Privacy and Truthful Equilibrium Selection for Aggregative Games.
WINE 2015 Proceedings of the 11th International Conference on Web and Internet Economics - Volume 9470(2015)
摘要
We study a very general class of games -- multi-dimensional aggregative games -- which in particular generalize both anonymous games and weighted congestion games. For any such game that is also large, we solve the equilibrium selection problem in a strong sense. In particular, we give an efficient weak mediator: a mechanism which has only the power to listen to reported types and provide non-binding suggested actions, such that a it is an asymptotic Nash equilibrium for every player to truthfully report their type to the mediator, and then follow its suggested action; and b that when players do so, they end up coordinating on a particular asymptotic pure strategy Nash equilibrium of the induced complete information game. In fact, truthful reporting is an ex-post Nash equilibrium of the mediated game, so our solution applies even in settings of incomplete information, and even when player types are arbitrary or worst-case i.e. not drawn from a common prior. We achieve this by giving an efficient differentially private algorithm for computing a Nash equilibrium in such games. The rates of convergence to equilibrium in all of our results are inverse polynomial in the number of players n. We also apply our main results to a multi-dimensional market game. Our results can be viewed as giving, for a rich class of games, a more robust version of the Revelation Principle, in that we work with weaker informational assumptions no common prior, yet provide a stronger solution concept ex-post Nash versus Bayes Nash equilibrium. In comparison to previous work, our main conceptual contribution is showing that weak mediators are a game theoretic object that exist in a wide variety of games --- previously, they were only known to exist in traffic routing games. We also give the first weak mediator that can implement an equilibrium optimizing a linear objective function, rather than implementing a possibly worst-case Nash equilibrium.
更多查看译文
关键词
Differential privacy, Equilibrium computation, Mechanism design
AI 理解论文
溯源树
样例
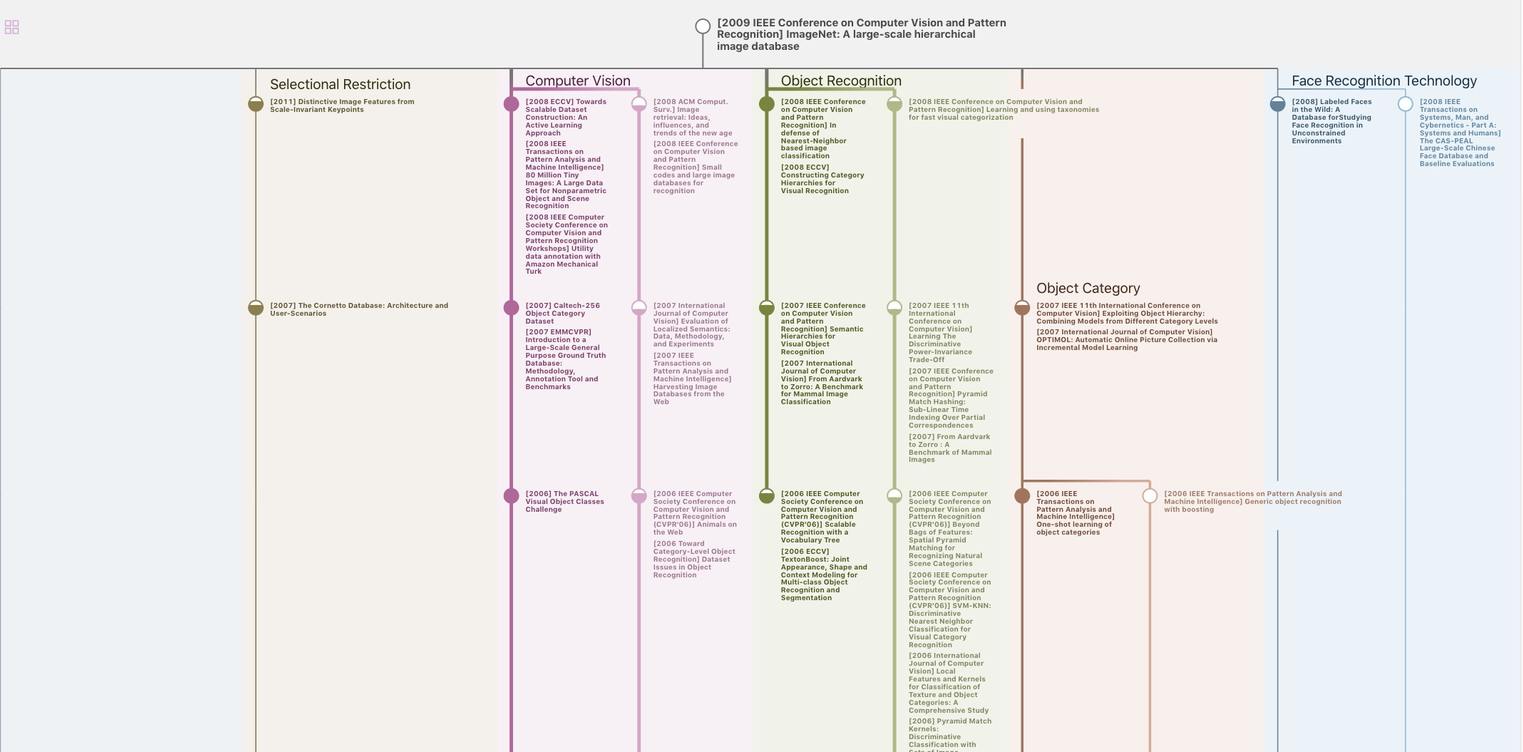
生成溯源树,研究论文发展脉络
Chat Paper
正在生成论文摘要