Improved open-vocabulary spoken content retrieval with word and subword lattices using acoustic feature similarity
Computer Speech & Language(2014)
摘要
Spoken content retrieval will be very important for retrieving and browsing multimedia content over the Internet, and spoken term detection (STD) is one of the key technologies for spoken content retrieval. In this paper, we show acoustic feature similarity between spoken segments used with pseudo-relevance feedback and graph-based re-ranking can improve the performance of STD. This is based on the concept that spoken segments similar in acoustic feature vector sequences to those with higher/lower relevance scores should have higher/lower scores, while graph-based re-ranking further uses a graph to consider the similarity structure among all the segments retrieved in the first pass. These approaches are formulated on both word and subword lattices, and a complete framework of using them in open vocabulary retrieval of spoken content is presented. Significant improvements for these approaches with both in-vocabulary and out-of-vocabulary queries were observed in preliminary experiments.
更多查看译文
关键词
Spoken content retrieval,Spoken term detection,Pseudo-relevance feedback,Random walk
AI 理解论文
溯源树
样例
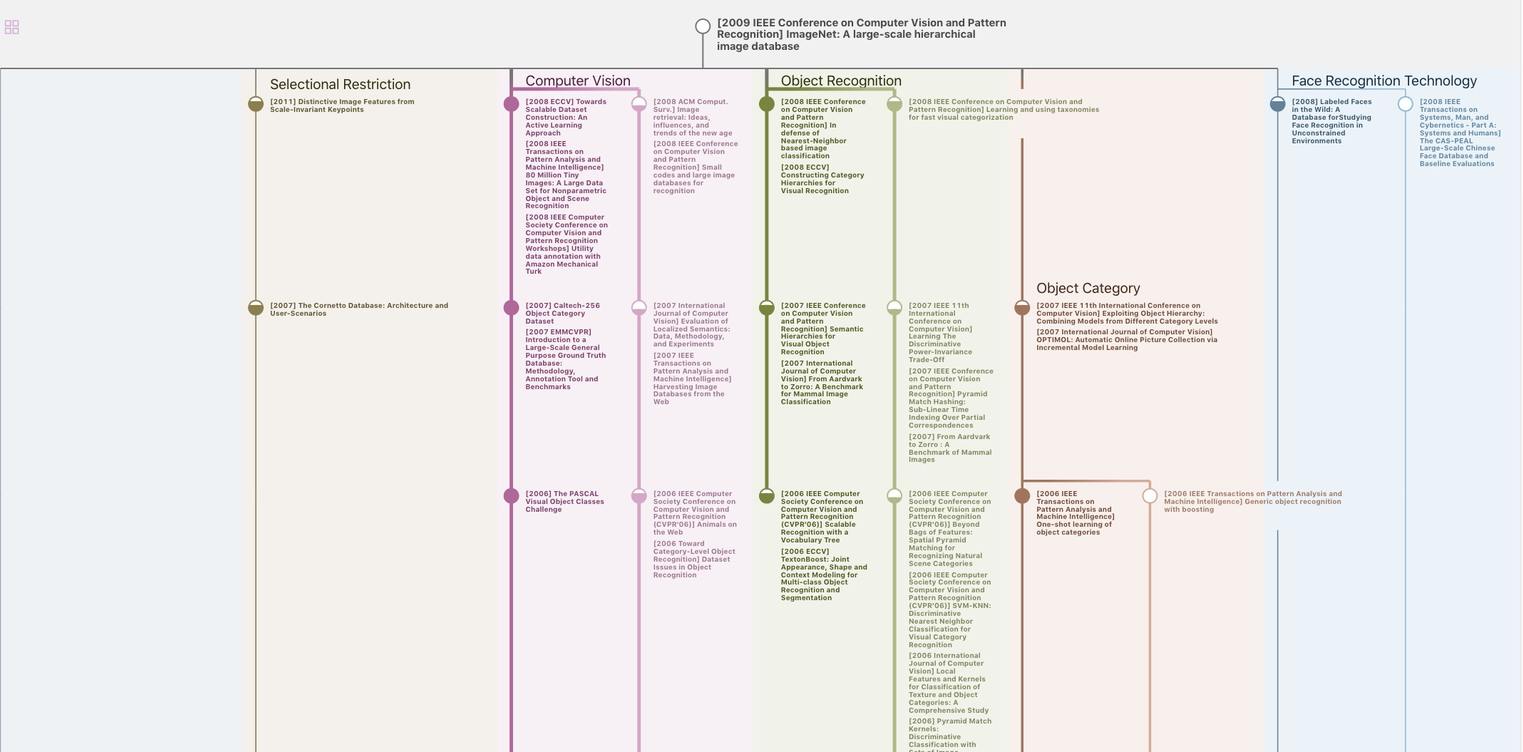
生成溯源树,研究论文发展脉络
Chat Paper
正在生成论文摘要