Learning to track multiple targets.
IEEE Trans. Neural Netw. Learning Syst.(2015)
摘要
Monocular multiple-object tracking is a fundamental yet under-addressed computer vision problem. In this paper, we propose a novel learning framework for tracking multiple objects by detection. First, instead of heuristically defining a tracking algorithm, we learn that a discriminative structure prediction model from labeled video data captures the interdependence of multiple influence factors. Given the joint targets state from the last time step and the observation at the current frame, the joint targets state at the current time step can then be inferred by maximizing the joint probability score. Second, our detection results benefit from tracking cues. The traditional detection algorithms need a nonmaximal suppression postprocessing to select a subset from the total detection responses as the final output and a large number of selection mistakes are induced, especially under a congested circumstance. Our method integrates both detection and tracking cues. This integration helps to decrease the postprocessing mistake risk and to improve performance in tracking. Finally, we formulate the entire model training into a convex optimization problem and estimate its parameters using the cutting plane optimization. Experiments show that our method performs effectively in a large variety of scenarios, including pedestrian tracking in crowd scenes and vehicle tracking in congested traffic.
更多查看译文
关键词
parameter estimation,discriminative model,prediction algorithms,detectors,computer vision,probability,vehicle tracking,cutting plane,interdependence,convex programming,trajectory,learning artificial intelligence
AI 理解论文
溯源树
样例
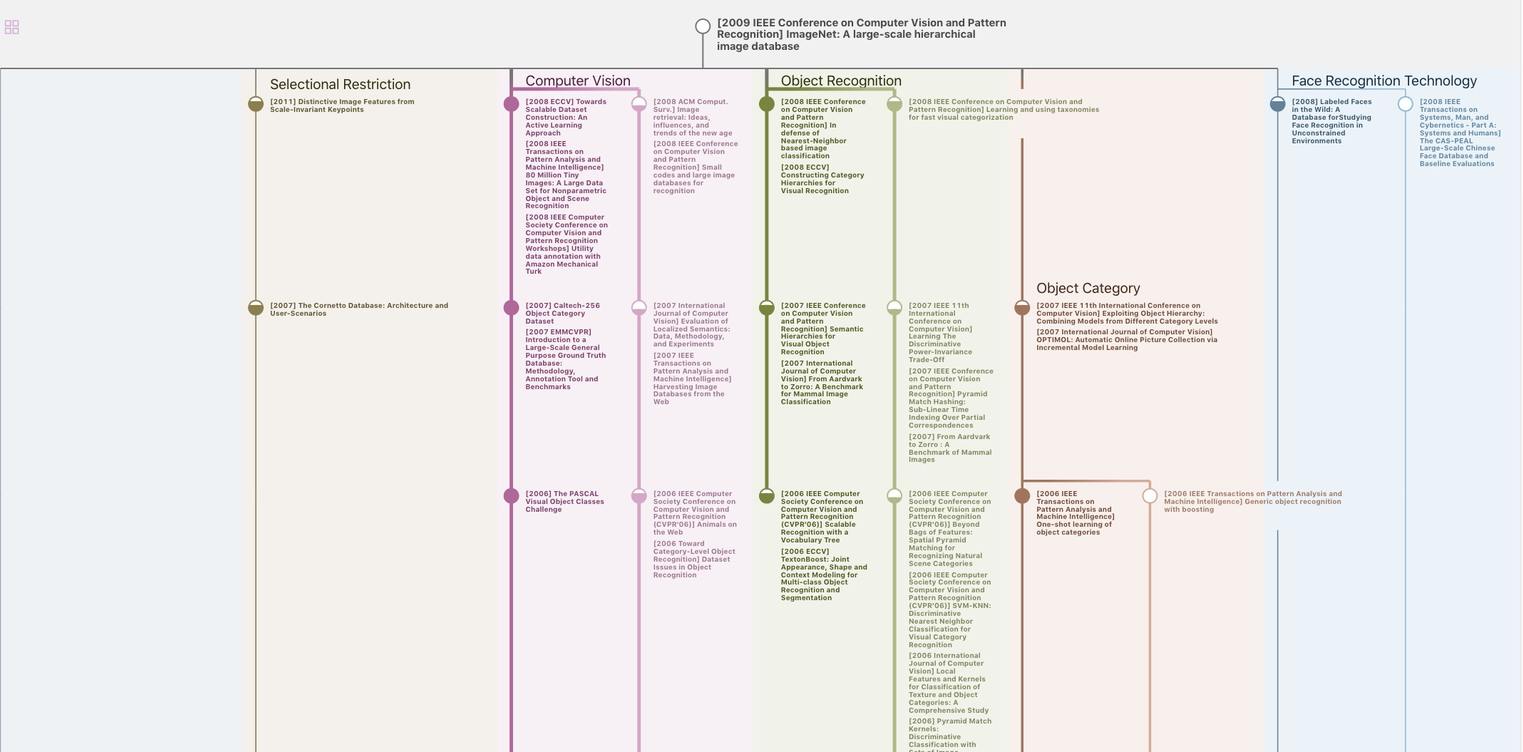
生成溯源树,研究论文发展脉络
Chat Paper
正在生成论文摘要