Blind Source Separation by Entropy Rate Minimization
IEEE Transactions on Signal Processing(2014)
摘要
By assuming latent sources are statistically independent, independent component analysis separates underlying sources from a given linear mixture. Since in many applications, latent sources are both non-Gaussian and have sample dependence, it is desirable to exploit both properties jointly. In this paper, we use mutual information rate to construct a general framework for analysis and derivation of algorithms that take both properties into account. We discuss two types of source models for entropy rate estimation-a Markovian and an invertible filter model-and give the general independent component analysis cost function, update rule, and performance analysis based on these. We also introduce four algorithms based on these two models, and show that their performance can approach the Cramér-Rao lower bound. In addition, we demonstrate that the algorithms with flexible models exhibit very desirable performance for “natural” data.
更多查看译文
关键词
maximum entropy distribution,markov model,mutual information rate,entropy rate minimization,independent component analysis,linear mixture,cramér-rao lower bound,blind source separation,invertible filter model,independent component analysis cost function,entropy rate estimation
AI 理解论文
溯源树
样例
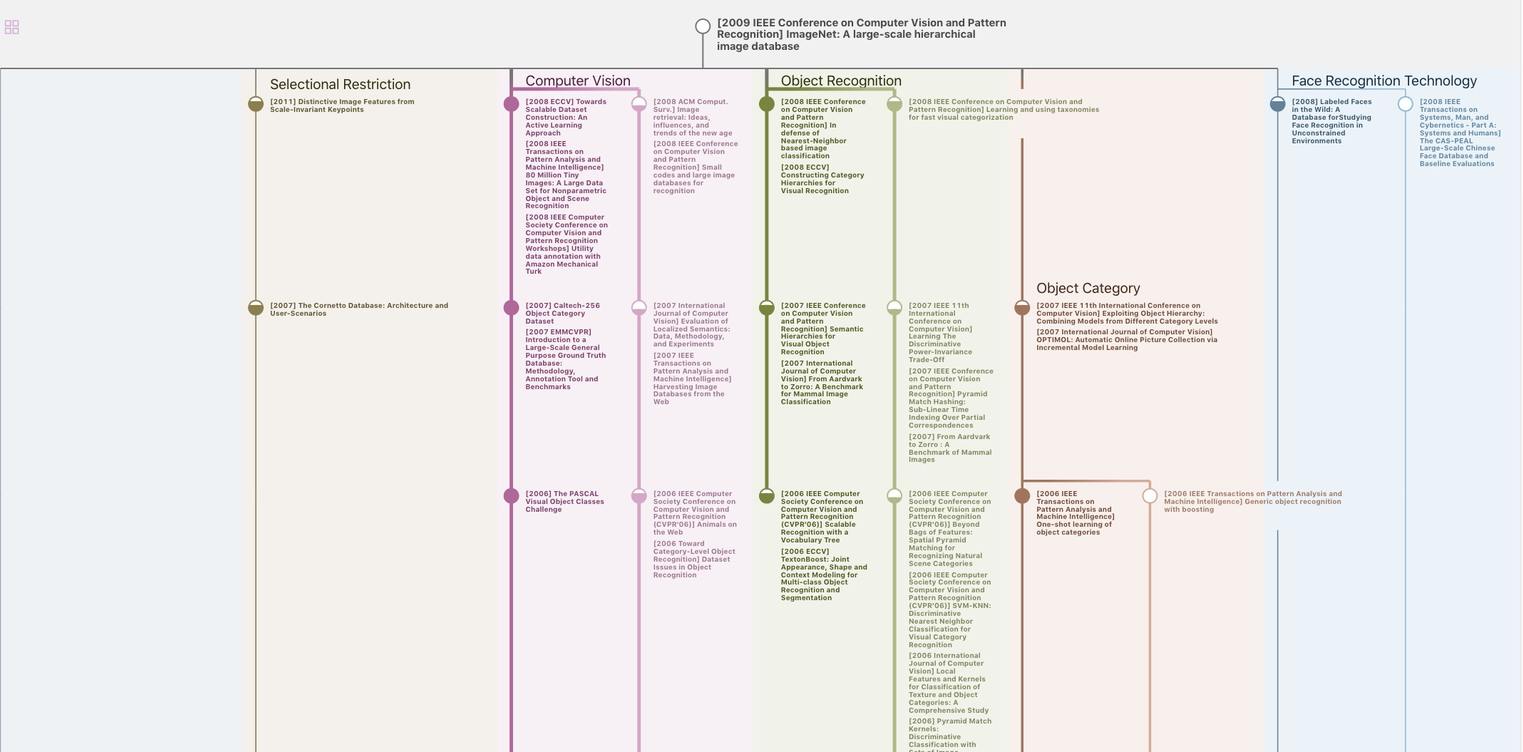
生成溯源树,研究论文发展脉络
Chat Paper
正在生成论文摘要