membrane plant. To our knowledge, this is the first model that explicitly includes all these principal components, in particular the motor plant that is vital for shaping and timing the behavioral response. Model assumptions and parameters were systematically investigated to disambiguate basic computational capacities of the model from features requiring tuning of properties and parameter values. Without such tuning, the model robustly reproduced a range of behaviors related to sensory prediction, by displaying appropriate trial-level associative learning effects for both single and multiple stimuli, including blocking and conditioned inhibition. In contrast, successful reproduction of the real-time motor behavior depended on appropriate specification of the plant, cerebellum and comparator models. Although some of these properties appear consistent with the system biology, fundamental questions remain about how the biological parameters are chosen if the cerebellar microcircuit applies a common computation to many distinct behavioral tasks. It is possible that the response profiles in classical conditioning of the eyeblink depend upon operant contingencies that have previously prevailed, for example in naturally occurring avoidance movements.
更多查看译文
关键词
classical conditioning,cerebellum,eyeblink conditioning,adaptive filter,Marr-Albus,motor learning,inferior olive
AI 理解论文
溯源树
样例
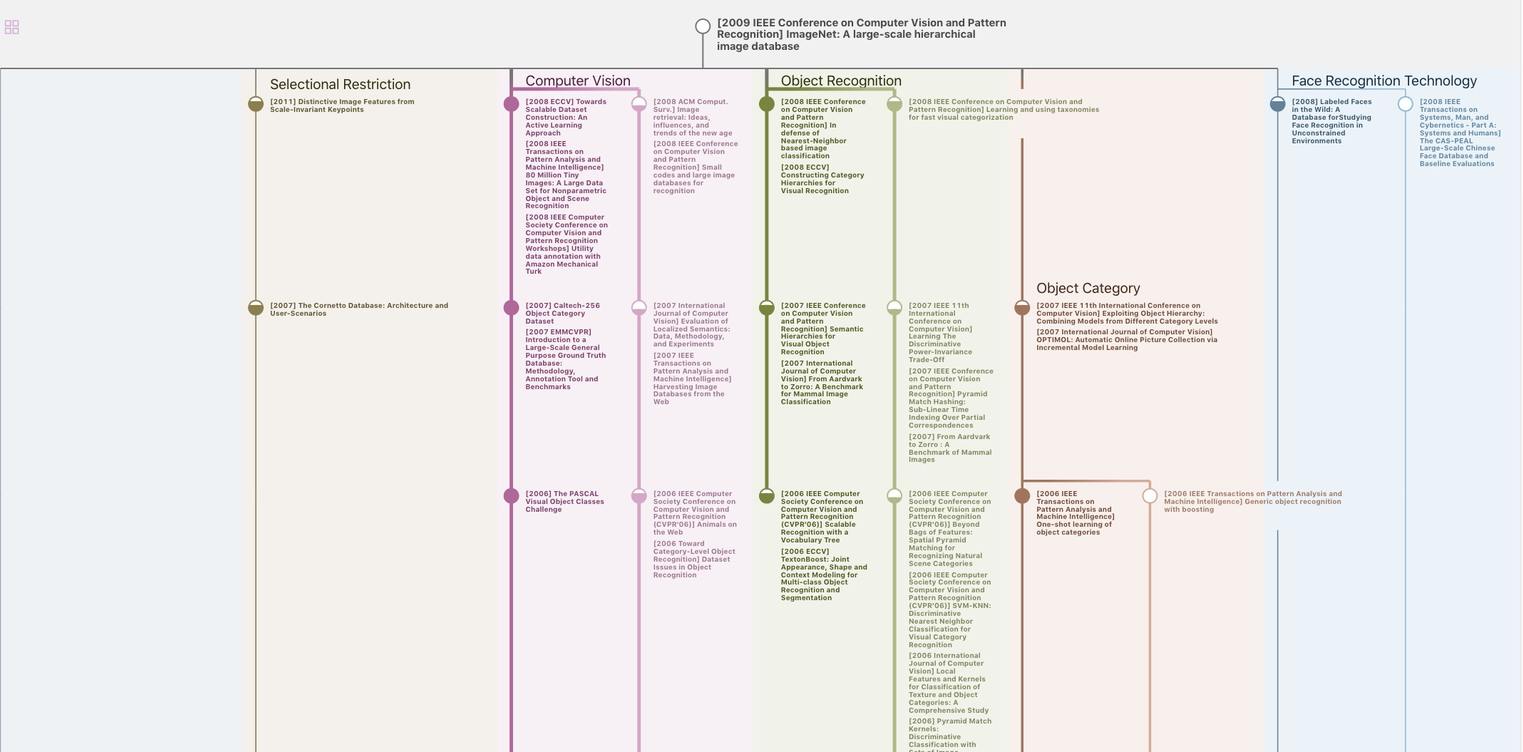
生成溯源树,研究论文发展脉络
Chat Paper
正在生成论文摘要