Random bits regression: a strong general predictor for big data
Big Data Analytics(2016)
摘要
Background Data-based modeling is becoming practical in predicting outcomes. In the era of big data, two practically conflicting challenges are eminent: (1) the prior knowledge on the subject is largely insufficient; (2) computation and storage cost of big data is unaffordable. Results To improve accuracy and speed of regressions and classifications, we present a data-based prediction method, Random Bits Regression (RBR). This method first generates a large number of random binary intermediate/derived features based on the original input matrix, and then performs regularized linear/logistic regression on those intermediate/derived features to predict the outcome. Benchmark analyses on a simulated dataset, UCI machine learning repository datasets and a GWAS dataset showed that RBR outperforms other popular methods in accuracy and robustness. Conclusions RBR (available on https://sourceforge.net/projects/rbr/ ) is very fast and requires reasonable memories, therefore, provides a strong, robust and fast predictor in the big data era.
更多查看译文
关键词
RBR,Regression,Classification,Machine learning,Big data prediction
AI 理解论文
溯源树
样例
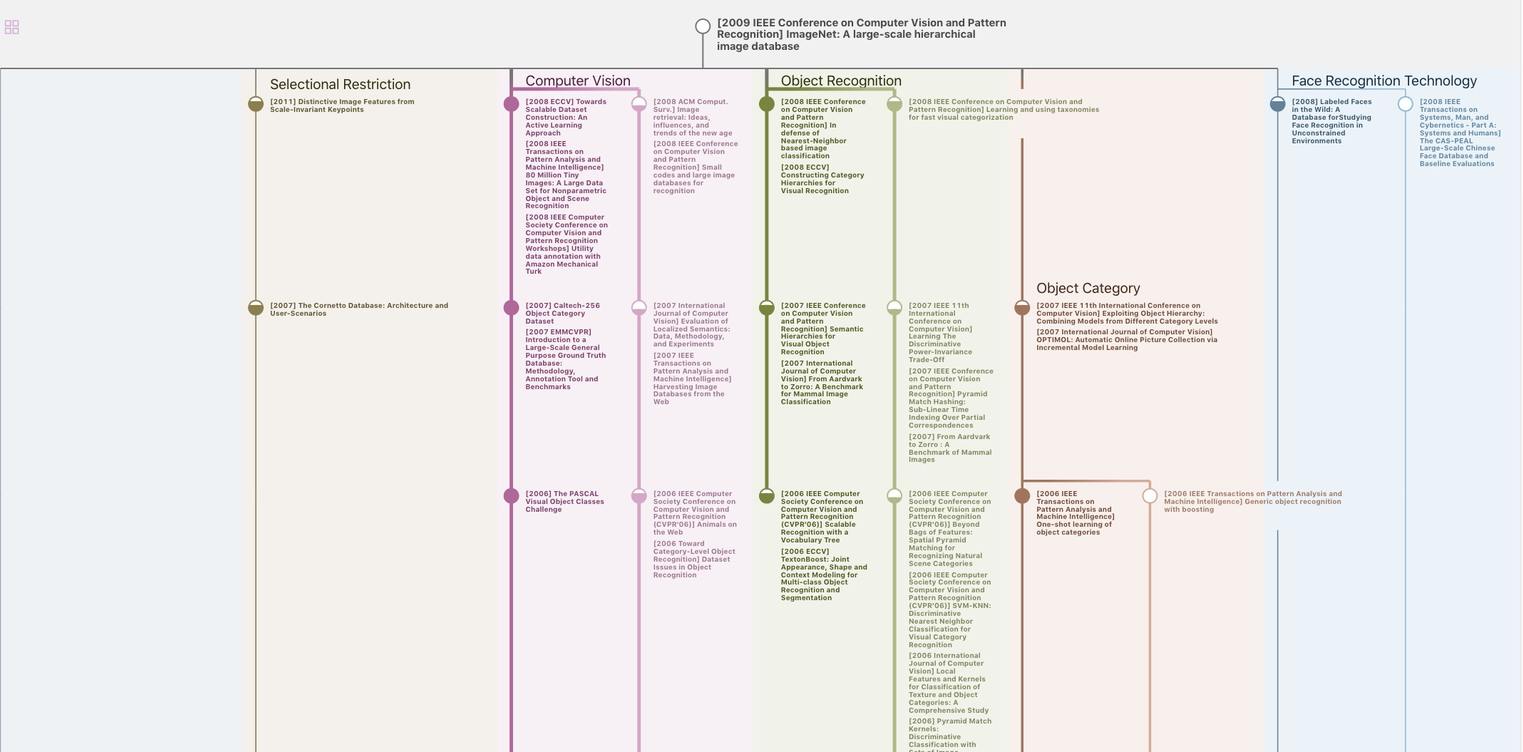
生成溯源树,研究论文发展脉络
Chat Paper
正在生成论文摘要