Variance Analysis of Linear SIMO Models with Spatially Correlated Noise.
Automatica(2017)
摘要
In this paper we address the identification of linear time-invariant single-input multi-output (SIMO) systems. In particular, we assess the performance of the prediction error method by quantifying the variance of the parameter estimates. Using an orthonormal representation for the modules composing the SIMO structure, we show that the parameter estimate of a module depends on the model structure of the other modules, and on the correlation structure of the output disturbances. We provide novel results which quantify the variance-error of the parameter estimates for finite model orders, where the effects of noise correlation structure, model structure and input spectrum are visible. In particular, we show that a sensor does not increase the accuracy of a module if common dynamics have to be estimated. When a module is identified using less parameters than the other modules, we derive the noise correlation structure that gives the minimum total variance. The implications of our results are illustrated through numerical examples and simulations.
更多查看译文
关键词
System identification,Asymptotic variance,Linear SIMO models,Least-squares
AI 理解论文
溯源树
样例
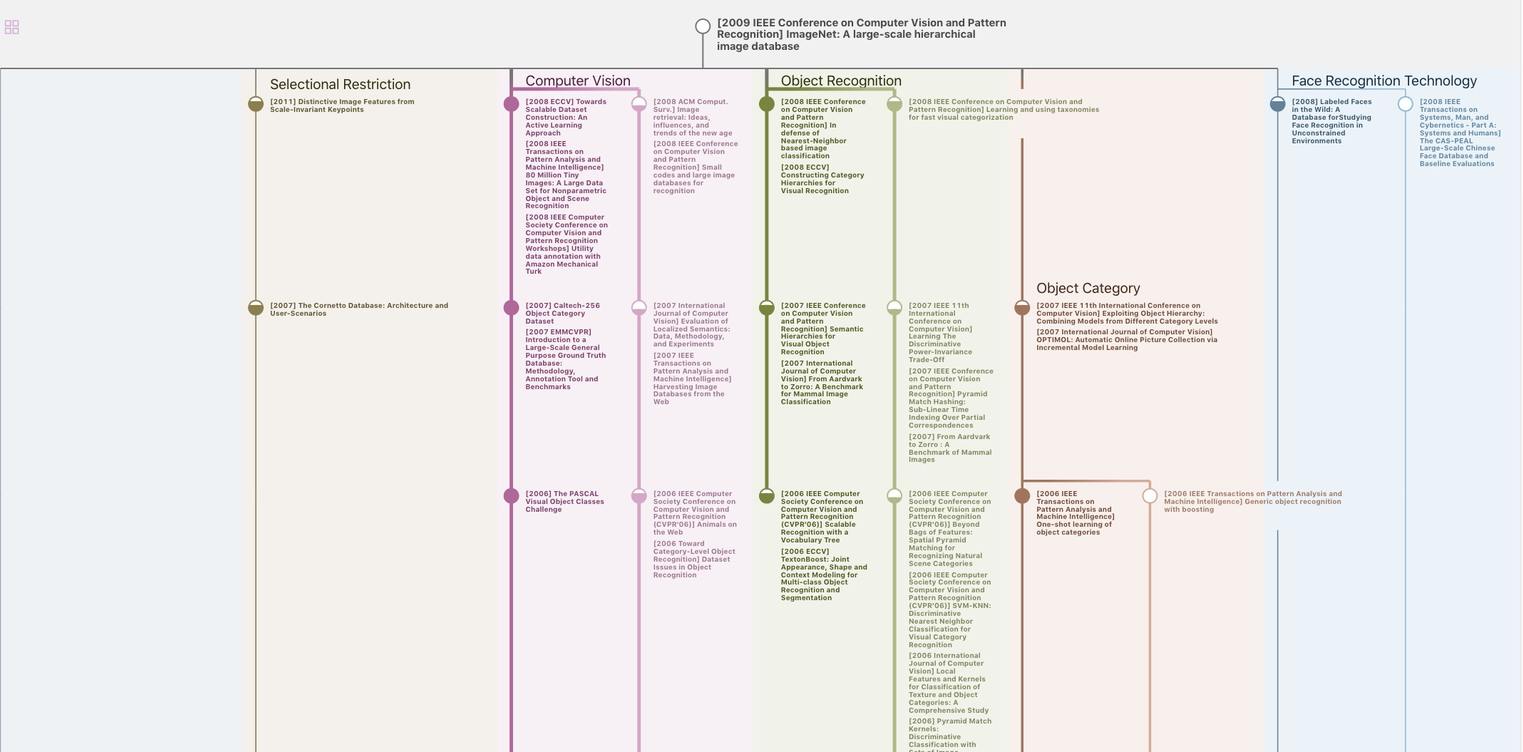
生成溯源树,研究论文发展脉络
Chat Paper
正在生成论文摘要