A Multiplicative Model For Learning Distributed Text-Based Attribute Representations
ADVANCES IN NEURAL INFORMATION PROCESSING SYSTEMS 27 (NIPS 2014)(2014)
摘要
In this paper we propose a general framework for learning distributed representations of attributes: characteristics of text whose representations can be jointly learned with word embeddings. Attributes can correspond to a wide variety of concepts, such as document indicators (to learn sentence vectors), language indicators (to learn distributed language representations), meta-data and side information (such as the age, gender and industry of a blogger) or representations of authors. We describe a third-order model where word context and attribute vectors interact multiplicatively to predict the next word in a sequence. This leads to the notion of conditional word similarity: how meanings of words change when conditioned on different attributes. We perform several experimental tasks including sentiment classification, cross-lingual document classification, and blog authorship attribution. We also qualitatively evaluate conditional word neighbours and attribute-conditioned text generation.
更多查看译文
AI 理解论文
溯源树
样例
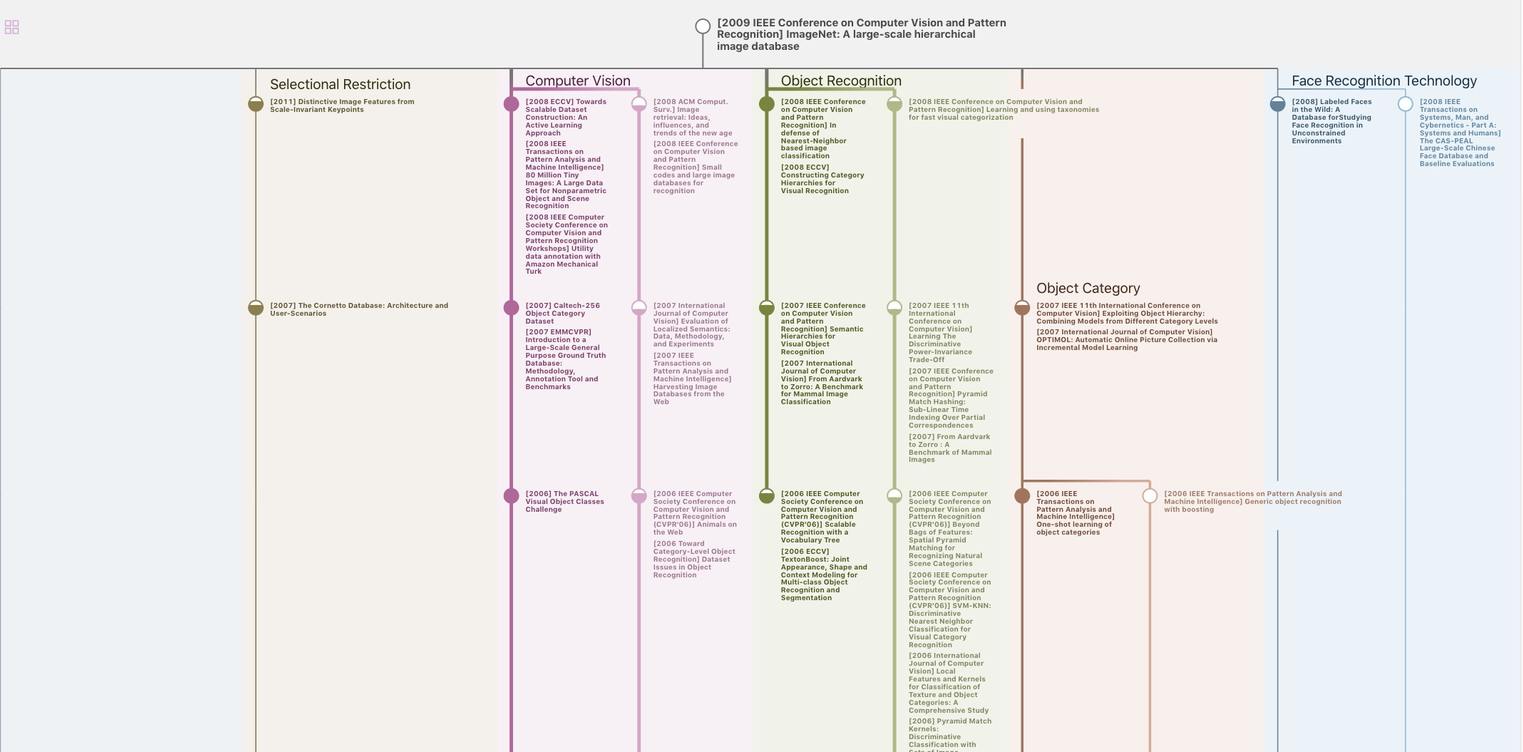
生成溯源树,研究论文发展脉络
Chat Paper
正在生成论文摘要