Generalized joint kernel regression and adaptive dictionary learning for single-image super-resolution.
Signal Processing(2014)
摘要
This paper proposes a new approach to single-image super-resolution (SR) based on generalized adaptive joint kernel regression (G-AJKR) and adaptive dictionary learning. The joint regression prior aims to regularize the ill-posed reconstruction problem by exploiting local structural regularity and nonlocal self-similarity of images. It is composed of multiple locally generalized kernel regressors defined over similar patches found in the nonlocal range which are combined, thus simultaneously exploiting both image statistics in a natural manner. Each regression group is then weighted by a regional redundancy measure we propose to control their relative effects of regularization adaptively. This joint regression prior is further generalized to the range of multi-scales and rotations. For robustness, adaptive dictionary learning and dictionary-based sparsity prior are introduced to interact with this prior. We apply the proposed method to both general natural images and human face images (face hallucination), and for the latter we incorporate a new global face prior into SR reconstruction while preserving face discriminativity. In both cases, our method outperforms other related state-of-the-art methods qualitatively and quantitatively. Besides, our face hallucination method also outperforms the others when applied to face recognition applications.
更多查看译文
关键词
Single-image super-resolution,Face hallucination,Face recognition,Joint kernel regression,Dictionary learning
AI 理解论文
溯源树
样例
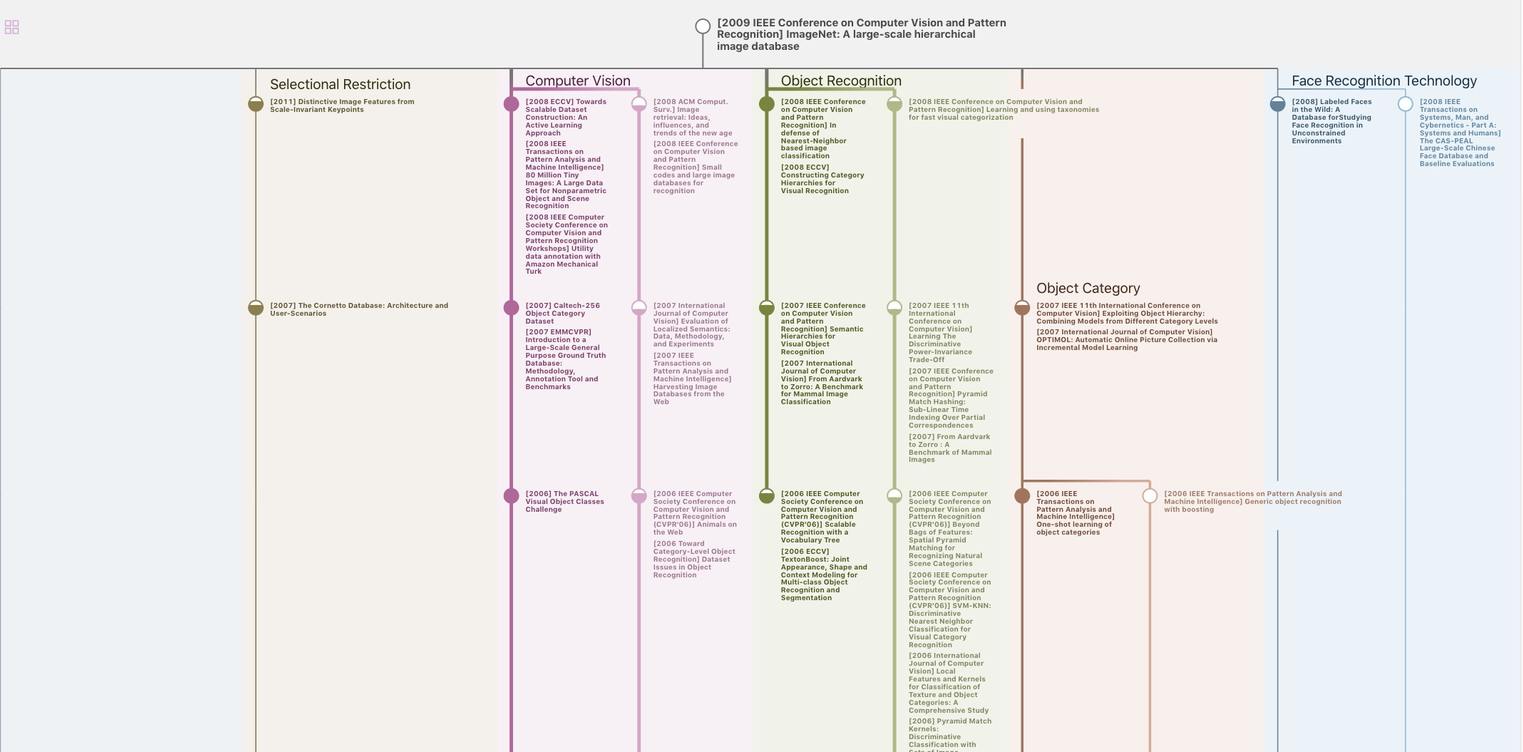
生成溯源树,研究论文发展脉络
Chat Paper
正在生成论文摘要