Joint mixing vector and binaural model based stereo source separation
IEEE/ACM Transactions on Audio, Speech & Language Processing(2014)
摘要
In this paper the mixing vector (MV) in the statistical mixing model is compared to the binaural cues represented by interaural level and phase differences (ILD and IPD). It is shown that the MV distributions are quite distinct while binaural models overlap when the sources are close to each other. On the other hand, the binaural cues are more robust to high reverberation than MV models. According to this complementary behavior we introduce a new robust algorithm for stereo speech separation which considers both additive and convolutive noise signals to model the MV and binaural cues in parallel and estimate probabilistic time-frequency masks. The contribution of each cue to the final decision is also adjusted by weighting the log-likelihoods of the cues empirically. Furthermore, the permutation problem of the frequency domain blind source separation (BSS) is addressed by initializing the MVs based on binaural cues. Experiments are performed systematically on determined and underdetermined speech mixtures in five rooms with various acoustic properties including anechoic, highly reverberant, and spatially-diffuse noise conditions. The results in terms of signal-to-distortion-ratio (SDR) confirm the benefits of integrating the MV and binaural cues, as compared with two state-of-the-art baseline algorithms which only use MV or the binaural cues.
更多查看译文
关键词
interaural level and phase differences,frequency domain blind source separation,statistical distributions,algorithms,design,spatially-diffuse noise condition,experimentation,speech recognition,joint mixing vector,ipd,time-frequency masking,acoustic properties,sound and music computing,reverberation,signal-to-distortion-ratio,speech mixtures,bss,anechoic properties,measurement,languages,binaural cues,blind source separation,probabilistic time-frequency masks,computational auditory scene analysis,convolutive noise signals,mv distribution,sdr,statistical mixing model,natural language processing,additive noise signals,reverberant properties,stereo speech separation,ild,performance,binaural model based stereo source separation,numerical algorithms and problems,time-frequency analysis
AI 理解论文
溯源树
样例
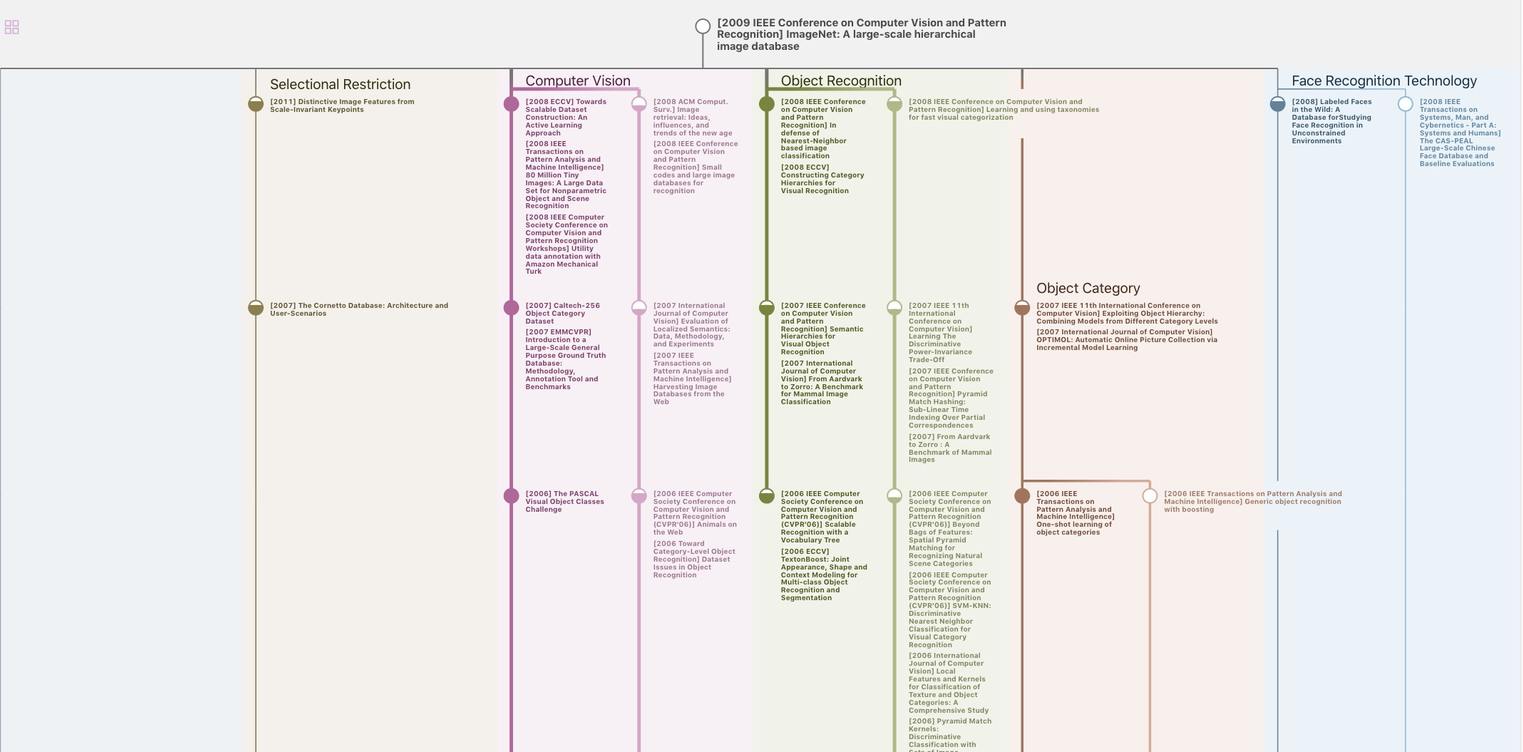
生成溯源树,研究论文发展脉络
Chat Paper
正在生成论文摘要