Multiobjective Pareto-Efficient Approaches for Recommender Systems.
ACM TIST(2015)
摘要
Recommender systems are quickly becoming ubiquitous in applications such as e-commerce, social media channels, and content providers, among others, acting as an enabling mechanism designed to overcome the information overload problem by improving browsing and consumption experience. A typical task in many recommender systems is to output a ranked list of items, so that items placed higher in the rank are more likely to be interesting to the users. Interestingness measures include how accurate, novel, and diverse are the suggested items, and the objective is usually to produce ranked lists optimizing one of these measures. Suggesting items that are simultaneously accurate, novel, and diverse is much more challenging, since this may lead to a conflicting-objective problem, in which the attempt to improve a measure further may result in worsening other measures. In this article, we propose new approaches for multiobjective recommender systems based on the concept of Pareto efficiency-a state achieved when the system is devised in the most efficient manner in the sense that there is no way to improve one of the objectives without making any other objective worse off. Given that existing multiobjective recommendation algorithms differ in their level of accuracy, diversity, and novelty, we exploit the Pareto-efficiency concept in two distinct manners: (i) the aggregation of ranked lists produced by existing algorithms into a single one, which we call Pareto-efficient ranking, and (ii) the weighted combination of existing algorithms resulting in a hybrid one, which we call Pareto-efficient hybridization. Our evaluation involves two real application scenarios: music recommendation with implicit feedback (i.e., Last. fm) and movie recommendation with explicit feedback (i.e., MovieLens). We show that the proposed Pareto-efficient approaches are effective in suggesting items that are likely to be simultaneously accurate, diverse, and novel. We discuss scenarios where the system achieves high levels of diversity and novelty without compromising its accuracy. Further, comparison against multiobjective baselines reveals improvements in terms of accuracy (from 10.4% to 10.9%), novelty (from 5.7% to 7.5%), and diversity (from 1.6% to 4.2%).
更多查看译文
关键词
Algorithms,Multiobjective recommender systems,Pareto efficiency
AI 理解论文
溯源树
样例
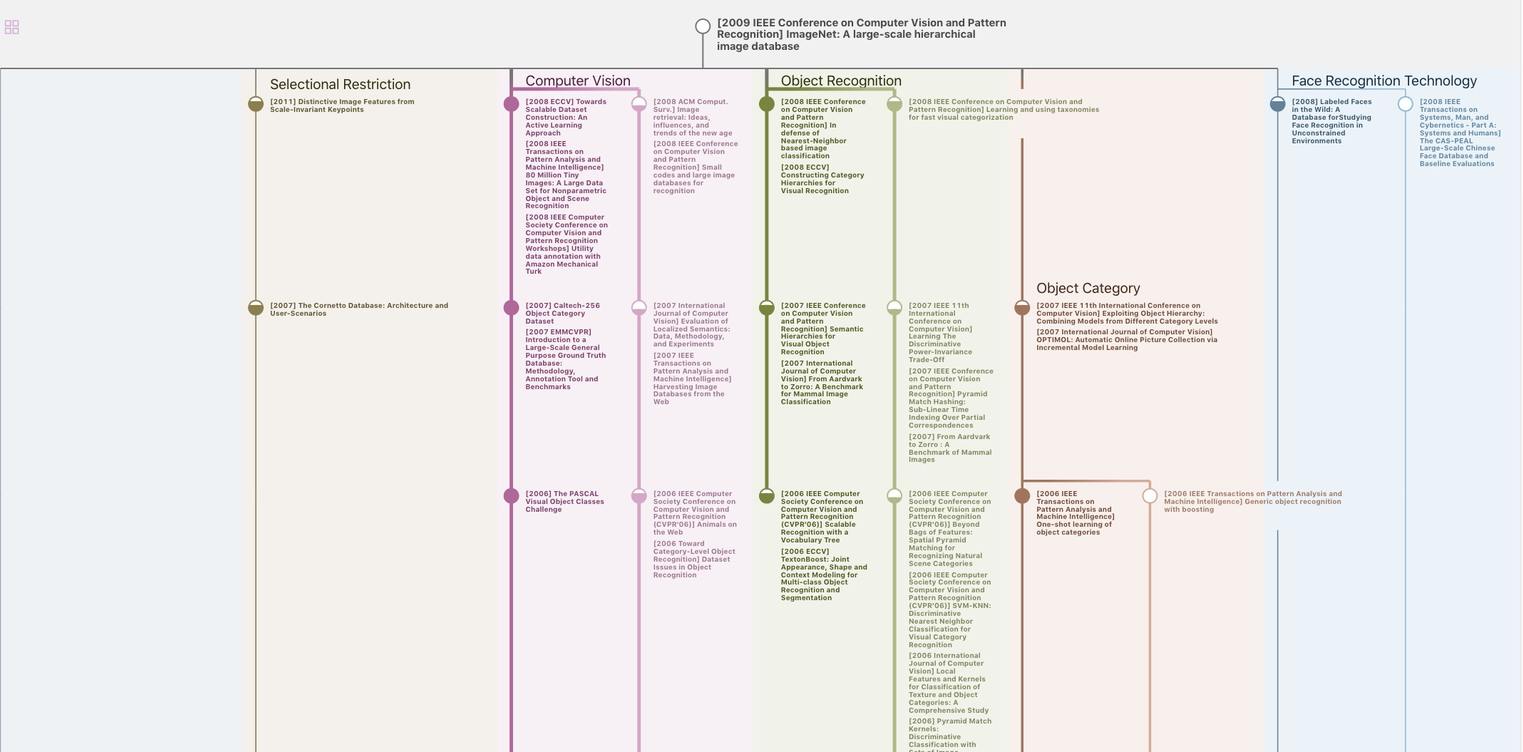
生成溯源树,研究论文发展脉络
Chat Paper
正在生成论文摘要