Minimax Analysis of Active Learning.
JOURNAL OF MACHINE LEARNING RESEARCH(2015)
摘要
This work establishes distribution-free upper and lower bounds on the minimax label complexity of active learning with general hypothesis classes, under various noise models. The results reveal a number of surprising facts. In particular, under the noise model of Tsybakov (2004), the minimax label complexity of active learning with a VC class is always asymptotically smaller than that of passive learning, and is typically significantly smaller than the best previously-published upper bounds in the active learning literature. In high noise regimes, it turns out that all active learning problems of a given VC dimension have roughly the same minimax label complexity, which contrasts with well-known results for bounded noise. In low-noise regimes, we find that the label complexity is well-characterized by a simple combinatorial complexity measure we call the star number. Interestingly, we find that almost all of the complexity measures previously explored in the active learning literature have worst-case values exactly equal to the star number. We also propose new active learning strategies that nearly achieve these minimax label complexities.
更多查看译文
关键词
active learning,selective sampling,sequential design,adaptive sampling,statistical learning theory,margin condition,Tsybakov noise,sample complexity,minimax analysis
AI 理解论文
溯源树
样例
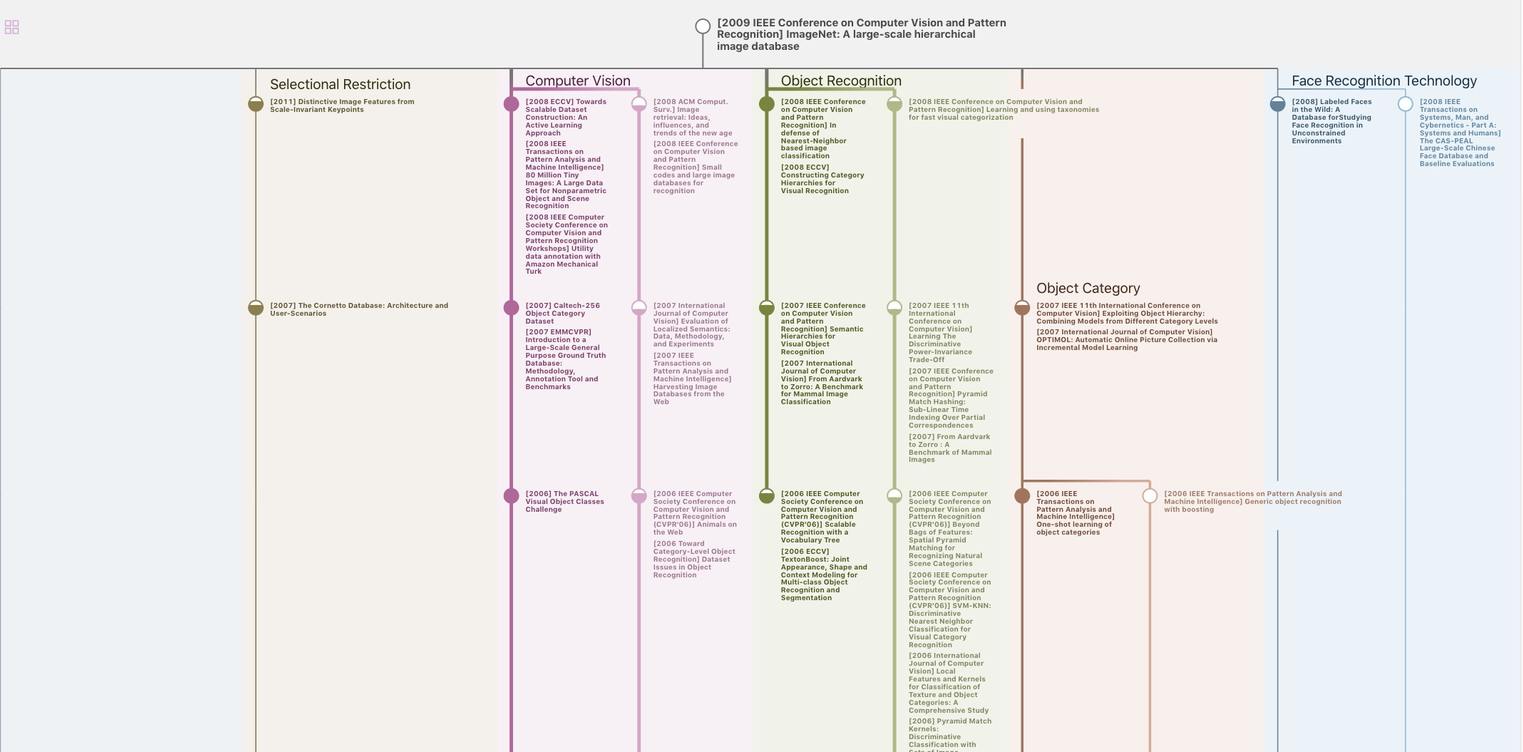
生成溯源树,研究论文发展脉络
Chat Paper
正在生成论文摘要