Background subtraction: separating the modeling and the inference
arXiv (Cornell University)(2013)
摘要
In its early implementations, background modeling was a process of building a model for the background of a video with a stationary camera, and identifying pixels that did not conform well to this model. The pixels that were not well-described by the background model were assumed to be moving objects. Many systems today maintain models for the foreground as well as the background, and these models compete to explain the pixels in a video. If the foreground model explains the pixels better, they are considered foreground. Otherwise they are considered background. In this paper, we argue that the logical endpoint of this evolution is to simply use Bayes’ rule to classify pixels. In particular, it is essential to have a background likelihood, a foreground likelihood, and a prior at each pixel. A simple application of Bayes’ rule then gives a posterior probability over the label. The only remaining question is the quality of the component models: the background likelihood, the foreground likelihood, and the prior. We describe a model for the likelihoods that is built by using not only the past observations at a given pixel location, but by also including observations in a spatial neighborhood around the location. This enables us to model the influence between neighboring pixels and is an improvement over earlier pixelwise models that do not allow for such influence. Although similar in spirit to the joint domain-range model, we show that our model overcomes certain deficiencies in that model. We use a spatially dependent prior for the background and foreground. The background and foreground labels from the previous frame, after spatial smoothing to account for movement of objects, are used to build the prior for the current frame. These components are, by themselves, not novel aspects in background modeling. As we will show, many existing systems account for these aspects in different ways. We argue that separating these components as suggested in this paper yields a very simple and effective model. Our intuitive description also isolates the model components from the classification or inference step. Improvements to each model component can be carried out without any changes to the inference or other components. The various components can hence be modeled effectively and their impact on the overall system understood more easily.
更多查看译文
关键词
Background modeling,Motion segmentation,Surveillance
AI 理解论文
溯源树
样例
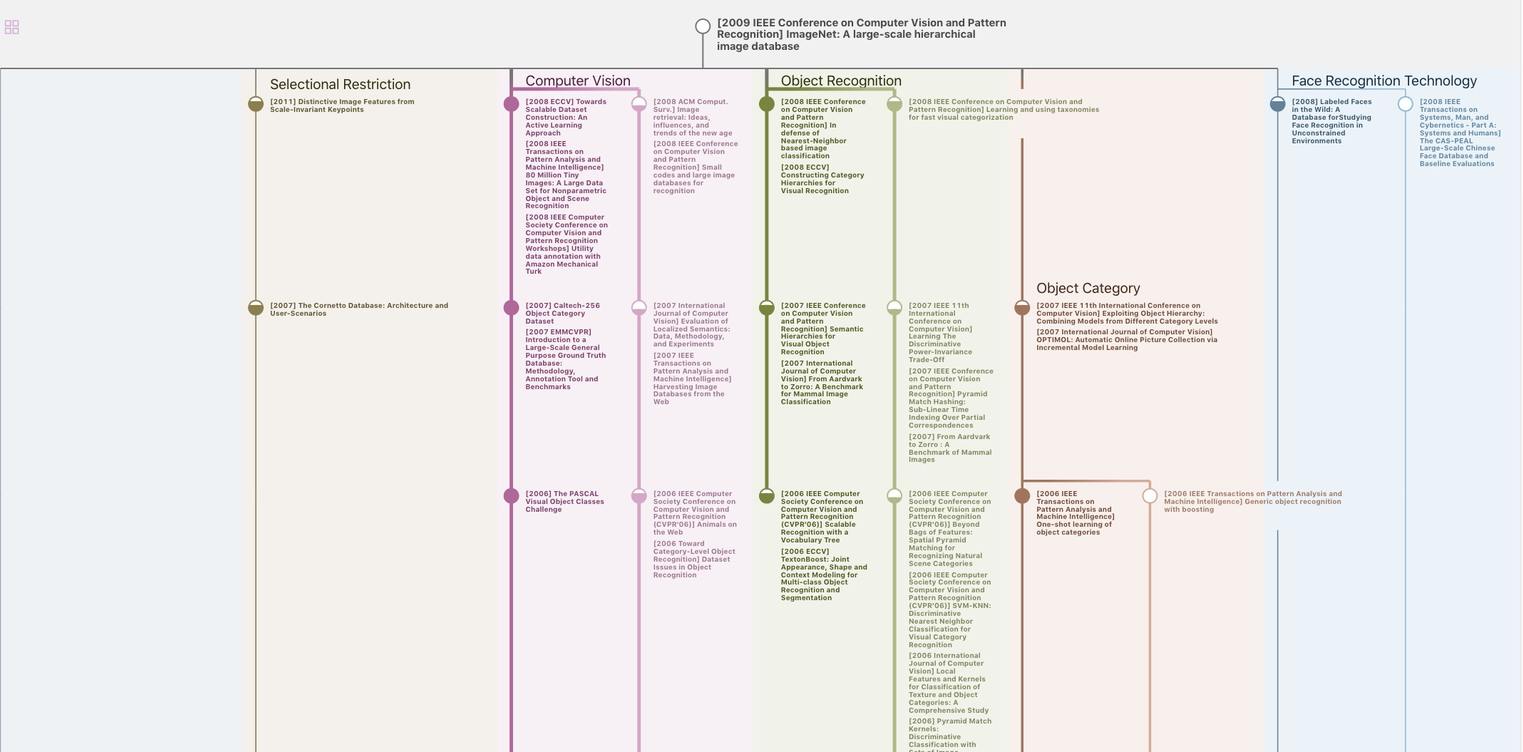
生成溯源树,研究论文发展脉络
Chat Paper
正在生成论文摘要